Backoff model training using partially observed data: application to dialog act tagging
HLT-NAACL(2006)
摘要
Dialog act (DA) tags are useful for many applications in natural language processing and automatic speech recognition. In this work, we introduce hidden backoff models (HBMs) where a large generalized backoff model is trained, using an embedded expectation-maximization (EM) procedure, on data that is partially observed. We use HBMs as word models conditioned on both DAs and (hidden) DA-segments. Experimental results on the ICSI meeting recorder dialog act corpus show that our procedure can strictly increase likelihood on training data and can effectively reduce errors on test data. In the best case, test error can be reduced by 6.1% relative to our baseline, an improvement on previously reported models that also use prosody. We also compare with our own prosody-based model, and show that our HBM is competitive even without the use of prosody. We have not yet succeeded, however, in combining the benefits of both prosody and the HBM.
更多查看译文
关键词
act corpus show,icsi meeting recorder dialog,training data,observed data,backoff model,act tagging,dialog act,test data,own prosody-based model,test error,backoff model training,large generalized backoff model,word model,natural language processing,expectation maximization,automatic speech recognition
AI 理解论文
溯源树
样例
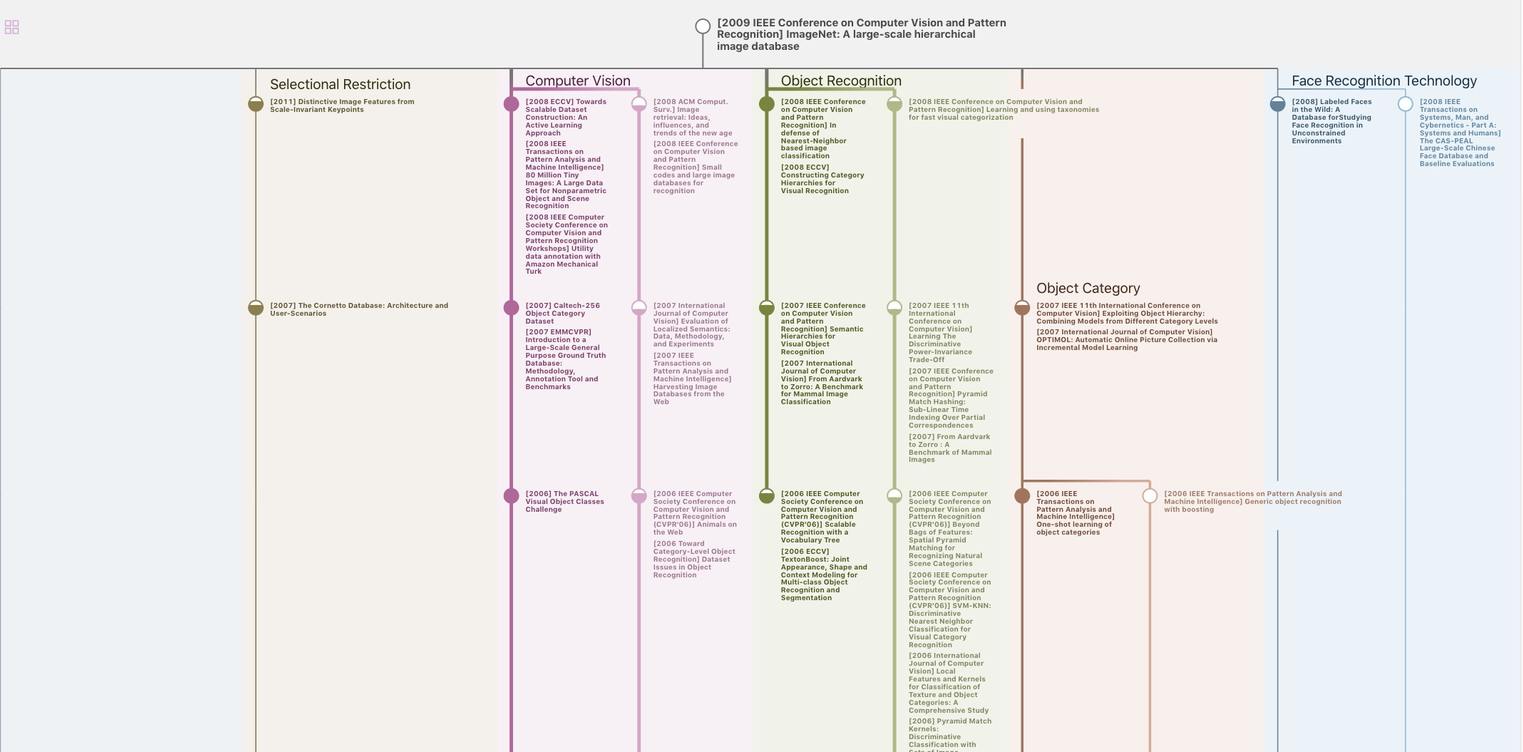
生成溯源树,研究论文发展脉络
Chat Paper
正在生成论文摘要