Artificial neural network modelling to predict cuticle cracking in greenhouse peppers and tomatoes
Computers and Electronics in Agriculture(2008)
摘要
Cuticle cracking in pepper and tomato fruit was studied in relation to fruit development and modelled with respect to environmental and cultural conditions. All data were collected over a 26-week period from seven commercial greenhouses in British Columbia, Canada. In terms of fruit development, cuticle cracking began one week after the period of maximum fruit growth rate in pepper and progressed the most rapidly over the next two weeks. In tomato, cracking started two weeks after the time of maximum fruit growth rate, increasing steadily thereafter until harvest. Feed-forward artificial neural networks were used to relate cuticle cracking to greenhouse and crop conditions. The incidence of cuticle cracking was initially related to over 20 input variables averaged over one or four weeks prior to the date of cracking assessment. All models were developed using a portion of the data and validated with the remainder. For each dataset, 30 models were analyzed at a time, from which the best models were chosen on the basis of a suitable architecture with a high R2 value and a low root mean square error when tested against the validation set using a 10-fold cross-validation procedure. Sensitivity analysis was used to determine the relative importance of each input and to reduce the number of inputs. The best week-1 and week-4 models for pepper consisted of nine inputs, with R2 values of 0.71 and 0.70, respectively. Day and night temperature, day-time CO2, day and night relative humidity, radiation and growing media were inputs held in common by both models. Growing media and cultivar were also important in the week-1 models while EC and week of the year (time) were important in the week-4 models. The best week-1 and week-4 models for tomato also used nine inputs, with R2 values of 0.70 and 0.64, respectively. In this case, week of the year, day and night relative humidity, day and night temperature, yield and the number of leaves were common to both models. Fruit size and fruit mass were also important in the week-1 models, while daytime CO2 and radiation were important in the week-4 models. The study shows that artificial neural networks can be used to predict cuticle cracking in greenhouse peppers and tomatoes up to four weeks in advance of harvest.
更多查看译文
关键词
Artificial neural networks,Cuticle cracking,Greenhouse,Pepper,Russeting,Tomato
AI 理解论文
溯源树
样例
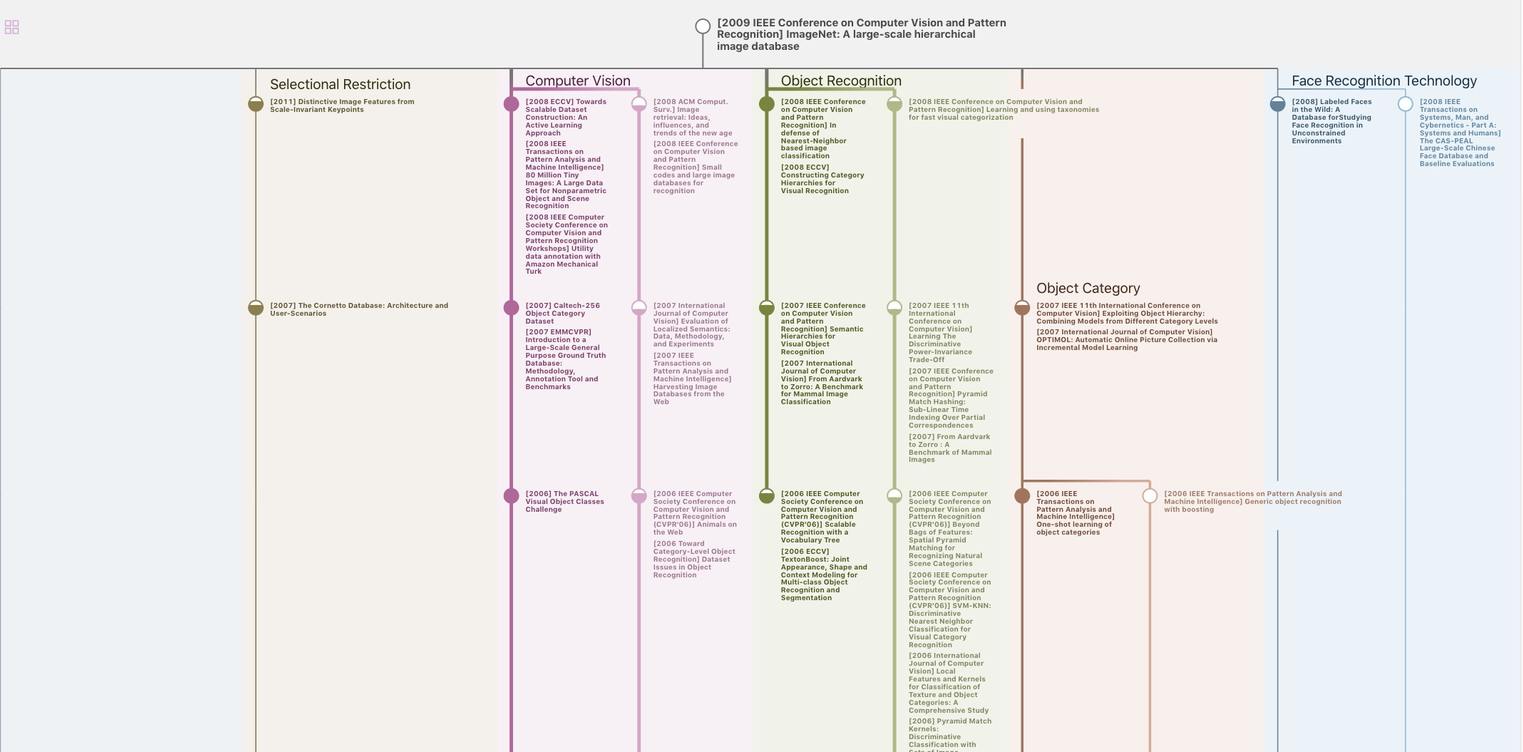
生成溯源树,研究论文发展脉络
Chat Paper
正在生成论文摘要