Learning Portable Skills by Identifying Generalizing Features with an Attention-Based Ensemble
ICLR 2023(2023)
摘要
The ability to rapidly generalize is crucial for reinforcement learning to be practical in real-world tasks. However, generalization is complicated by the fact that, in many settings, some state features reliably support generalization while others do not. We consider the problem of learning generalizable policies and skills (in the form of options) by identifying feature sets that generalize across instances. We propose an attention-ensemble approach, where a collection of minimally overlapping feature masks is learned, each of which individually maximizes performance on the source instance. Subsequent tasks are instantiated using the ensemble, and transfer performance is used to update the estimated probability that each feature set will generalize in the future. We show that our approach leads to fast policy generalization for eight tasks in the Procgen benchmark. We then show its use in learning portable options in Montezuma's Revenge, where it is able to generalize skills learned in the first screen to the remainder of the game.
更多查看译文
关键词
Hierarchical reinforcement learning,Skill transfer,Ensembling
AI 理解论文
溯源树
样例
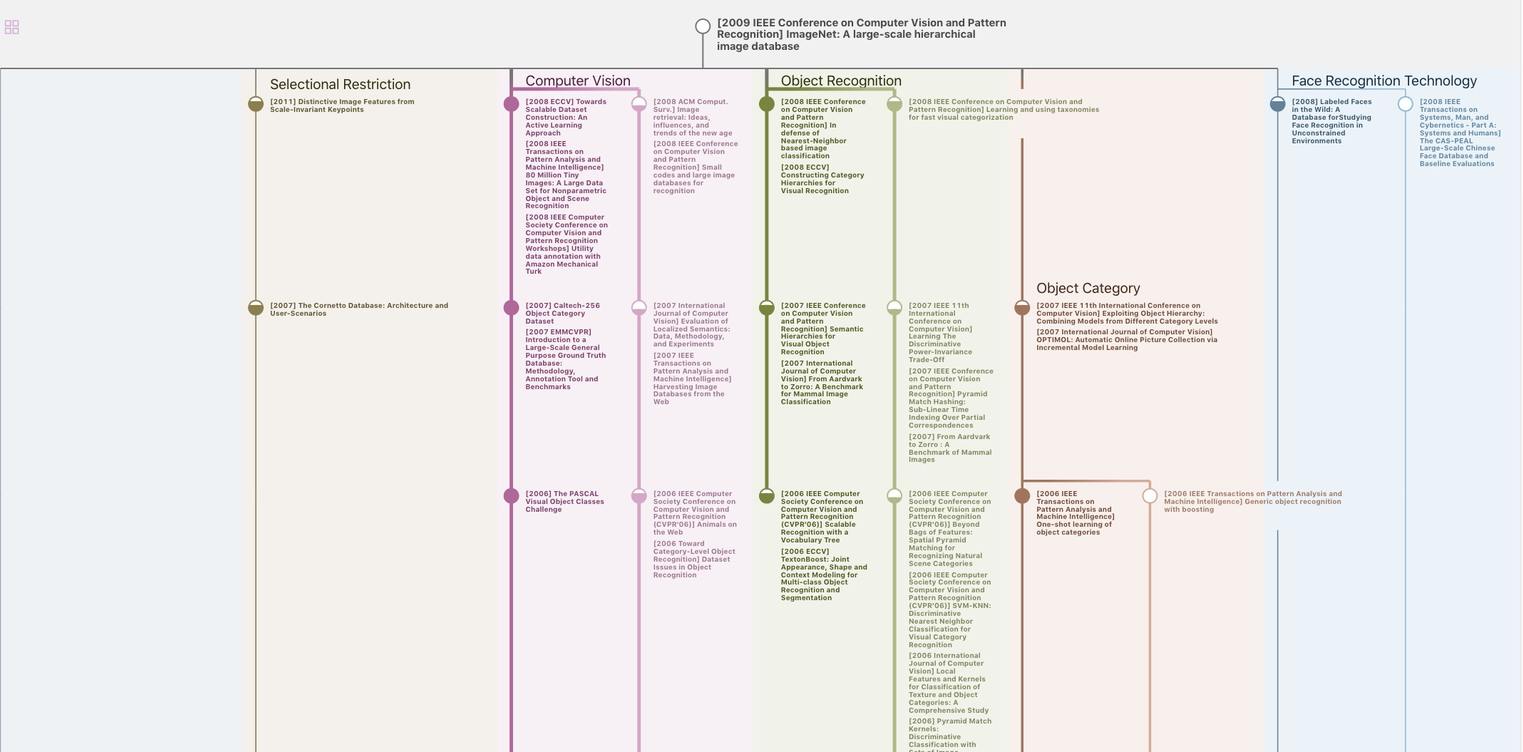
生成溯源树,研究论文发展脉络
Chat Paper
正在生成论文摘要