Learning-Based Attribute-Augmented Proximity Matrix Factorization for Attributed Network Embedding
IEEE Transactions on Knowledge and Data Engineering(2024)
摘要
Given a graph
$\mathcal {G}$
with a set of attributes, the
attributed network embedding (ANE)
aims to learn low-dimensional representations of nodes that preserve both graph topology and node attribute proximity. ANE is shown to be more effective than plain network embedding methods (using only graph topology) on many graph mining tasks. However, existing ANE solutions still provide inferior performance on tasks like node classification and link prediction, as will be shown in our experiments. The key issue is that when combining graph topology and attribute information, most existing solutions take attributes with equal importance, while in real scenarios, different attribute exerts distinct influence over the network due to the heterogeneous nature among attributes. Motivated by this, we present
LATAM
, a learning-based framework for ANE via trainable proximity matrix factorization. To capture the node-attribute relationships, we first construct the attribute-augmented graph by adding attribute nodes (resp. edges) to the original graph. Then, we define the attribute-augmented random walk and proximity on the attribute-augmented graph, where the weights of different attributes can be learned automatically by our designed loss functions so that more indicative attributes tend to have higher weights, imposing a higher impact on the node connectivity. To achieve this, we incorporate a differentiable SVD to back-propagate gradients of attribute weights in an end-to-end process. To scale our LATAM to large graphs, we further propose sampling techniques to learn attribute weights and an efficient attribute-augmented push algorithm to compute the proximity matrix. Extensive experiments on 8 public attributed networks against 11 existing methods show the effectiveness of our LATAM.
更多查看译文
关键词
Attributed network embedding,matrix factorization,trainable proximity
AI 理解论文
溯源树
样例
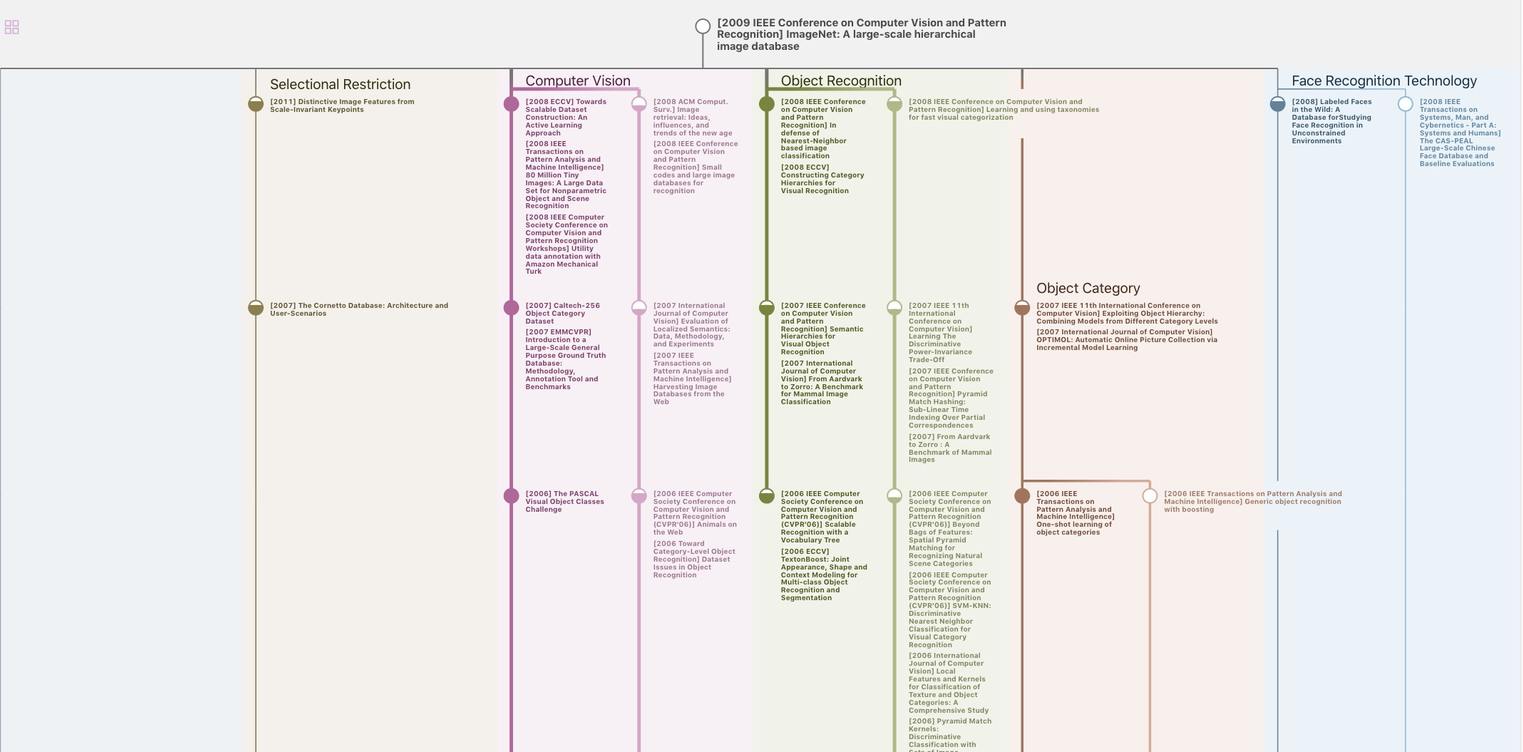
生成溯源树,研究论文发展脉络
Chat Paper
正在生成论文摘要