Natural Language Understanding by Combining Statistical Methods and Extended Context-Free Grammars
PATTERN RECOGNITION(2008)
摘要
This paper introduces an novel framework for speech understanding using extended context-free grammars (ECFGs) by combining statistical methods and rule based knowledge. By only using 1st level labels a considerable lower expense of annotation effort can be achieved. In this paper we derive hierarchical non-deterministic automata from the ECFGs, which are transformed into transition networks (TNs) representing all kinds of labels. A sequence of recognized words is hierarchically decoded by using a Viterbi algorithm. In experiments the difference between a hand-labeled tree bank annotation and our approach is evaluated. The conducted experiments show the superiority of our proposed framework. Comparing to a hand-labeled baseline system ($\widehat{=} 100\%$) we achieve 95,4 % acceptance rate for complete sentences and 97.8 % for words. This induces an accuray rate of 95.1 % and error rate of 4.9 %, respectively F1-measure 95.6 % in a corpus of 1 300 sentences.
更多查看译文
关键词
accuray rate,combining statistical methods,proposed framework,complete sentence,hand-labeled tree bank annotation,novel framework,hand-labeled baseline system,error rate,annotation effort,extended context-free grammars,viterbi algorithm,natural language understanding,acceptance rate,context free grammar,rule based
AI 理解论文
溯源树
样例
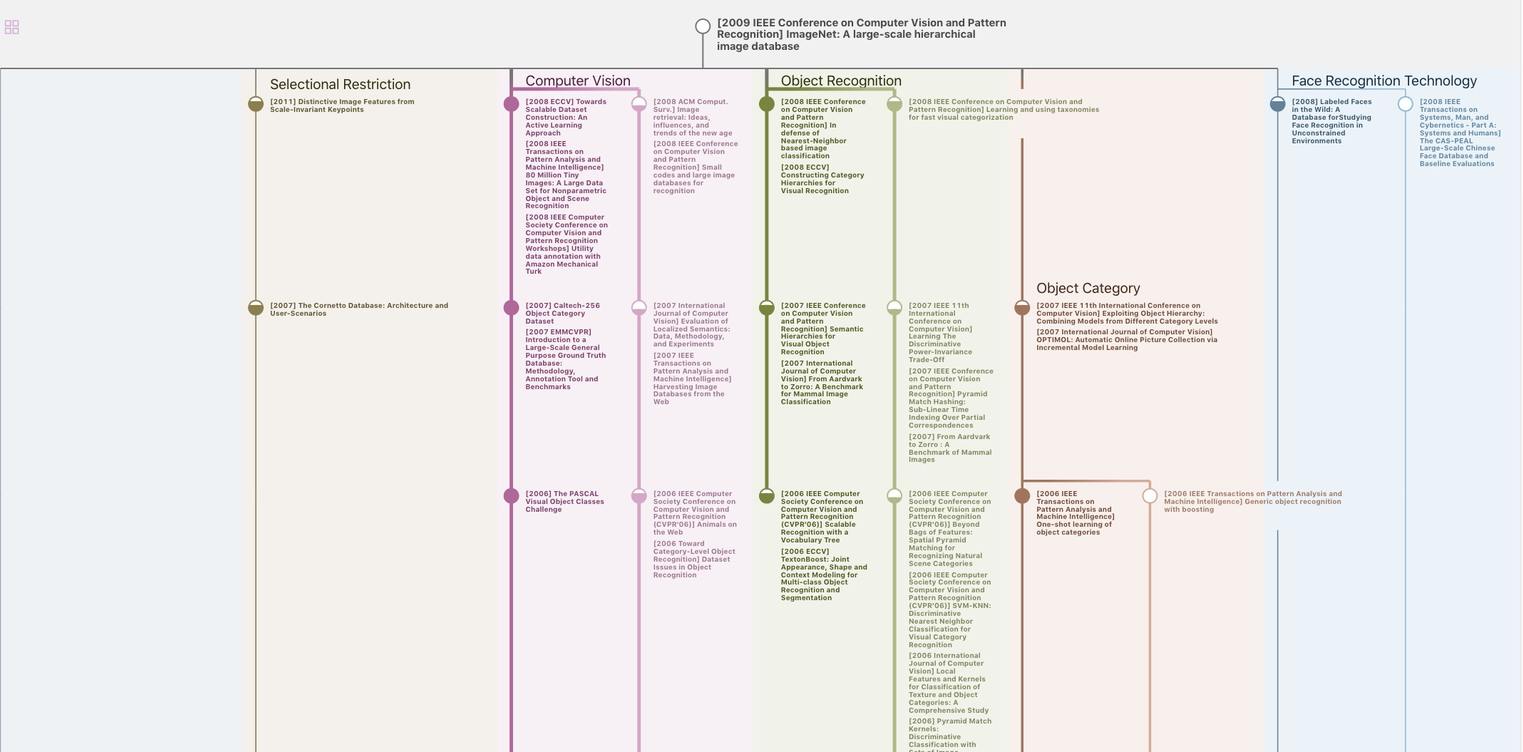
生成溯源树,研究论文发展脉络
Chat Paper
正在生成论文摘要