Medical steganography: Enhanced security and image quality, and new S-Q assessment
Signal Processing(2024)
摘要
Medical diagnosing systems generate sensitive information involving the patient’s privacy. Steganography supports securely embedding secrecy, guaranteeing that an adversary cannot distinguish cover images and stego images. However, directly applying natural-image steganography in medicine significantly affects efficacy and security. Until now, medical steganography is still in its infancy, mostly solving simple data-hiding policies, remaining barren in enhancing security.To fill this gap, we propose an end-to-end steganographic framework targeting protecting medical images efficiently. Our framework integrates medical-image adaptive steganography with a generative adversarial network named MedSteGAN. As for medical images without rich semantics and textures, we observe the heightened importance of involving both security and image quality. We shed light on designing a “security-quality (S-Q) assessment”, attaining comprehensive evaluation for all aspects. Following the S-Q assessment, we improve the overall practicality of medical steganography by (i) redesigning an effective U-shape generator network for removing the dependence on complex modules, (ii) improving feature extraction capability via convolutions with various kernel sizes and avoiding the checkerboard effect through upsampling layers, (iii) optimizing the loss function via the multi-scale structural similarity and high-frequency components. Extensive experiments demonstrate that MedSteGAN outperforms state-of-the-art steganographic schemes, showing the best overall results in the security-quality domain.
更多查看译文
AI 理解论文
溯源树
样例
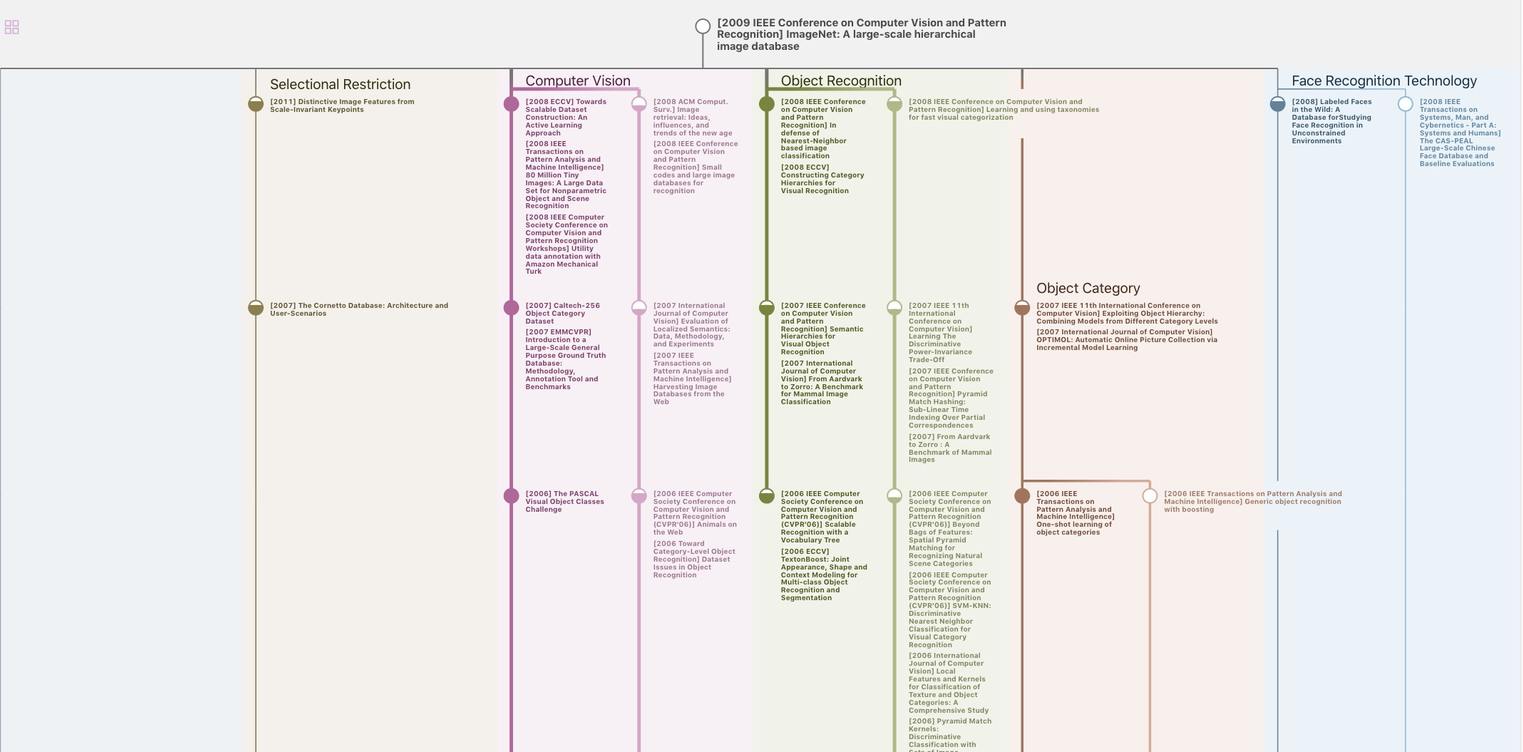
生成溯源树,研究论文发展脉络
Chat Paper
正在生成论文摘要