Focus on Informative Graphs! Semi-supervised Active Learning for Graph-Level Classification
Pattern recognition(2024)
摘要
Graph-level classification is a critical problem in social analysis and bioinformatics. Since annotated labels are typically costly, we intend to study this challenging task in semi-supervised scenarios with limited budgets. Inspired by the fact that active learning is capable of interactively querying an oracle to annotate a small number of informative examples in the unlabeled dataset, we develop a novel Semi-supervised active learning framework termed GraphSpa for graph-level classification. To make the most of labeling budgets, we propose an effective unlabeled data selection strategy that takes both local similarity and global semantic structure into account. Specifically, we first construct an adaptive queue with labeled samples and select informative samples that have a low degree of similarity to the queue using the Min-Max principle from the local view. Further, we introduce class prototypes and select samples with a large predictive loss discrepancy from the global view. To harness the full potential of unlabeled data, we develop a semi-supervised active learning framework on the basis of our fusion selection strategy coupled with graph contrastive learning during active learning. The effectiveness of our GraphSpa is validated against state-of-the-art methods through experimental results on diverse real-world benchmark datasets.
更多查看译文
关键词
Graph classification,Graph neural networks,Semi-supervised learning,Active learning
AI 理解论文
溯源树
样例
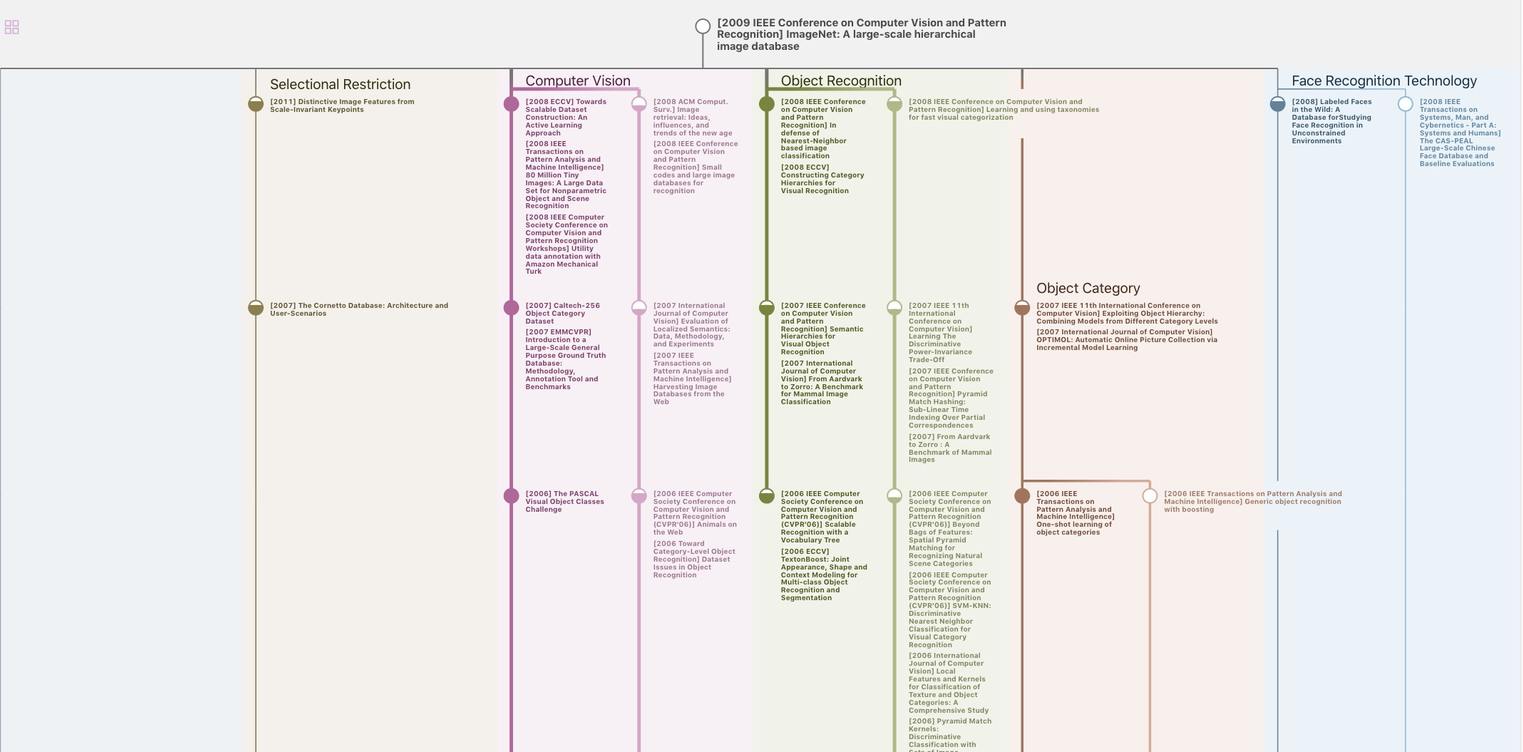
生成溯源树,研究论文发展脉络
Chat Paper
正在生成论文摘要