Recursive prediction for long term time series forecasting using advanced models
Neurocomputing(2007)
摘要
There exists a wide range of paradigms, and a high number of different methodologies that are applied to the problem of time series prediction. Most of them are presented as a modified function approximation problem using input/output data, in which the input data are expanded using values of the series at previous steps. Thus, the model obtained normally predicts the value of the series at a time (t+h) using previous time steps (t-@t"1),(t-@t"2),...,(t-@t"n). Nevertheless, learning a model for long term time series prediction might be seen as a more complicated task, since it might use its own outputs as inputs for long term prediction (recursive prediction). This paper presents the utility of two different methodologies, the TaSe fuzzy TSK model and the least-squares SVMs, to solve the problem of long term time series prediction using recursive prediction. This work also introduces some techniques that upgrade the performance of those advanced one-step-ahead models (and in general of any one-step-ahead model), where they are used recursively for long term time series prediction.
更多查看译文
关键词
long term time series,one-step-ahead model,different methodology,advanced model,tase fuzzy tsk model,advanced one-step-ahead model,recursive prediction,previous time step,modified function approximation problem,long term prediction,time series prediction,least square,fuzzy system,function approximation,input output,fuzzy systems,time series forecasting
AI 理解论文
溯源树
样例
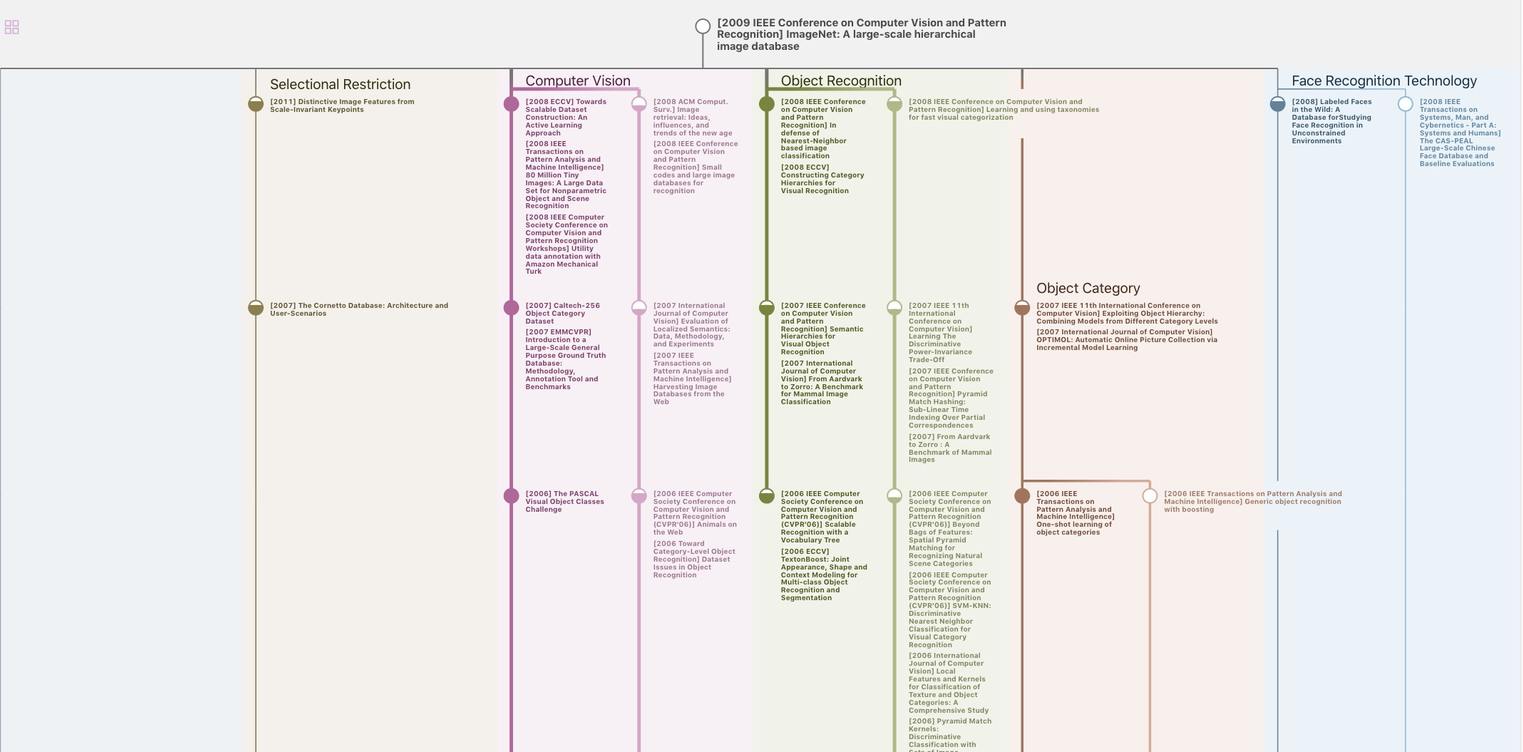
生成溯源树,研究论文发展脉络
Chat Paper
正在生成论文摘要