Selection of the optimal parameter value for the Isomap algorithm
MICAI 2005: ADVANCES IN ARTIFICIAL INTELLIGENCE(2006)
摘要
The isometric feature mapping (Isomap) method has demonstrated promising results in finding low-dimensional manifolds from data points in high-dimensional input space. Isomap has one free parameter (number of nearest neighbours K or neighbourhood radius @e), which has to be specified manually. In this paper we present a new method for selecting the optimal parameter value for Isomap automatically. Numerous experiments on synthetic and real data sets show the effectiveness of our method.
更多查看译文
关键词
nearest neighbour,isomap algorithm,high-dimensional data,neighbourhood radius,manifold learning,free parameter,neighborhood size,data point,dijkstra-like shortest path algorithm,high-dimensional input space,optimal parameter value,nonlinear dimensionality reduction,neighbors method,suitable neighborhood size,effective method,high-dimensional space,straightforward method,isometric feature mapping,euclidean distance metric,isomap,new method,low-dimensional manifold
AI 理解论文
溯源树
样例
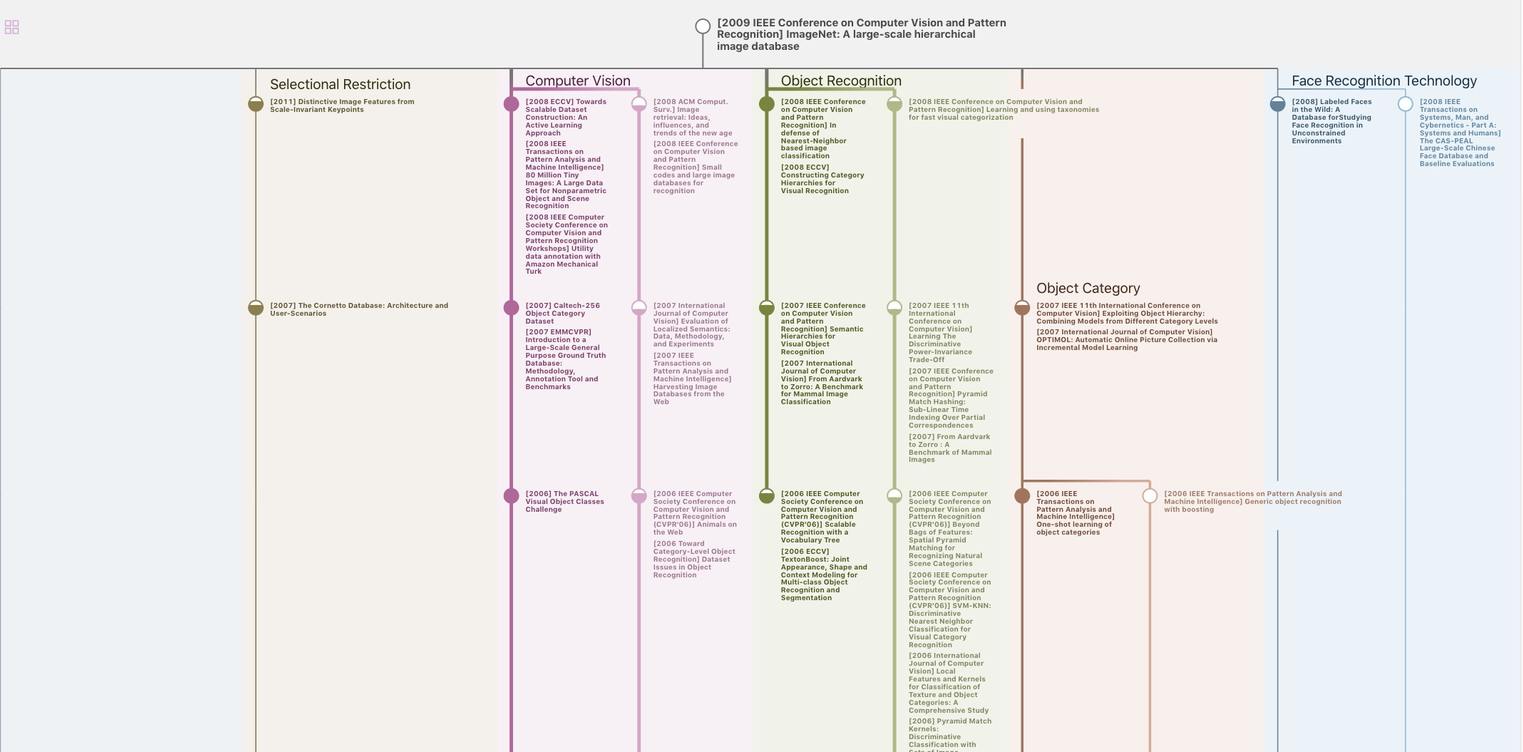
生成溯源树,研究论文发展脉络
Chat Paper
正在生成论文摘要