VQDNA: Unleashing the Power of Vector Quantization for Multi-Species Genomic Sequence Modeling
ICML 2024(2024)
摘要
Similar to natural language models, pre-trained genome language models areproposed to capture the underlying intricacies within genomes with unsupervisedsequence modeling. They have become essential tools for researchers andpractitioners in biology. However, the hand-crafted tokenizationpolicies used in these models may not encode the most discriminative patternsfrom the limited vocabulary of genomic data. In this paper, we introduce VQDNA,a general-purpose framework that renovates genome tokenization from theperspective of genome vocabulary learning. By leveraging vector-quantizedcodebook as learnable vocabulary, VQDNA can adaptively tokenizegenomes into pattern-aware embeddings in an end-to-end manner. Tofurther push its limits, we propose Hierarchical Residual Quantization (HRQ),where varying scales of codebooks are designed in a hierarchy to enrich thegenome vocabulary in a coarse-to-fine manner. Extensive experiments on 32genome datasets demonstrate VQDNA's superiority and favorable parameterefficiency compared to existing genome language models. Notably, empiricalanalysis of SARS-CoV-2 mutations reveals the fine-grained pattern awareness andbiological significance of learned HRQ vocabulary, highlighting its untappedpotential for broader applications in genomics.
更多查看译文
AI 理解论文
溯源树
样例
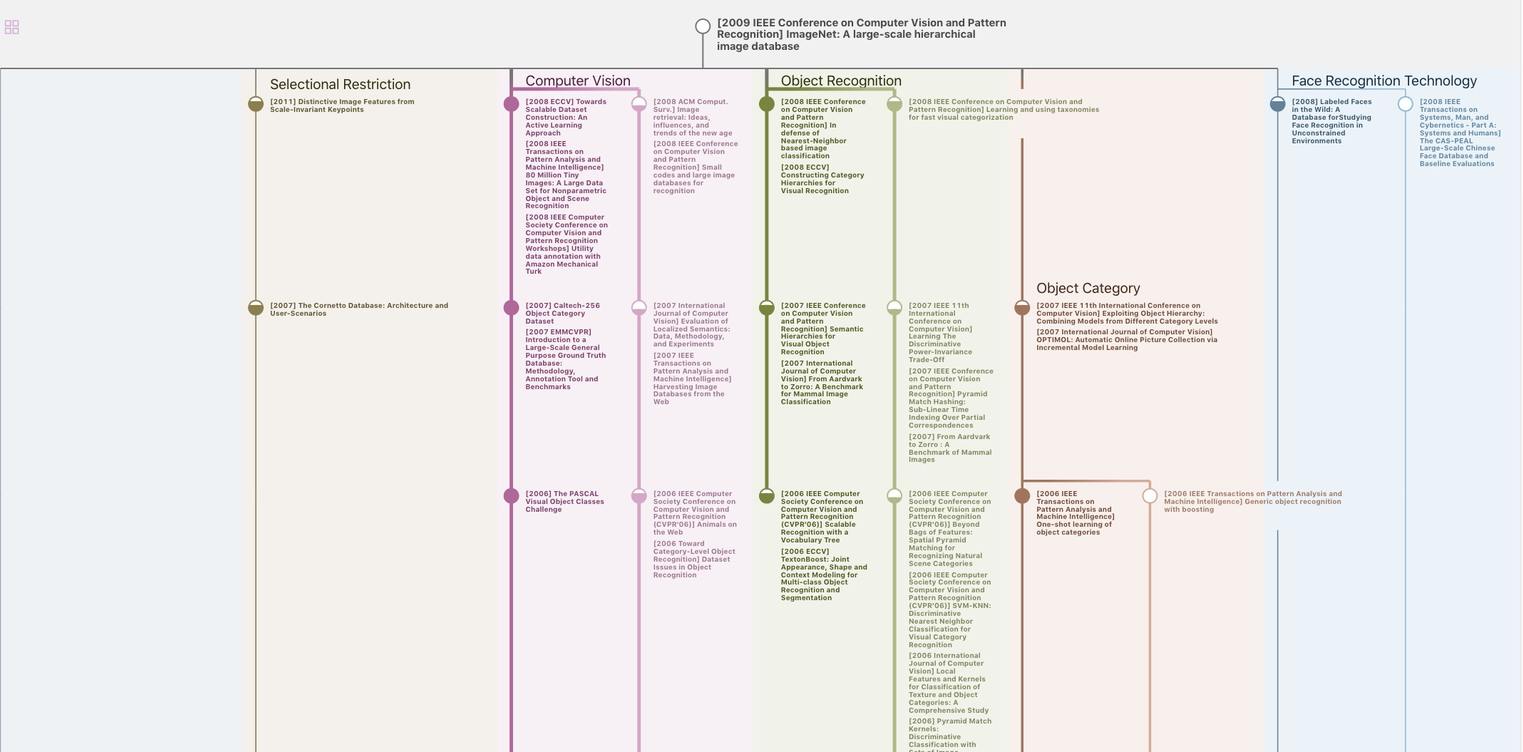
生成溯源树,研究论文发展脉络
Chat Paper
正在生成论文摘要