Achieving EEG-based depression recognition using Decentralized-Centralized structure
Biomedical Signal Processing and Control(2024)
摘要
Currently, electroencephalography (EEG)-based depression recognition using deep learning methods has become an important approach for early diagnosis and treatment. Due to the functional differences and activation differences among various brain regions, the channels in EEG data may potentially exhibit a certain degree of local correlation. Furthermore, several studies have indicated that there are significant differences in the synchronization for multiple brain regions between patients with depression and healthy controls. However, few researchers have designed depression recognition model architectures according to the spatial distribution characteristics of EEG data. In multiple existing EEG-based classification models, the channels of EEG data are directly merged. This approach hinders the model from effectively capturing the spatial information of EEG data and leads to a partial loss of synchronized information of several brain regions. In light of this, we propose a novel attention network named Decentralized-Centralized Structure Transformer (DCST), which can achieve effective depression recognition utilizing the spatial distribution characteristics of EEG data. The leave-one-subject-out cross-validation is employed to evaluate the classification performance for all models. The experimental results show that our proposed model achieves the highest classification accuracy, 89.8%.
更多查看译文
关键词
Depression recognition,Electroencephalography (EEG),Deep learning,Transformer,Attention network
AI 理解论文
溯源树
样例
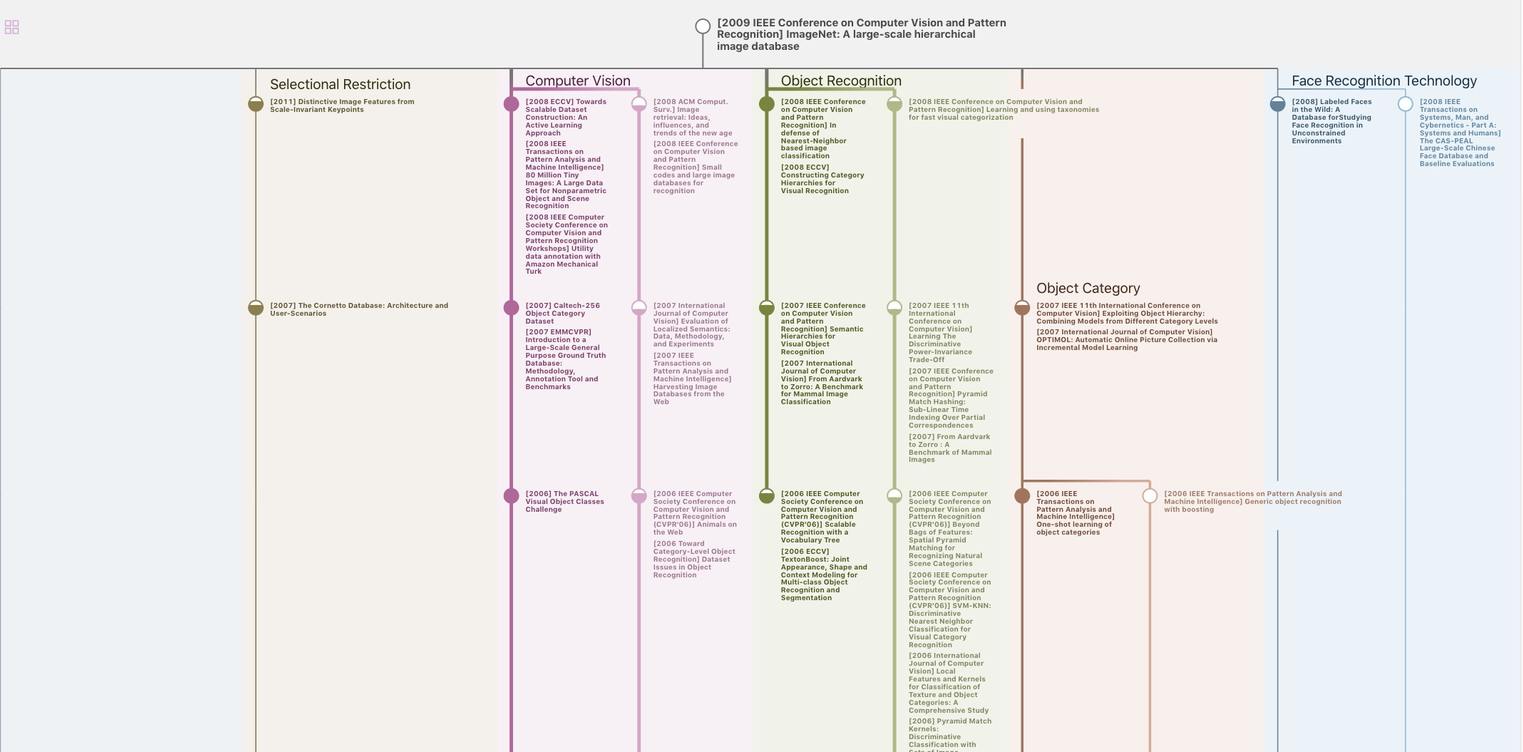
生成溯源树,研究论文发展脉络
Chat Paper
正在生成论文摘要