STORM: A Spatio-Temporal Context-Aware Model for Predicting Event-Triggered Abnormal Crowd Traffic
IEEE Transactions on Intelligent Transportation Systems(2024)
摘要
Urban events, such as hurricanes, floods, and epidemic outbreaks, usually have a significant impact on crowd behaviors that may dramatically change people’s mobility patterns, interaction manners, and etc. Accurately foreseeing the abnormal crowd behaviors triggered by urban events can help authorities to dynamically schedule urban resources and services, such as providing shuttle buses, temporal shelters, and psychological first aid. However, traditional crowd behavior prediction models tend to learn regular trends and patterns of crowd traffic, and usually fail to predict the abnormal traffic fluctuations triggered by urban events. In this work, we propose a context-aware framework to accurately predict event-triggered abnormal crowd traffic by explicitly modeling event contexts and their impact. We start by training a state-of-the-art spatiotemporal multi-graph model (MG) leveraging a multi-graph convolutional network (MGCN) to model city-wide venues and their connections, as well as gated recurrent unit (GRU) to capture the crowd traffic patterns within venues. In order to model the time-occasional impact of sudden influential event context (e.g., weather conditions), we propose a multi-task fusion diagram to co-train the MG model by learning a temporal impact embedding with an attention mechanism and recurrent neural network to obtain the MG-MT model. In order to model the hyper-space impact of irregularly distributed event context (e.g., epicenters and trajectories), we propose a multi-view fusion diagram to fine-tune the above-mentioned model by learning a spatial impact graph with a stimulus-response mechanism to obtain the spatiotemporal crowd traffic model (STORM). Experiments using real-world urban data collected from Xiamen, China show that our approach improves over the state-of-the-art baseline by more than 6% in predicting crowd traffic and by more than 40% in the abnormal crowd traffic part.
更多查看译文
关键词
Crowd-behavior prediction,spatiotemporal data,urban computing
AI 理解论文
溯源树
样例
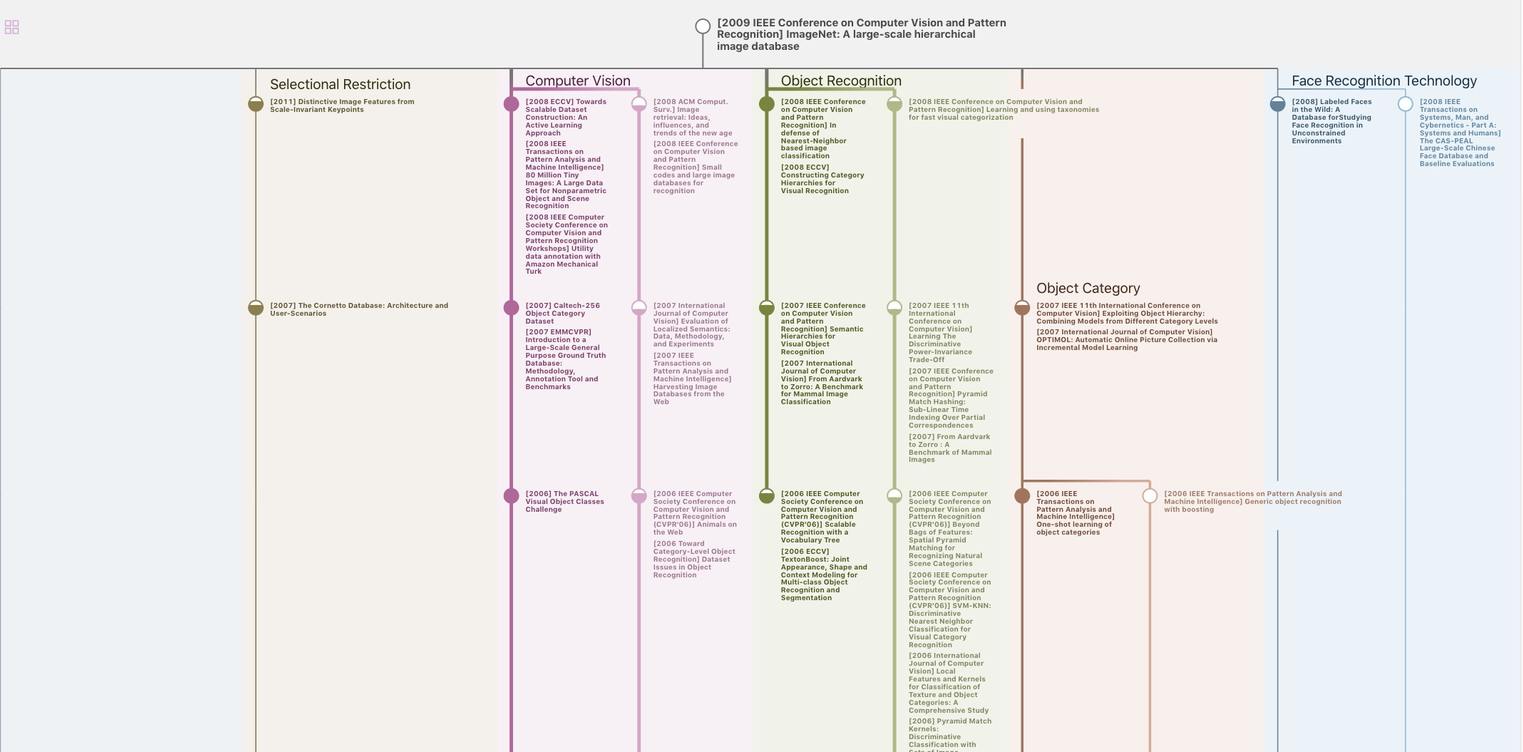
生成溯源树,研究论文发展脉络
Chat Paper
正在生成论文摘要