CARE: Finding Local Linear Correlations in High Dimensional Data.
Cancun(2008)
摘要
Finding latent patterns in high dimensional data is an important research problem with numerous applications. Existing approaches can be summarized into 3 categories: feature selection, feature transformation (or feature projection) and projected clustering. Being widely used in many applications, these methods aim to capture global patterns and are typically performed in the full feature space. In many emerging biomedical applications, however, scientists are interested in the local latent patterns held by feature subsets, which may be invisible via any global transformation. In this paper, we investigate the problem of finding local linear correlations in high dimensional data. Our goal is to find the latent pattern structures that may exist only in some subspaces. We formalize this problem as finding strongly correlated feature subsets which are supported by a large portion of the data points. Due to the combinatorial nature of the problem and lack of monotonicity of the correlation measurement, it is prohibitively expensive to exhaustively explore the whole search space. In our algorithm, CARE, we utilize spectrum properties and effective heuristic to prune the search space. Extensive experimental results show that our approach is effective in finding local linear correlations that may not be identified by existing methods.
更多查看译文
关键词
important research problem,feature subsets,local linear correlation,finding local linear correlations,data point,feature projection,high dimensional data,feature selection,feature transformation,correlated feature subsets,full feature space,application software,biomedical research,clustering algorithms,feature space,vectors,spectrum,data mining,covariance matrix,principal component analysis,data analysis,computer science,linear discriminant analysis,gene expression,search space,correlation,bioinformatics
AI 理解论文
溯源树
样例
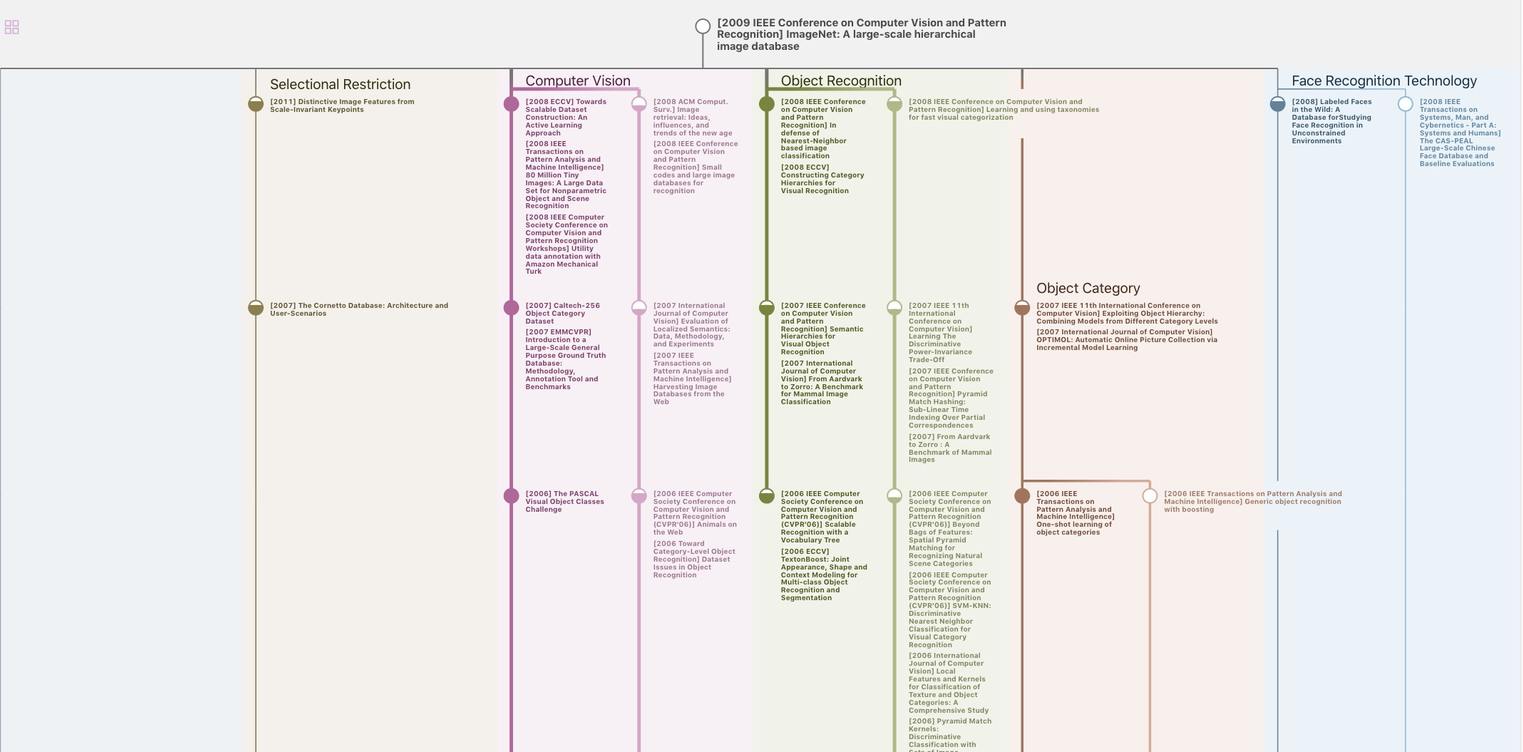
生成溯源树,研究论文发展脉络
Chat Paper
正在生成论文摘要