Solving Decentralized Continuous Markov Decision Problems with Structured Reward
KI 2007: Advances in Artificial Intelligence, Proceedings(2007)
摘要
We present an approximation method that solves a class of Decentralized hybrid Markov Decision Processes (DEC-HMDPs). These DEC-HMDPs have both discrete and continuous state variables and represent individual agents with continuous measurable state-space, such as resources. Adding to the natural complexity of decentralized problems, continuous state variables lead to a blowup in potential decision points. Representing value functions as Rectangular Piecewise Constant (RPWC) functions, we formalize and detail an extension to the Coverage Set Algorithm (CSA) [1] that solves transition independent DEC-HMDPs with controlled error. We apply our algorithm to a range of multi-robot exploration problems with continuous resource constraints.
更多查看译文
关键词
decentralized continuous markov decision,decentralized hybrid markov decision,rectangular piecewise,continuous state variable,controlled error,representing value function,continuous measurable state-space,approximation method,coverage set algorithm,transition independent dec-hmdps,continuous resource constraint,state space,markov decision process,value function
AI 理解论文
溯源树
样例
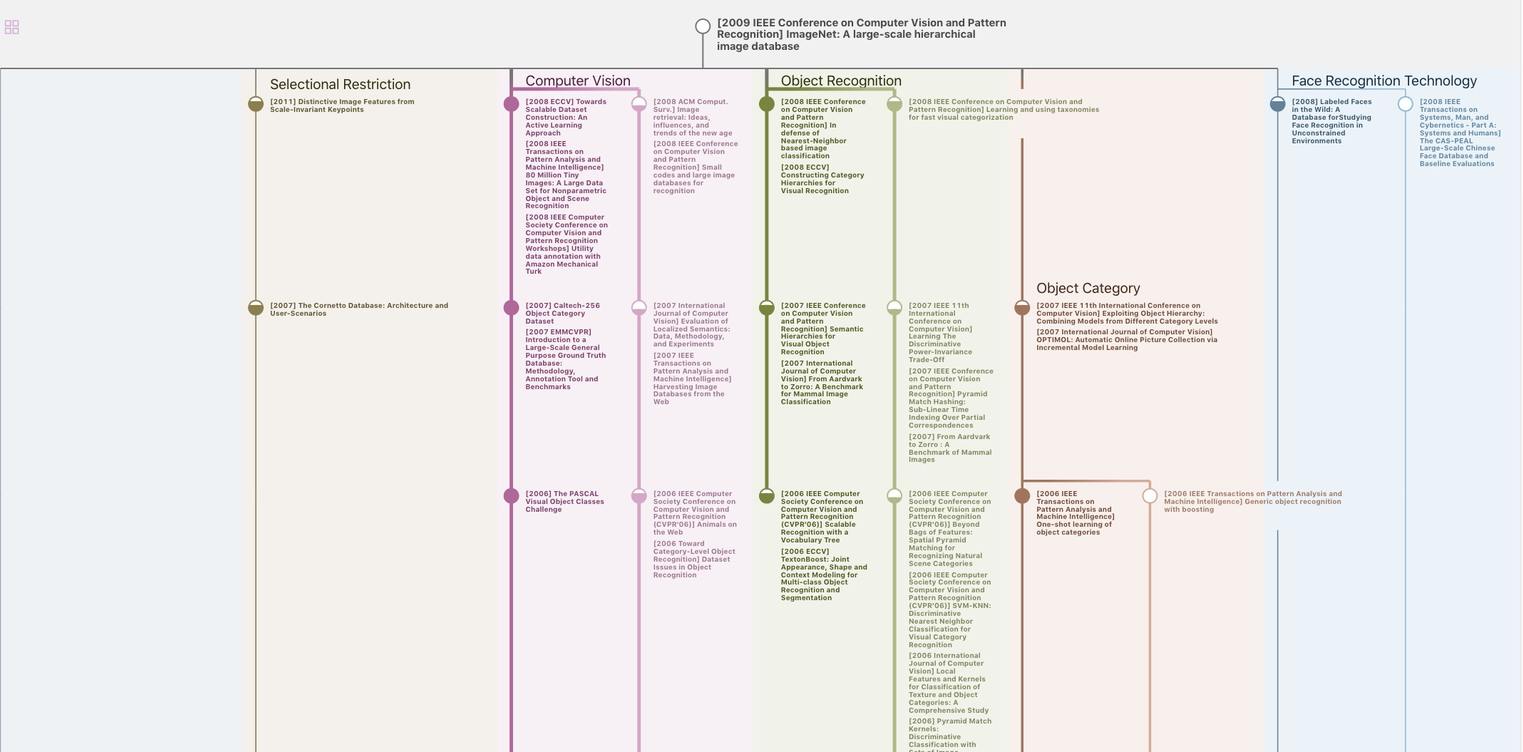
生成溯源树,研究论文发展脉络
Chat Paper
正在生成论文摘要