Mélange: Cost Efficient Large Language Model Serving by Exploiting GPU Heterogeneity
arxiv(2024)
摘要
Large language models (LLMs) are increasingly integrated into many online
services. However, a major challenge in deploying LLMs is their high cost, due
primarily to the use of expensive GPU instances. To address this problem, we
find that the significant heterogeneity of GPU types presents an opportunity to
increase GPU cost efficiency and reduce deployment costs. The broad and growing
market of GPUs creates a diverse option space with varying costs and hardware
specifications. Within this space, we show that there is not a linear
relationship between GPU cost and performance, and identify three key LLM
service characteristics that significantly affect which GPU type is the most
cost effective: model request size, request rate, and latency service-level
objective (SLO). We then present Mélange, a framework for navigating the
diversity of GPUs and LLM service specifications to derive the most
cost-efficient set of GPUs for a given LLM service. We frame the task of GPU
selection as a cost-aware bin-packing problem, where GPUs are bins with a
capacity and cost, and items are request slices defined by a request size and
rate. Upon solution, Mélange derives the minimal-cost GPU allocation that
adheres to a configurable latency SLO. Our evaluations across both real-world
and synthetic datasets demonstrate that Mélange can reduce deployment costs
by up to 77
importance of making heterogeneity-aware GPU provisioning decisions for LLM
serving. Our source code is publicly available at
https://github.com/tyler-griggs/melange-release.
更多查看译文
AI 理解论文
溯源树
样例
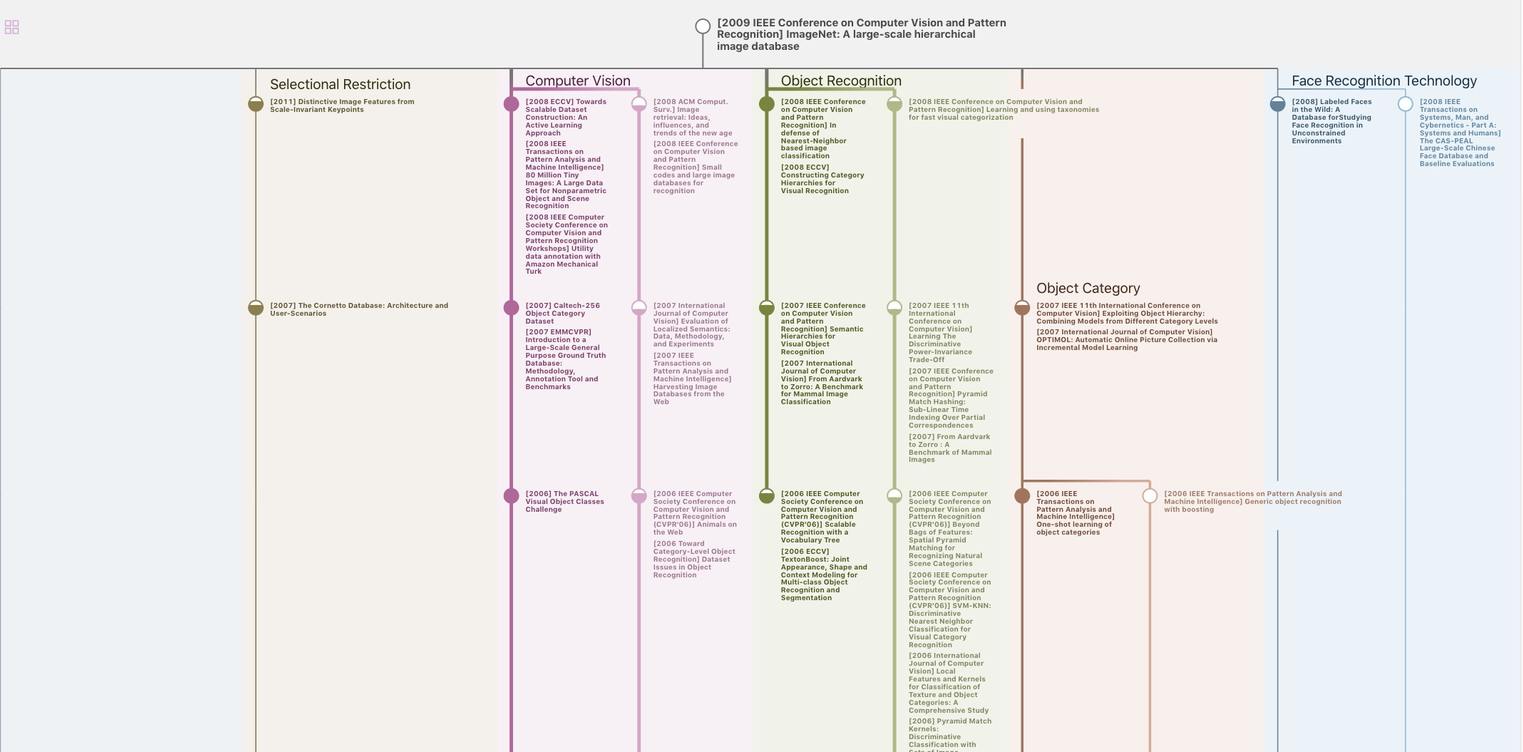
生成溯源树,研究论文发展脉络
Chat Paper
正在生成论文摘要