De2Net: Under-display camera image restoration with feature deconvolution and kernel decomposition
Computer Vision and Image Understanding(2024)
摘要
While the under-display camera (UDC) system provides an effective solution for notch-free full-screen displays, it inevitably causes severe image quality degradation due to the diffraction phenomenon. Recent methods have achieved decent performance with deep neural networks, yet the characteristic of the point spread function (PSF) is less studied. In this paper, considering the large support and spatial inconsistency of PSF, we propose De2Net for UDC image restoration with feature deconvolution and kernel decomposition. In terms of feature deconvolution, we introduce Wiener deconvolution as a preliminary process, which alleviates feature entanglement caused by the large PSF support. Besides, the deconvolution kernel can be learned from training images, eliminating the tedious PSF-obtaining process. As for kernel decomposition, we observe regular patterns for PSFs at different positions. Thus, with a kernel prediction network (KPN) deployed for handling the spatial inconsistency problem, we improve it from two aspects, i.e., (i) decomposing the predicted kernels into a set of bases and weights, (ii) decomposing kernels into groups with different dilation rates. These modifications largely improve the receptive field under certain memory limits. Extensive experiments on three commonly used UDC datasets show that De2Net outperforms existing methods both quantitatively and qualitatively. Source code and pre-trained models are available at https://github.com/HyZhu39/De2Net.
更多查看译文
关键词
Under-display camera,Image restoration,Point spread function,Kernel prediction network
AI 理解论文
溯源树
样例
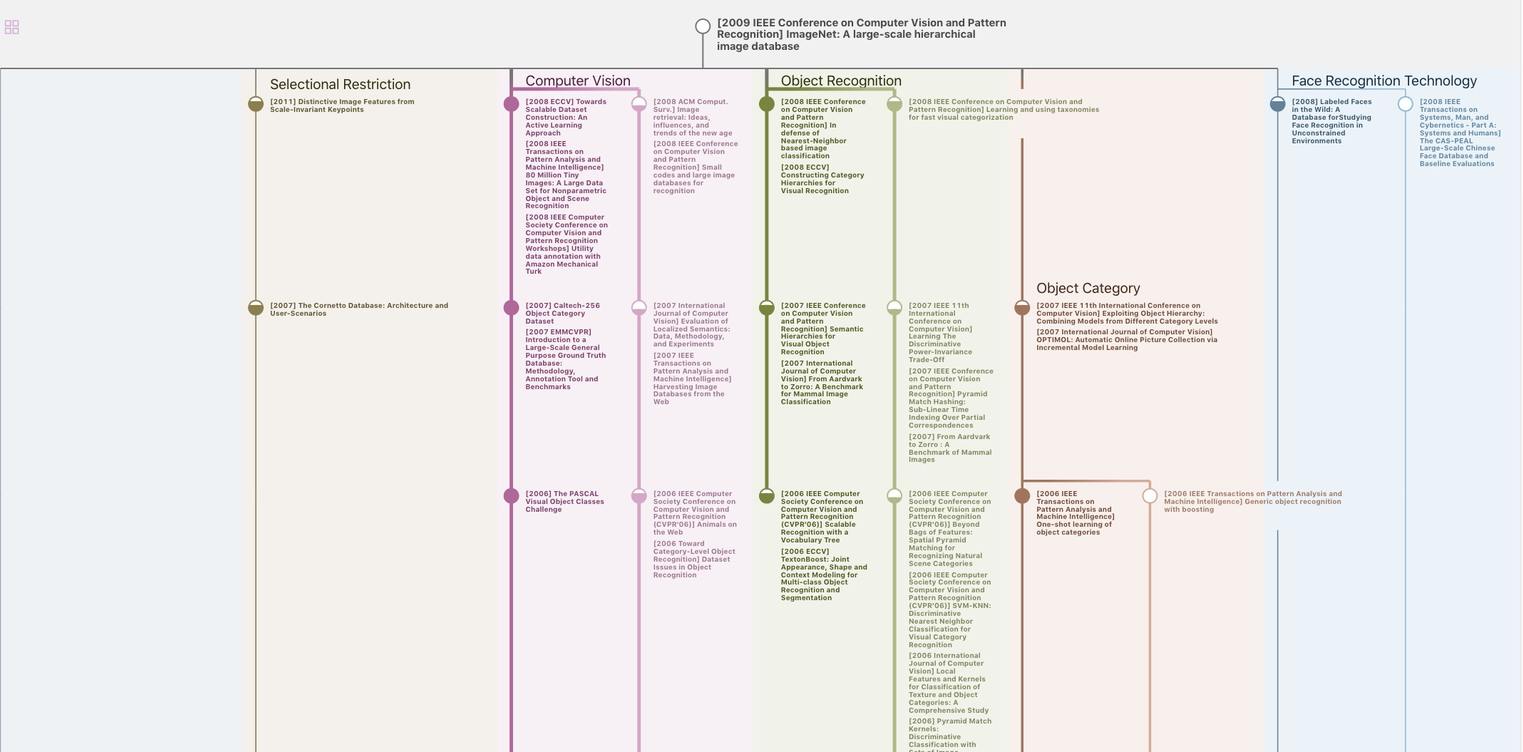
生成溯源树,研究论文发展脉络
Chat Paper
正在生成论文摘要