Similarity-based analysis for large networks of ultra-low resolution sensors
Pattern Recognition(2006)
摘要
By analyzing the similarities between bit streams coming from a network of motion detectors, we can recover the network geometry and discover structure in the human behavior being observed. This means that a low-cost network of sensors can provide powerful contextual information to building systems: improving the efficiency of elevators, lighting, heating, and cooling; enhancing safety and security; and opening up new opportunities for human-centered information systems. This paper will show how signal similarity can be used to calibrate a sensor network to accuracies below the resolution of the individual sensors. This is done by analyzing the similarity structures in the unconstrained movement of people in the observed space. We will also present our efficient behavior-learning algorithm that yields 90% correct behavior-detection in data from a sensor network comprised of motion detectors by employing similarity-based clustering to automatically decompose complex activities into detectable sub-classes.
更多查看译文
关键词
individual sensor,ultra-low resolution sensor,low-cost network,similarity-based analysis,observed space,human-centered information system,powerful contextual information,similarity structure,motion detector,sensor network,network geometry,signal similarity,large network,sensor networks,human behavior,information system,localization,behavior,low resolution,similarity
AI 理解论文
溯源树
样例
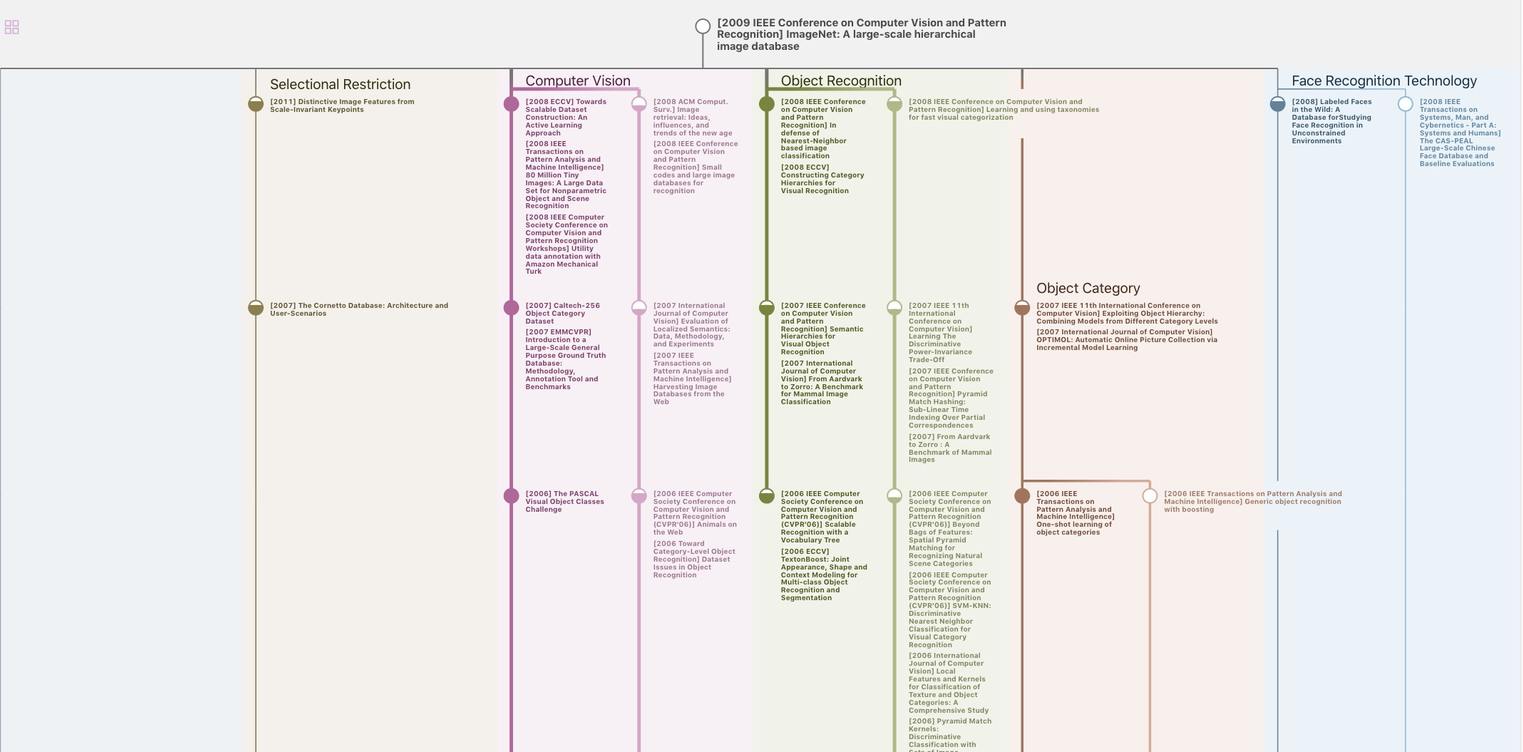
生成溯源树,研究论文发展脉络
Chat Paper
正在生成论文摘要