Robust Probabilistic Projections.
ICML '06 Proceedings of the 23rd international conference on Machine learning(2006)
摘要
Principal components and canonical correlations are at the root of many exploratory data mining techniques and provide standard pre-processing tools in machine learning. Lately, probabilistic reformulations of these methods have been proposed (Roweis, 1998; Tipping & Bishop, 1999b; Bach & Jordan, 2005). They are based on a Gaussian density model and are therefore, like their non-probabilistic counterpart, very sensitive to atypical observations. In this paper, we introduce robust probabilistic principal component analysis and robust probabilistic canonical correlation analysis. Both are based on a Student- t density model. The resulting probabilistic reformulations are more suitable in practice as they handle outliers in a natural way. We compute maximum likelihood estimates of the parameters by means of the EM algorithm.
更多查看译文
AI 理解论文
溯源树
样例
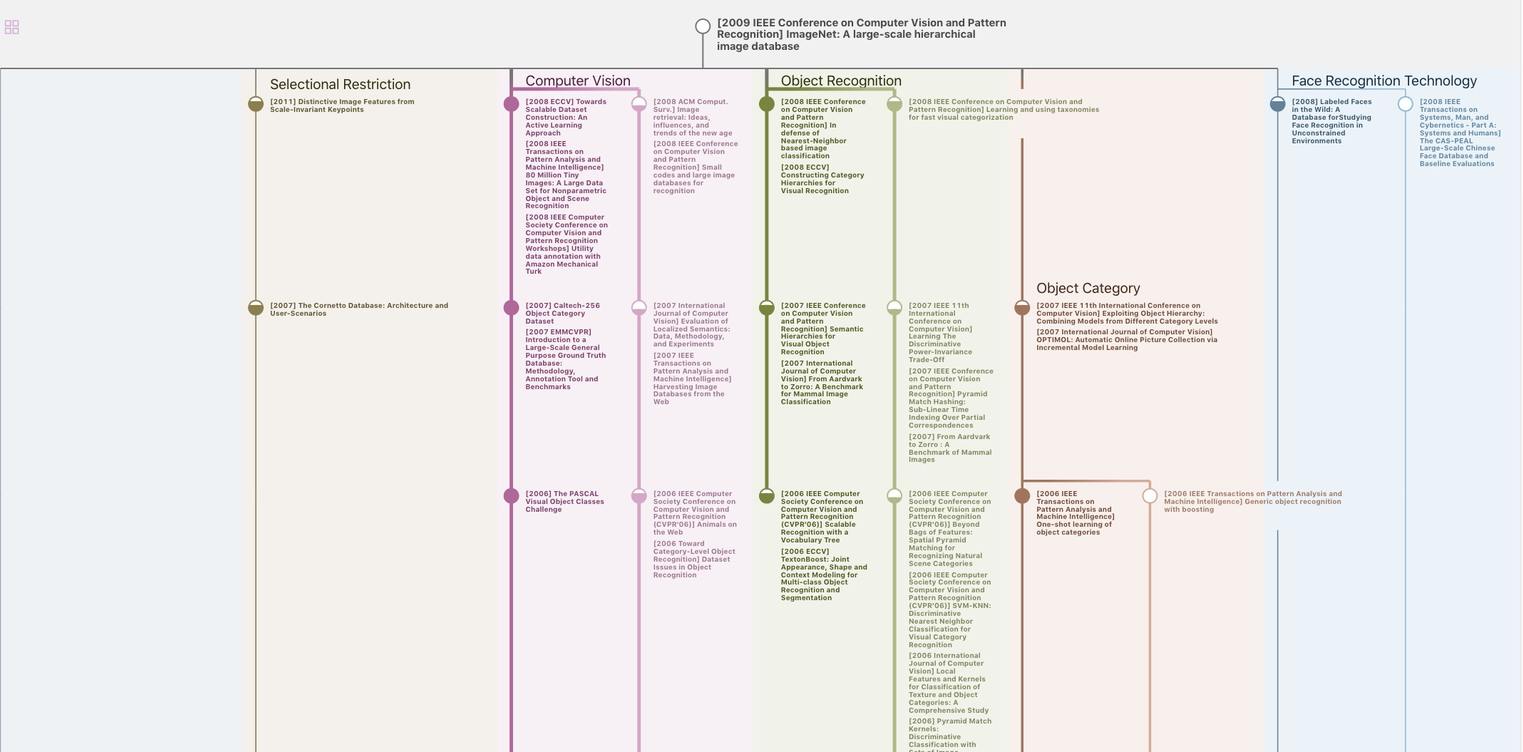
生成溯源树,研究论文发展脉络
Chat Paper
正在生成论文摘要