Adaptive super-resolution networks for single-pixel imaging at ultra-low sampling rates
IEEE Access(2024)
摘要
Single-pixel imaging (SPI) leverages sequential pattern illumination and intensity detection to reconstruct images, facing the challenge of balancing high-resolution output with ultra-low sampling rates for rapid imaging processes. We introduce a network architecture specifically tailored for SPI, which demonstrates improved performance even before integrating with SPI’s physical sampling processes. This integration, particularly focusing on the nuanced effects of sampling rates within the model’s loss function and data preprocessing, enhances image reconstruction quality and adaptability at low sampling rates, down to 1.56%. Our approach achieves a balance between advanced computational methods and the physical principles of SPI, resulting in a peak signal-to-noise ratio of 30.93 dB, a structural similarity index measure of 0.8818, and a perceptual index (PI) of 5.31 at a 6.25% sampling rate, alongside a notable PI of 2.68 at a 1.56% sampling rate in practical tests. By merging sophisticated network design with strategic integration of physical sampling rates, our model provides a refined solution for high-quality, high-resolution SPI at minimal sampling rates, facilitating progress in ultra-fast imaging applications.
更多查看译文
关键词
Single-pixel imaging,super-resolution,generative adversarial network,computational imaging,perceptual image-error assessment,ultra-low sampling rate,ultrafast imaging
AI 理解论文
溯源树
样例
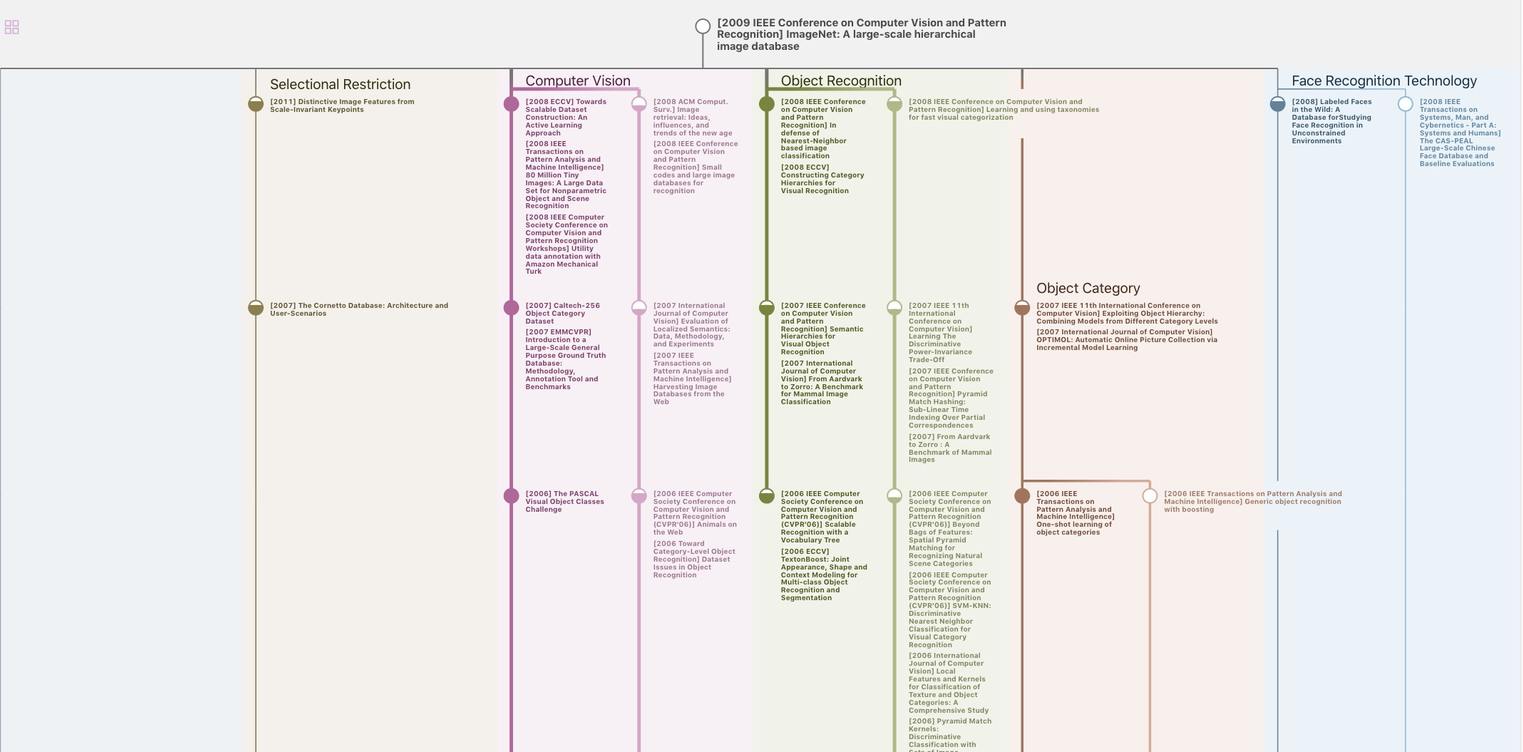
生成溯源树,研究论文发展脉络
Chat Paper
正在生成论文摘要