基本信息
浏览量:199
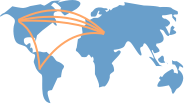
个人简介
My current research in machine learning focuses on convex models for learning predictive representation. Most existing machine learning methods infer representation from data in a way that is independent of its subsequent use, e.g. learning a predictive model. This is suboptimal. My research goal is to jointly infer latent representation and learn predictors for massive datasets by combining them into a single convex optimization problem. Convexity allows jointly optimal solutions to be found for these two tasks, and scale up efficiently to large application problems. To achieve this goal, my key strategies are: 1) find appropriate convex relaxations that retain the structure of the data, e.g. semi-definite relaxations; and 2) design efficient algorithms for optimization such as low-rank approximation.
研究兴趣
论文共 304 篇作者统计合作学者相似作者
按年份排序按引用量排序主题筛选期刊级别筛选合作者筛选合作机构筛选
时间
引用量
主题
期刊级别
合作者
合作机构
CoRR (2024)
引用0浏览0EI引用
0
0
2023 IEEE 11th International Conference on Healthcare Informatics (ICHI) (2023): 292-300
ICML 2023pp.15769-15785, (2023)
引用1浏览0EI引用
1
0
加载更多
作者统计
合作学者
合作机构
D-Core
- 合作者
- 学生
- 导师
数据免责声明
页面数据均来自互联网公开来源、合作出版商和通过AI技术自动分析结果,我们不对页面数据的有效性、准确性、正确性、可靠性、完整性和及时性做出任何承诺和保证。若有疑问,可以通过电子邮件方式联系我们:report@aminer.cn