Turn ‘noise’ to signal: accurately rectify millions of erroneous short reads through graph learning on edit distances
biorxiv(2024)
摘要
Although the per-base erring rate of NGS is very low at 0.1% to 0.5%, the percentage/probability of erroneous reads in a short-read sequencing dataset can be as high as 10% to 15% or in the number of millions. Correction of these wrongly sequenced reads to retrieve their huge missing value will improve many downstream applications. As current methods correct only some of the errors at the cost of introducing many new errors, we solve this problem by turning erroneous reads into their original states, without bringing up any non-existing reads to keep the data integrity. The novelty of our method is originated in a computable rule translated from PCR erring mechanism that: a rare read is erroneous if it has a neighbouring read of high abundance. With this principle, we construct a graph to link every pair of reads of tiny edit distances to detect a solid part of erroneous reads; then we consider them as training data to learn the erring mechanisms to identify possibly remaining hard-case errors between pairs of high-abundance reads. Compared with state-of-the-art methods on tens of datasets of UMI-based ground truth, our method has made a remarkably better performance under 19 metrics including two entropy metrics that measure noise levels in a dataset. Case studies found that our method can make substantial impact on genome abundance quantification, isoform identification, SNP profiling, and genome editing efficiency estimation. For example, the abundance level of the reference genome of SARS-CoV-2 can be increased by 12% and that of Monkeypox can be boosted by 52.12% after error correction. Moreover, the number of distinct isomiRs is decreased by 31.56%, unveiling there are so many previously identified isomiRs that are actually sequencing errors.
Author summary Detecting short-read sequencing errors and correcting the related erroneous reads is a long-standing problem in bioinformatics. Current error correction algorithms correct only small parts of the errors but simultaneously introduce thousands of non-existing sequences. We present a new method to rectify erroneous reads under 300 bp produced by PCR-involved miRNA-sequencing, small RNA sequencing, or paired-end RNA sequencing, regardless of platform or sample type. Our method is the first kind considering the PCR erring mechanism and machine learning technique to improve sequencing data quality by turning millions of erroneous short reads into their original state without bringing up any non-existing sequences into the read set. Our error correction method can make a significant impact on a wide range of cutting-edge downstream applications. The observations and advantages in the case studies lay down strong evidence to question the accuracies of current downstream research outcomes and open new avenues to conduct downstream analysis whenever short-read data are adopted.
### Competing Interest Statement
The authors have declared no competing interest.
更多查看译文
AI 理解论文
溯源树
样例
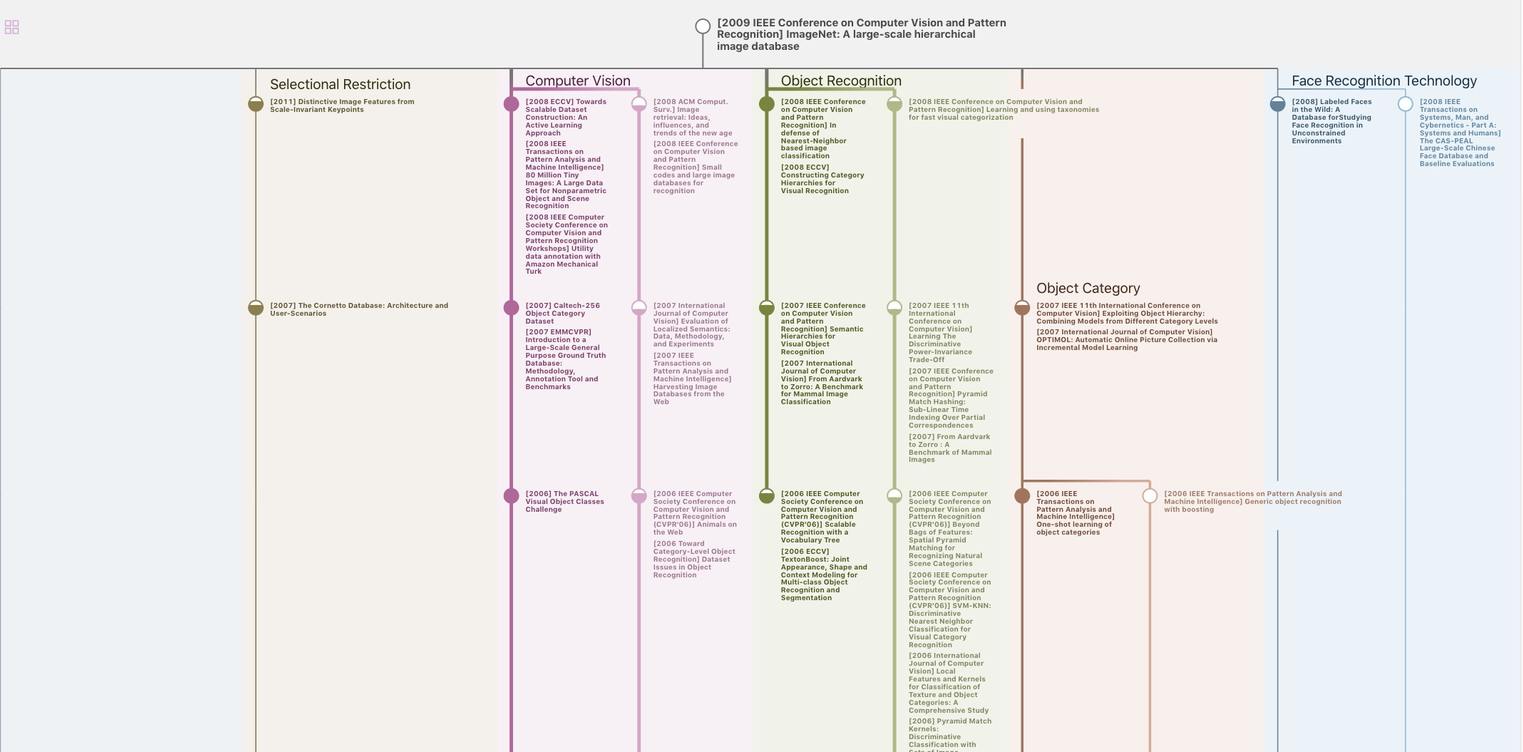
生成溯源树,研究论文发展脉络
Chat Paper
正在生成论文摘要