SciQAG: A Framework for Auto-Generated Scientific Question Answering Dataset with Fine-grained Evaluation
arxiv(2024)
摘要
The use of question-answer (QA) pairs for training and evaluating large
language models (LLMs) has attracted considerable attention. Yet few available
QA datasets are based on knowledge from the scientific literature. Here we
bridge this gap by presenting Automatic Generation of Scientific Question
Answers (SciQAG), a framework for automatic generation and evaluation of
scientific QA pairs sourced from published scientific literature. We fine-tune
an open-source LLM to generate 960000 scientific QA pairs from full-text
scientific papers and propose a five-dimensional metric to evaluate the quality
of the generated QA pairs. We show via LLM-based evaluation that the generated
QA pairs consistently achieve an average score of 2.5 out of 3 across five
dimensions, indicating that our framework can distill key knowledge from papers
into high-quality QA pairs at scale. We make the dataset, models, and
evaluation codes publicly available.
更多查看译文
AI 理解论文
溯源树
样例
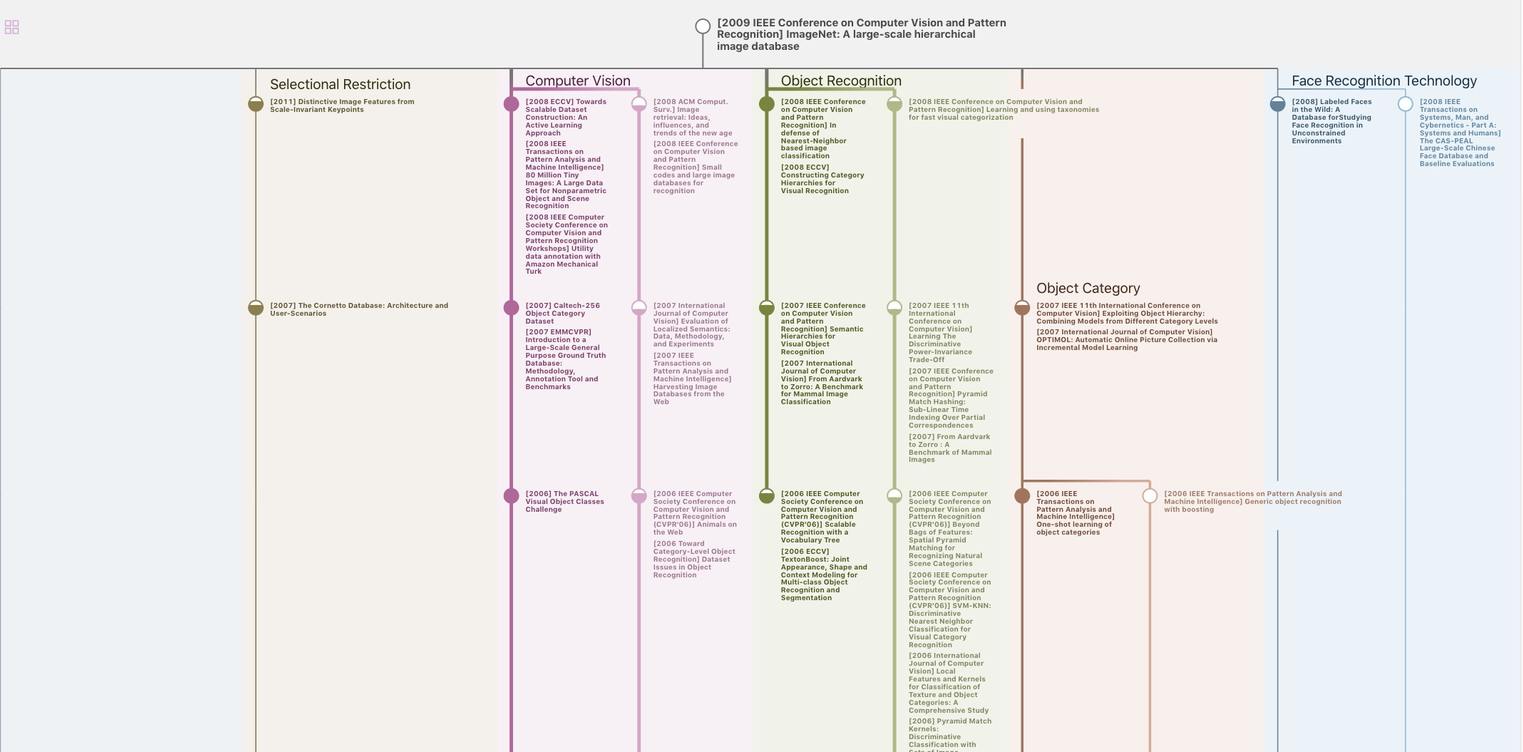
生成溯源树,研究论文发展脉络
Chat Paper
正在生成论文摘要