On The Relationship Between Models For Learning In Helpful Environments
ICGI '00 Proceedings of the 5th International Colloquium on Grammatical Inference: Algorithms and Applications(2000)
摘要
The PAC and other equivalent learning models are widely accepted models for polynomial learnability of concept classes. However, negative results abound in the PAC learning framework (concept classes such as deterministic finite state automata (DFA) are not efficiently learnable in the PAC model). The PAC model's requirement of learnability under all conceivable distributions could be considered too stringent a restriction for practical applications. Several models for learning in more helpful environments have been proposed in the literature including: learning from example based queries [2], online learning allowing a bounded number of mistakes [14], learning with the help of teaching sets [7], learning from characteristic sets [5], and learning from simple examples [12,4]. Several concept classes that are not learnable in the standard PAC model have been shown to be learnable in these models. In this paper we identify the relationships between these different learning models. We also address the issue of unnatural collusion between the teacher and the learner that can potentially trivialize the task of learning in helpful environments.
更多查看译文
关键词
Models of learning,Query learning,Mistake bounded learning,PAC learning,teaching sets,characteristic samples,DFA learning
AI 理解论文
溯源树
样例
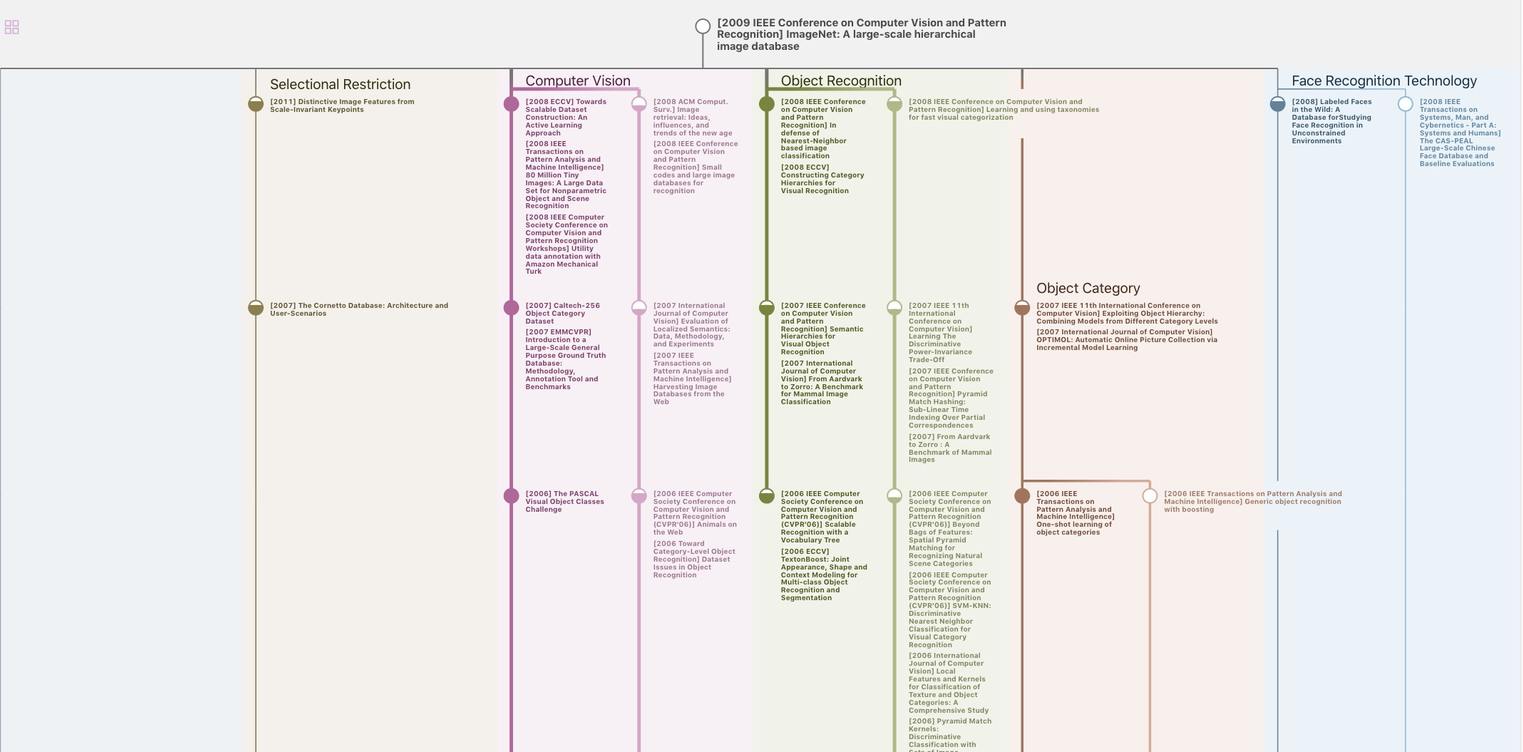
生成溯源树,研究论文发展脉络
Chat Paper
正在生成论文摘要