Two Phase Parallel Particle Swarm Algorithm based on Regional and Social Study of Object Optimization 1
ICNC 2007: THIRD INTERNATIONAL CONFERENCE ON NATURAL COMPUTATION, VOL 3, PROCEEDINGS(2007)
摘要
This paper proposed a new Two Phase Parallel Particle Swarm Optimization(TP_PPSO) Algorithm based on region and social study of multi-object optimization in economic data analysis and mining. This study(1)changes the traditional particle information sharing mechanism and introduces region study for making that particles can reference other particles information when they updates; and divides particles groups evolution into two classes optimization by the advantage of divided-group strategy .(2)partitions particles swarm evolution into two phase. One phase is propitious to expand the scope of the search and another phase is conducive to the fine overall search. (3)proposes an disturbance strategy Which the best particles in each particles swarm will be initialized in locally around and other particles will initialized in the overall scope, when the un-updated population of global optimal solution is bigger than certain population. (4)proves the convergence of PSO and convergence condition and the rationality of TP_PPSO. (5)demonstrates that of TP_PPSO has improved the accuracy of the solution 60% at least than the classical parallel particles swarm algorithm - IPPSO by optimization and contrastive experiments using five functions.
更多查看译文
关键词
particles groups evolution,parallel particle swarm algorithm,object optimization,partitions particles swarm evolution,classes optimization,region study,particles swarm,particles information,certain population,social study,multi-object optimization,classical parallel particles swarm,multi objective optimization,global optimization,data mining,data analysis,social studies,particle swarm
AI 理解论文
溯源树
样例
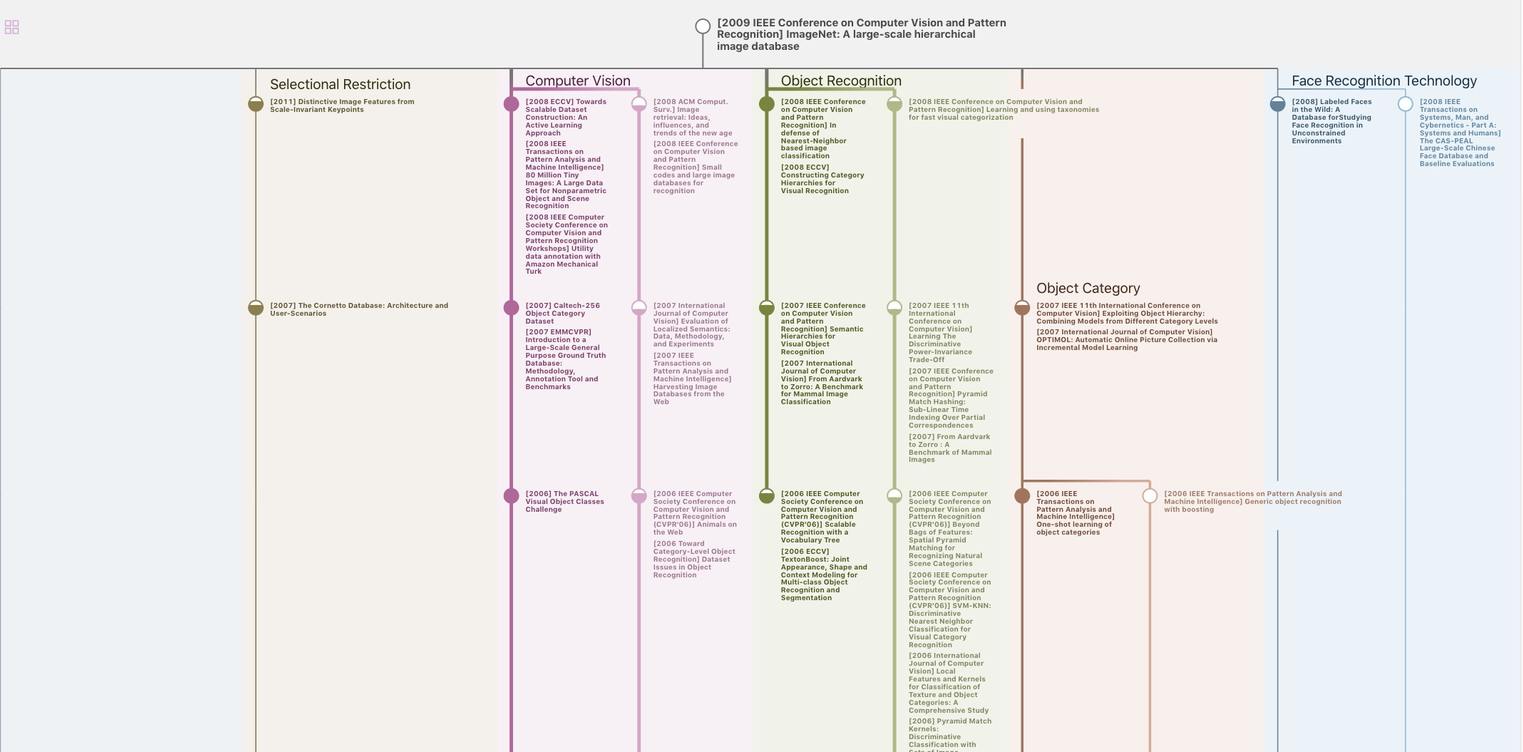
生成溯源树,研究论文发展脉络
Chat Paper
正在生成论文摘要