Workload-aware data partitioning in community-driven data grids
EDBT '09: Proceedings of the 12th International Conference on Extending Database Technology: Advances in Database Technology(2009)
摘要
Collaborative research in various scientific disciplines requires support for scalable data management enabling the efficient correlation of globally distributed data sources. Motivated by the expected data rates of upcoming projects and a growing number of users, communities explore new data management techniques for achieving high throughput. Community-driven data grids deliver such high-throughput data distribution for scientific federations by partitioning data according to application-specific data and query characteristics. Query hot spots are an important and challenging problem in this environment. Existing approaches to load-balancing from Peer-to-Peer (P2P) data management and sensor networks do not directly meet the requirements of a data-intensive e-science environment. In this paper, our contributions are partitioning schemes based on multi-dimensional index structures enabling communities to trade off data load balancing and handling query hot spots via splitting and replication. We evaluate the partitioning schemes with two typical kinds of data sets from the astrophysics domain and workloads extracted from Sloan Digital Sky Survey (SDSS) query traces and perform throughput measurements in real and simulated networks. The experiments demonstrate the improved workload distribution capabilities and give promising directions for the development of future community grids.
更多查看译文
关键词
community-driven data grid,workload-aware data,scalable data management,partitioning data,data load balancing,high-throughput data distribution,data source,new data management technique,expected data rate,data management,sensor network,data grid,p2p,data warehousing,load balance,business intelligence,hot spot,data integration,high throughput
AI 理解论文
溯源树
样例
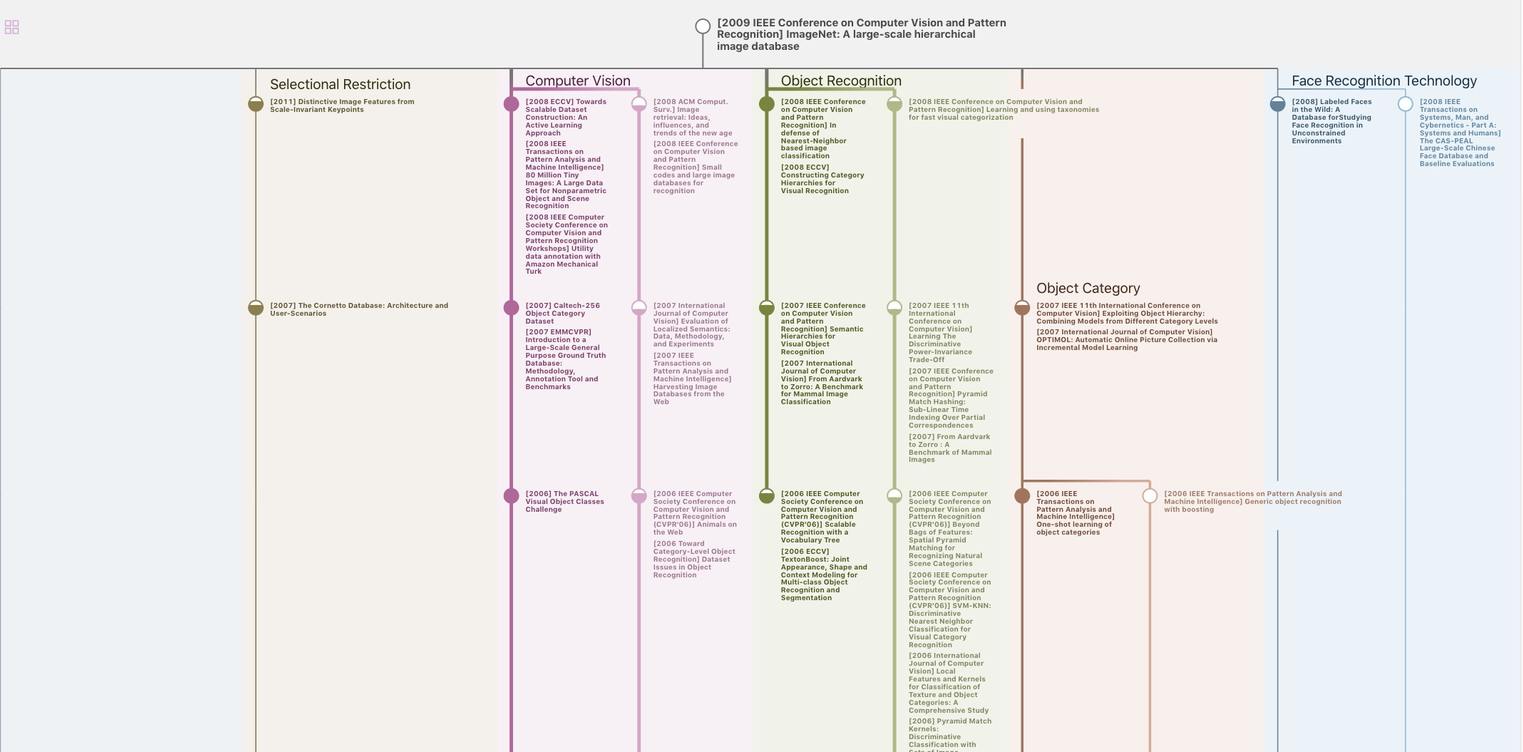
生成溯源树,研究论文发展脉络
Chat Paper
正在生成论文摘要