Preserving Privacy in Time Series Data Classification by Discretization
MACHINE LEARNING AND DATA MINING IN PATTERN RECOGNITION(2009)
摘要
In this paper, we propose discretization-based schemes to preserve privacy in time series data mining. Traditional research on preserving privacy in data mining focuses on time-invariant privacy issues. With the emergence of time series data mining, traditional snapshot-based privacy issues need to be extended to be multi-dimensional with the addition of time dimension . In this paper, we defined three threat models based on trust relationship between the data miner and data providers. We propose three different schemes for these three threat models. The proposed schemes are extensively evaluated against public-available time series data sets [1]. Our experiments show that proposed schemes can preserve privacy with cost of reduction in mining accuracy. For most data sets, proposed schemes can achieve low privacy leakage with slight reduction in classification accuracy. We also studied effect of parameters of proposed schemes in this paper.
更多查看译文
关键词
time series data mining,data mining,time-invariant privacy issue,data provider,preserving privacy,low privacy leakage,public-available time series data,data miner,time series data classification,proposed scheme,threat model,time series data
AI 理解论文
溯源树
样例
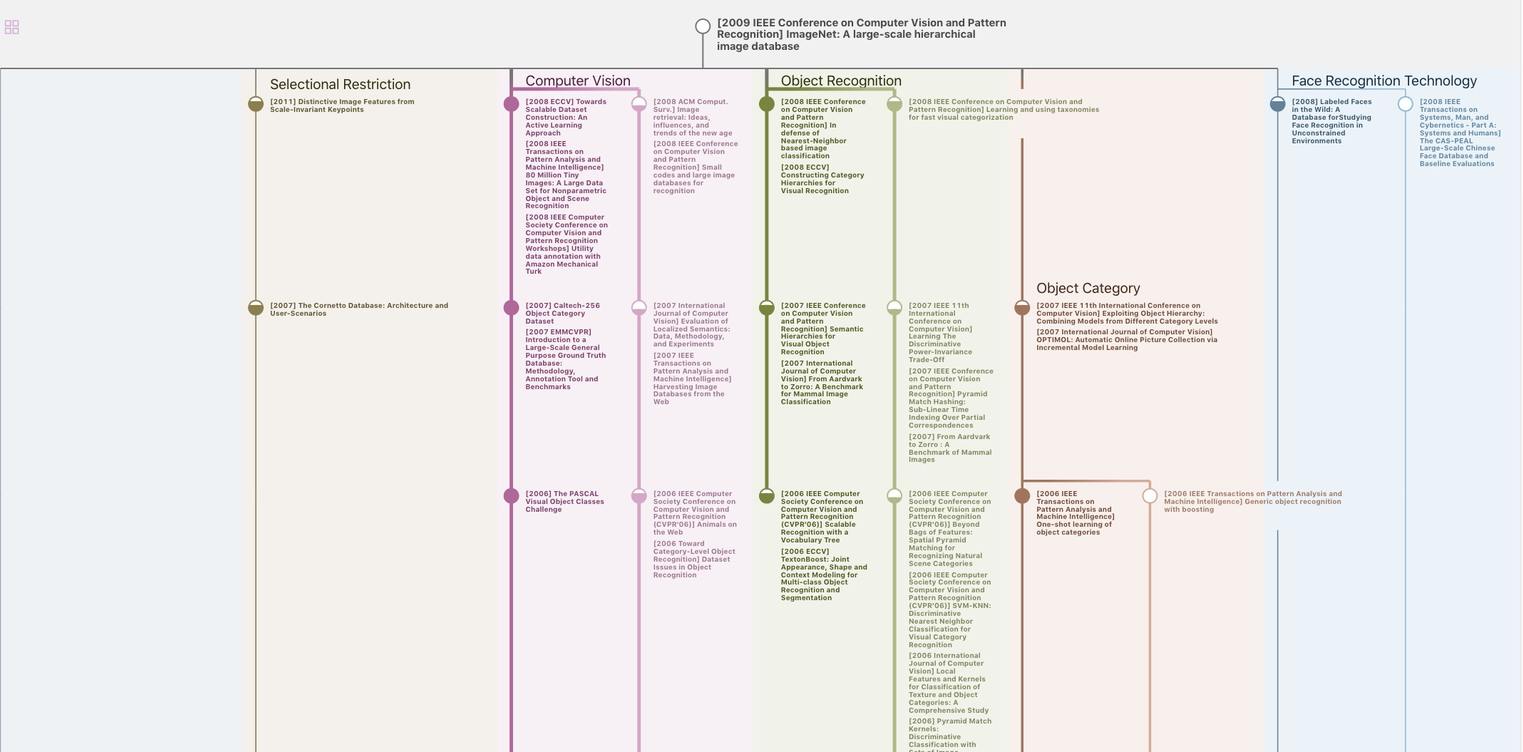
生成溯源树,研究论文发展脉络
Chat Paper
正在生成论文摘要