Semi-supervised Text Classification Using RBF Networks
ADVANCES IN INTELLIGENT DATA ANALYSIS VIII, PROCEEDINGS(2009)
摘要
Semi-supervised text classification has numerous applications and is particularly applicable to the problems where large quantities of unlabeled data are readily available while only a small number of labeled training samples are accessible. The paper proposes a semi-supervised classifier that integrates a clustering based Expectation Maximization (EM) algorithm into radial basis function (RBF) neural networks and can learn for classification from a very small number of labeled training samples and a large pool of unlabeled data effectively. A generalized centroid clustering algorithm is also investigated in this work to balance predictive values between labeled and unlabeled training data and to improve classification accuracy. Experimental results with three popular text classification corpora show that the proper use of additional unlabeled data in this semi-supervised approach can reduce classification errors by up to 26%.
更多查看译文
关键词
rbf networks,unlabeled training data,popular text classification corpus,classification accuracy,classification error,large pool,semi-supervised text classification,additional unlabeled data,small number,unlabeled data,training sample,expectation maximization,em algorithm,radial basis function
AI 理解论文
溯源树
样例
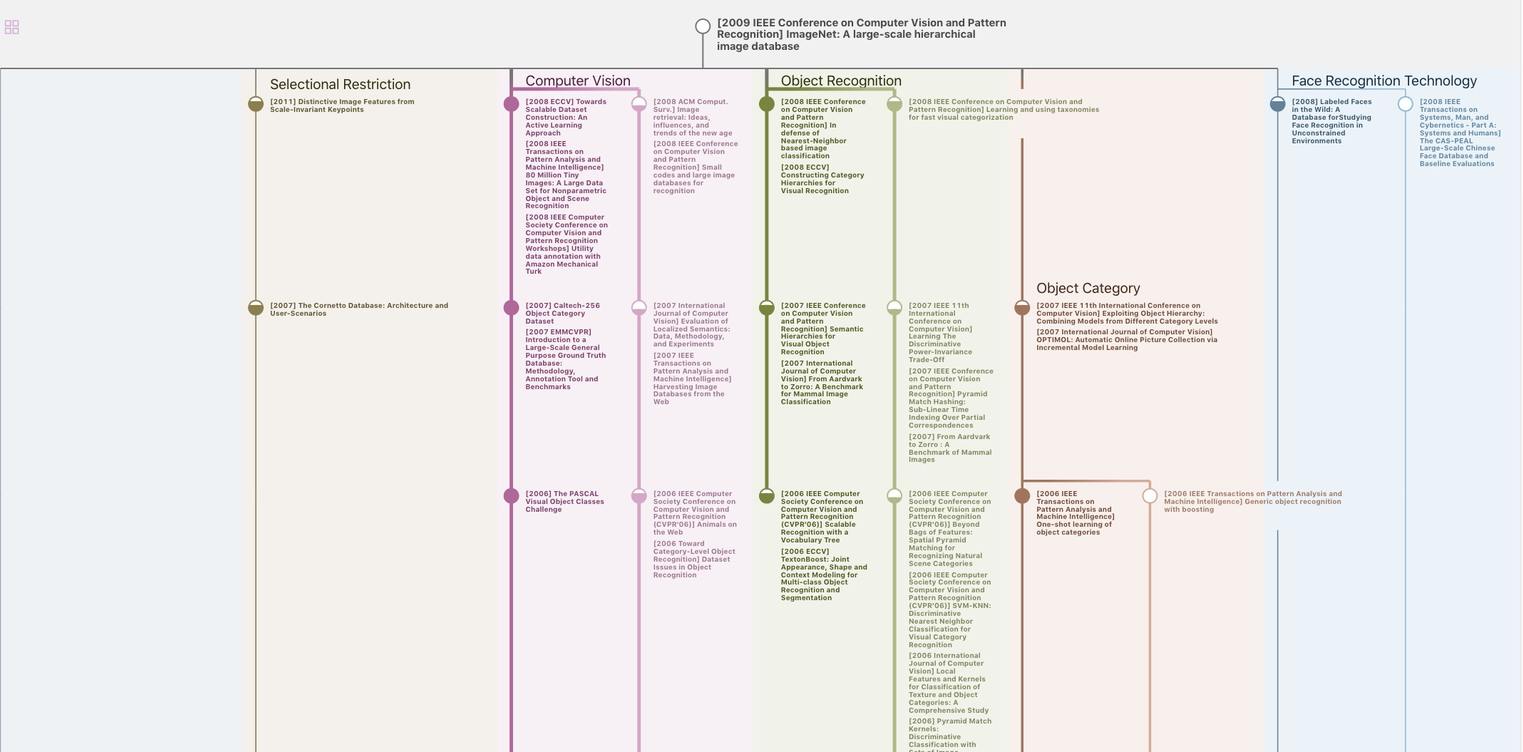
生成溯源树,研究论文发展脉络
Chat Paper
正在生成论文摘要