Global Meets Local: Dual Activation Hashing Network for Large-Scale Fine-Grained Image Retrieval
IEEE transactions on knowledge and data engineering(2024)
摘要
In the Internet era, the exponential growth of fine-grained image databases poses a considerable challenge for efficient information retrieval. Hashing-based approaches gained traction for their computational and storage efficiency, yet fine-grained hashing retrieval presents unique challenges due to small inter-class and large intra-class variations inherent to fine-grained entities. Thus, traditional hashing algorithms falter in discerning these subtle, yet critical, visual differences and fail to generate compact yet semantically rich hash codes. To address this, we introduce a Dual Activation Hashing Network ( DAHNet ) designed to convert high-dimensional image data into optimized binary codes via an innovative feature activation paradigm. The architecture consists of dual branches specifically tailored for global and local semantic activation, thereby establishing direct correspondences between hash codes and distinguishable object parts through a hierarchical activation pipeline. Specifically, our spatial-oriented semantic activation module modulates dominant visual regions while amplifying the activations of subtle yet semantically rich areas in a controlled manner. Building on these activated visual representations, the proposed inter-region semantic enrichment module further enriches them by unearthing semantically complementary cues. Concurrently, DAHNet integrates a channel-oriented semantic activation module that exploits channel-specific correlations to distill contextual cues from spatially-activated visual features, thereby reinforcing robust learning to hash. To maintain the similarity of the original entities, we amalgamate final hash codes from both activation branches, capturing both local textural details and global structural information. Comprehensive evaluations on five fine-grained image retrieval benchmarks demonstrate DAHNet 's superior performance over existing state-of-the-art hashing solutions, especially on 12-bit, improving performance by 4%-15% compared to the current best results on the five benchmarks. Moreover, generalization studies validate the efficacy of our dual-activation framework in the domain of content-based fine-grained image retrieval. The code is publicly available at: https://github.com/WhiteJiang/DAHNet .
更多查看译文
关键词
Feature activation,fine-grained hashing,fine-grained image retrieval,learning to hash
AI 理解论文
溯源树
样例
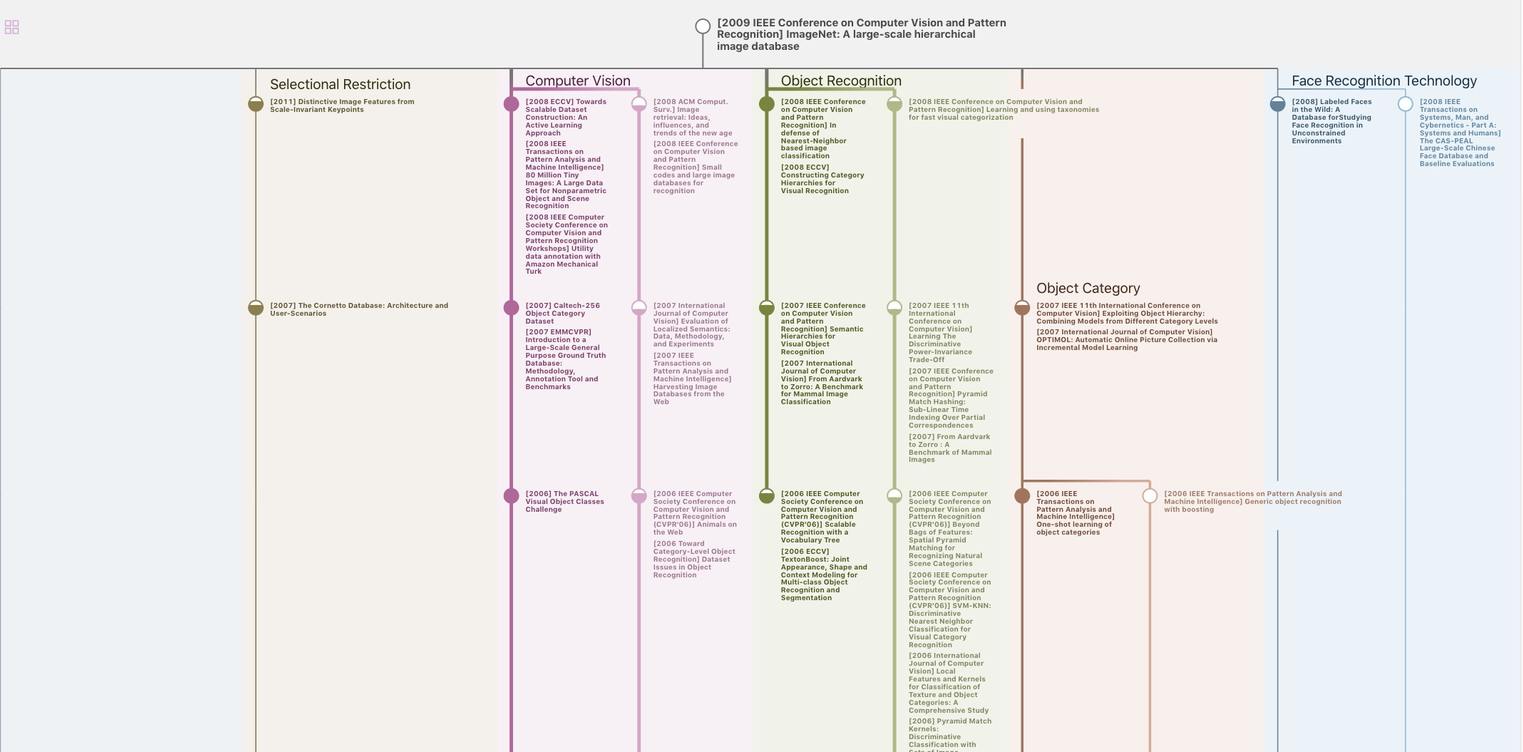
生成溯源树,研究论文发展脉络
Chat Paper
正在生成论文摘要