Challenges for Responsible AI Design and Workflow Integration in Healthcare: A Case Study of Automatic Feeding Tube Qualification in Radiology
arxiv(2024)
摘要
Nasogastric tubes (NGTs) are feeding tubes that are inserted through the nose
into the stomach to deliver nutrition or medication. If not placed correctly,
they can cause serious harm, even death to patients. Recent AI developments
demonstrate the feasibility of robustly detecting NGT placement from Chest
X-ray images to reduce risks of sub-optimally or critically placed NGTs being
missed or delayed in their detection, but gaps remain in clinical practice
integration. In this study, we present a human-centered approach to the problem
and describe insights derived following contextual inquiry and in-depth
interviews with 15 clinical stakeholders. The interviews helped understand
challenges in existing workflows, and how best to align technical capabilities
with user needs and expectations. We discovered the trade-offs and complexities
that need consideration when choosing suitable workflow stages, target users,
and design configurations for different AI proposals. We explored how to
balance AI benefits and risks for healthcare staff and patients within broader
organizational and medical-legal constraints. We also identified data issues
related to edge cases and data biases that affect model training and
evaluation; how data documentation practices influence data preparation and
labelling; and how to measure relevant AI outcomes reliably in future
evaluations. We discuss how our work informs design and development of AI
applications that are clinically useful, ethical, and acceptable in real-world
healthcare services.
更多查看译文
AI 理解论文
溯源树
样例
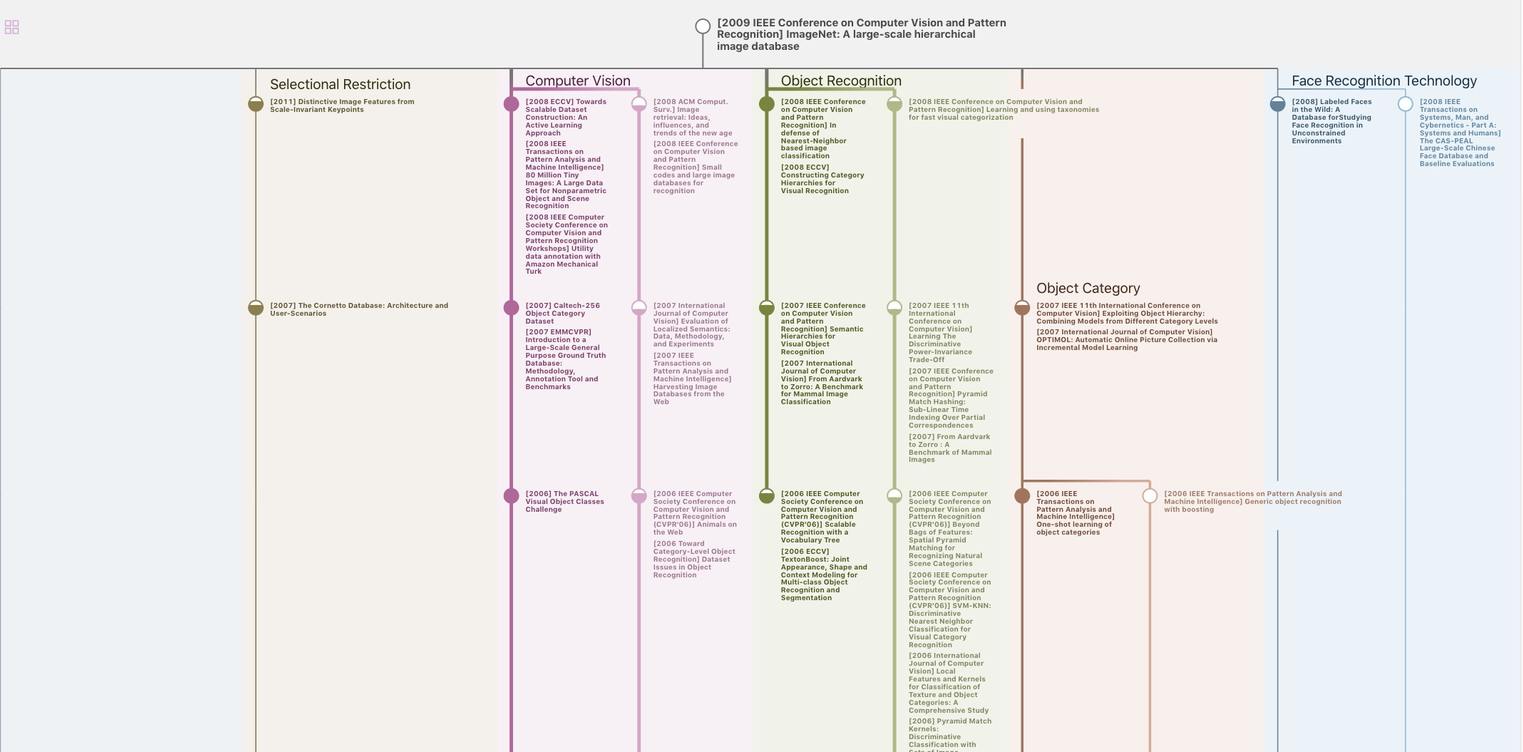
生成溯源树,研究论文发展脉络
Chat Paper
正在生成论文摘要