Joint Uncertainty Decoding with the Second Order Approximation for Noise Robust Speech Recognition
2009 IEEE International Conference on Acoustics, Speech and Signal Processing(2009)
摘要
Joint uncertainty decoding has recently achieved promising results by integrating the front-end uncertainty into the back-end in a mathematically consistent framework. In this paper, joint uncertainty decoding is compared with the widely used vector Taylor series (VTS). We show that the two methods are identical except that joint uncertainty decoding applies the Taylor expansion on each regression class whereas VTS applies it to each HMM mixture. The relatively rougher expansion points used in joint uncertainty decoding make it computationally cheaper than VTS but inevitably worse on recognition accuracy. To overcome this drawback, this paper proposes an improved joint uncertainty decoding algorithm which employs second-order Taylor expansion on each regression class in order to reduce the expansion errors. Special considerations are further given to limit the overall computational cost by adopting different number of regression classes for different orders in the Taylor expansion. Experiments on the Aurora 2 database show that the proposed method is able to beat VTS on recognition accuracy and computational cost with relative improvement up to 6% and 60%, respectively.
更多查看译文
关键词
speech recognition,noise robustness,VTS,uncertainty decoding
AI 理解论文
溯源树
样例
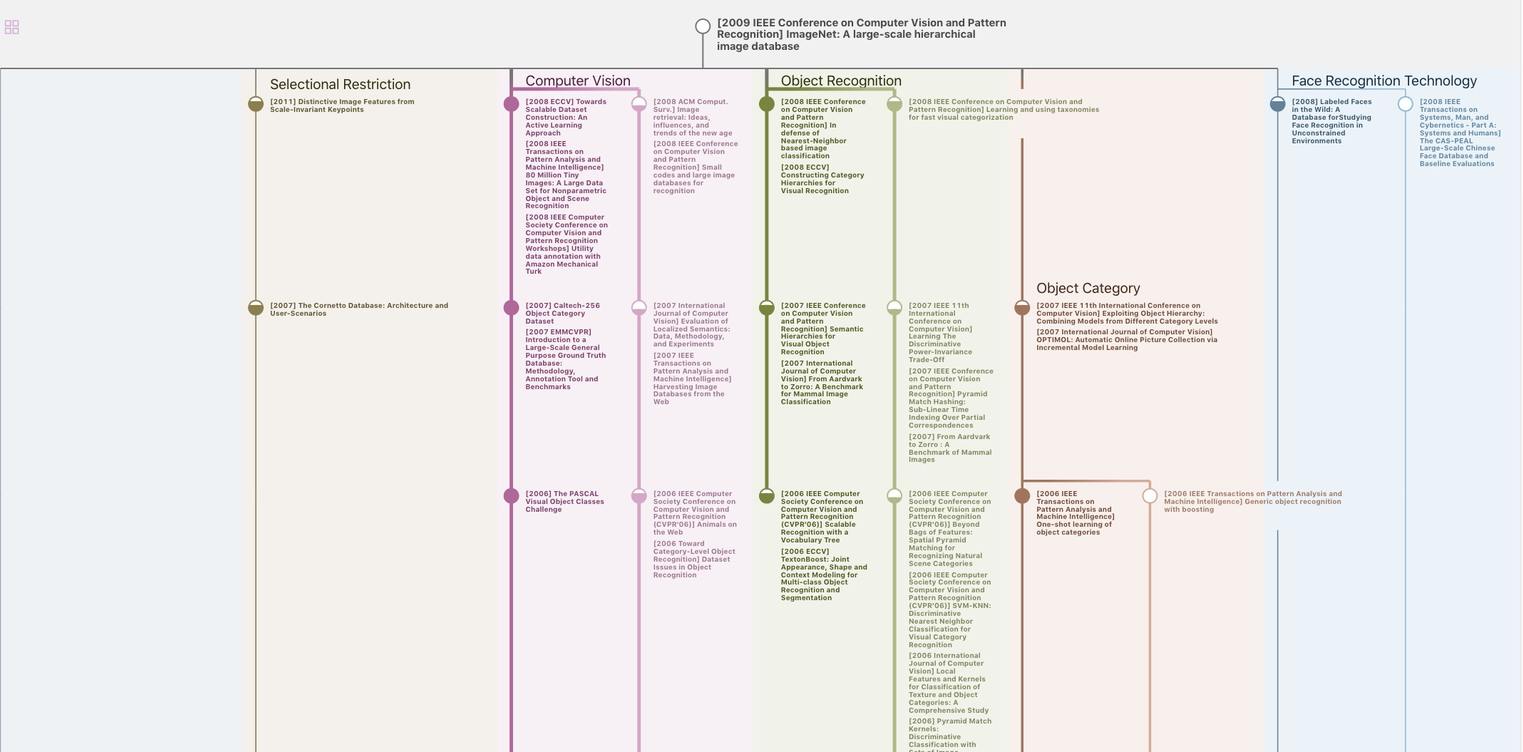
生成溯源树,研究论文发展脉络
Chat Paper
正在生成论文摘要