Mixtures of robust probabilistic principal component analyzers
Neurocomputing(2008)
摘要
Mixtures of probabilistic principal component analyzers model high-dimensional nonlinear data by combining local linear models. Each mixture component is specifically designed to extract the local principal orientations in the data. An important issue with this generative model is its sensitivity to data lying off the low-dimensional manifold. In order to address this problem, the mixtures of robust probabilistic principal component analyzers are introduced. They take care of atypical points by means of a long tail distribution, the Student-t. It is shown that the resulting mixture model is an extension of the mixture of Gaussians, suitable for both robust clustering and dimensionality reduction. Finally, we briefly discuss how to construct a robust version of the closely related mixture of factor analyzers.
更多查看译文
关键词
principal component analysis,robustness to outliers,local principal orientation,model high-dimensional nonlinear data,robust probabilistic principal component,robust clustering,mixture component,resulting mixture model,related mixture,probabilistic principal component analyzer,non-gaussianity,mixture model,dimensionality reduction,generative model,local linear model,em algorithm,linear model,long tail,mixture of gaussians,principal component,non gaussianity
AI 理解论文
溯源树
样例
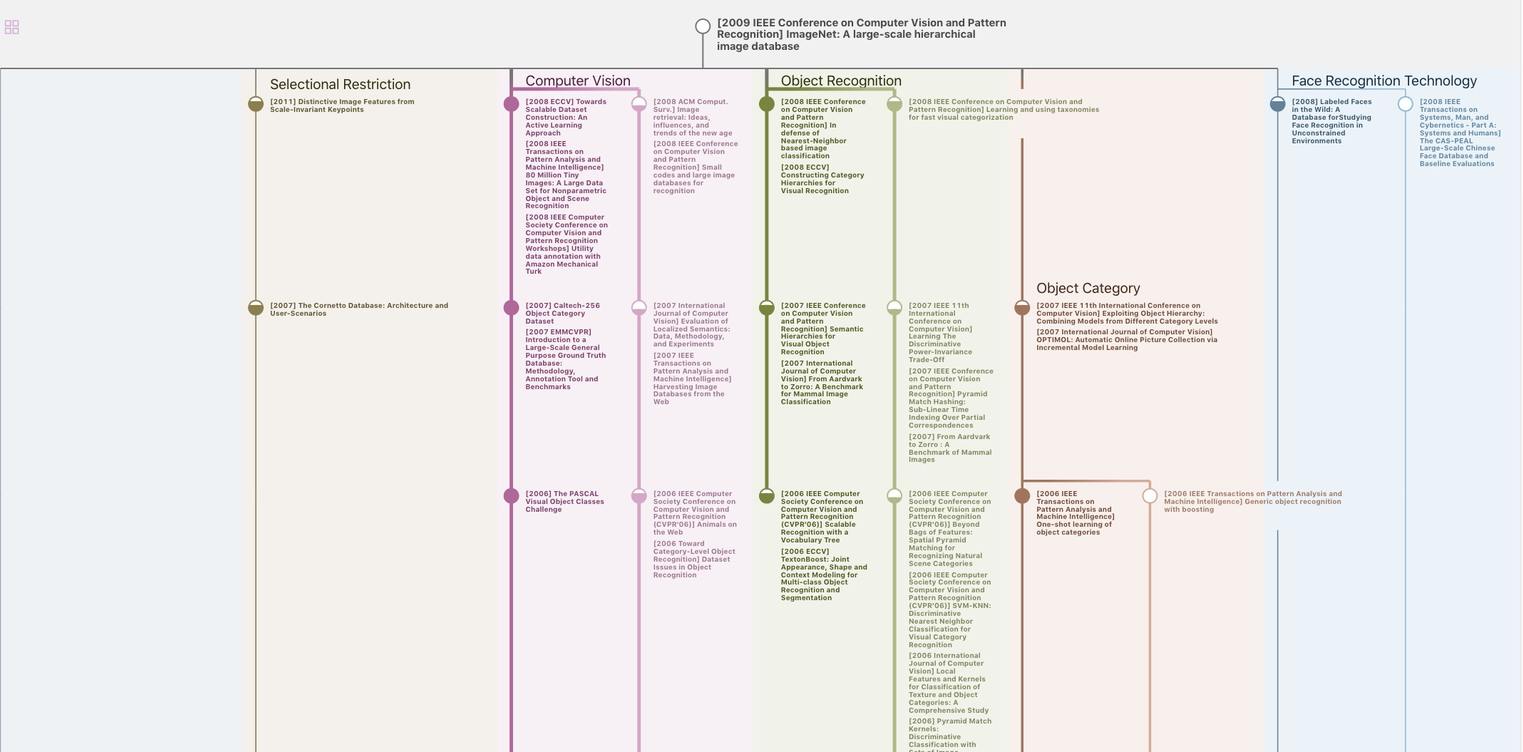
生成溯源树,研究论文发展脉络
Chat Paper
正在生成论文摘要