CatTSunami: Accelerating Transition State Energy Calculations with Pre-trained Graph Neural Networks
arxiv(2024)
摘要
Direct access to transition state energies at low computational cost unlocks
the possibility of accelerating catalyst discovery. We show that the top
performing graph neural network potential trained on the OC20 dataset, a
related but different task, is able to find transition states energetically
similar (within 0.1 eV) to density functional theory (DFT) 91
a 28x speedup. This speaks to the generalizability of the models, having never
been explicitly trained on reactions, the machine learned potential
approximates the potential energy surface well enough to be performant for this
auxiliary task. We introduce the Open Catalyst 2020 Nudged Elastic Band
(OC20NEB) dataset, which is made of 932 DFT nudged elastic band calculations,
to benchmark machine learned model performance on transition state energies. To
demonstrate the efficacy of this approach, we replicated a well-known, large
reaction network with 61 intermediates and 174 dissociation reactions at DFT
resolution (40 meV). In this case of dense NEB enumeration, we realize even
more computational cost savings and used just 12 GPU days of compute, where DFT
would have taken 52 GPU years, a 1500x speedup. Similar searches for complete
reaction networks could become routine using the approach presented here.
Finally, we replicated an ammonia synthesis activity volcano and systematically
found lower energy configurations of the transition states and intermediates on
six stepped unary surfaces. This scalable approach offers a more complete
treatment of configurational space to improve and accelerate catalyst
discovery.
更多查看译文
AI 理解论文
溯源树
样例
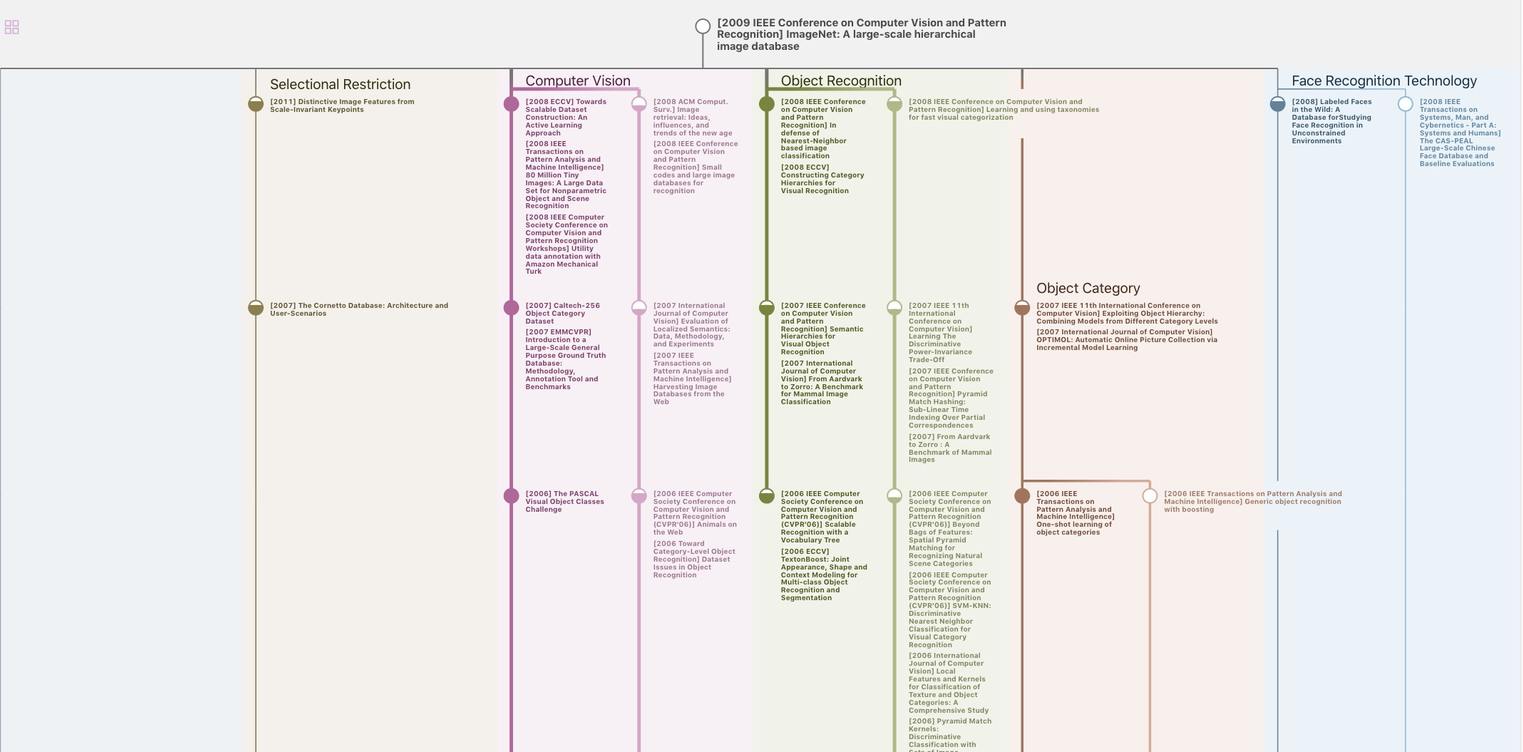
生成溯源树,研究论文发展脉络
Chat Paper
正在生成论文摘要