基本信息
浏览量:351
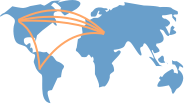
个人简介
Research Interests
My interests lie in challenges and limitations that arise when people theorize and draw inductive inferences from data. My research on uncertainty representation and interactive data analysis explores how to best align data-driven interfaces and summaries with human reasoning capabilities, how to understand the role of interactive analysis across different stages of a statistical workflow, how to evaluate data interfaces as well as the experiments researchers use to identify differences between them, and how to develop tools that support reasoning under uncertainty in domains like strategic games or privacy. I approach many of these problems by drawing on formal models of rational inference as a basis for comparison and proposing solutions.
My interests lie in challenges and limitations that arise when people theorize and draw inductive inferences from data. My research on uncertainty representation and interactive data analysis explores how to best align data-driven interfaces and summaries with human reasoning capabilities, how to understand the role of interactive analysis across different stages of a statistical workflow, how to evaluate data interfaces as well as the experiments researchers use to identify differences between them, and how to develop tools that support reasoning under uncertainty in domains like strategic games or privacy. I approach many of these problems by drawing on formal models of rational inference as a basis for comparison and proposing solutions.
研究兴趣
论文共 115 篇作者统计合作学者相似作者
按年份排序按引用量排序主题筛选期刊级别筛选合作者筛选合作机构筛选
时间
引用量
主题
期刊级别
合作者
合作机构
The Behavioral and brain sciences (2024): e49-e49
CoRR (2024)
引用0浏览0EI引用
0
0
CoRRno. 1 (2024): 338-347
CoRR (2024)
引用0浏览0EI引用
0
0
Fumeng Yang, Mandi Cai, Chloe Mortenson, Hoda Fakhari,Ayse D. Lokmanoglu,Jessica Hullman,Steven Franconeri,Nicholas Diakopoulos, Erik C. Nisbet,Matthew Kay
CoRRno. 1 (2024): 208-218
CoRRno. 1 (2024): 934-943
CoRR (2024)
CoRRno. 1 (2024): 672-682
加载更多
作者统计
合作学者
合作机构
D-Core
- 合作者
- 学生
- 导师
数据免责声明
页面数据均来自互联网公开来源、合作出版商和通过AI技术自动分析结果,我们不对页面数据的有效性、准确性、正确性、可靠性、完整性和及时性做出任何承诺和保证。若有疑问,可以通过电子邮件方式联系我们:report@aminer.cn