Latent Dirichlet Bayesian Co-Clustering
MACHINE LEARNING AND KNOWLEDGE DISCOVERY IN DATABASES, PT II(2009)
摘要
Co-clustering has emerged as an important. technique for mining contingency data matrices. However, almost all existing co-clustering algorithms are hard partitioning, assigning each row and column of the data matrix to one cluster. Recently a Bayesian co-clustering approach has been proposed which allows a probability distribution membership in row and column clusters. The approach uses variational inference for parameter estimation. In this work. we modify the Bayesian co-clustering model, and rise collapsed Gibbs sampling and collapsed variational inference for parameter estimation. Our empirical evaluation on real data sets shows that both collapsed Gibbs sampling and collapsed variational inference are able to find more accurate likelihood estimates than the standard variational Bayesian co-clustering approach.
更多查看译文
关键词
Co-Clustering,Graph Learning,Dirichlet Distribution
AI 理解论文
溯源树
样例
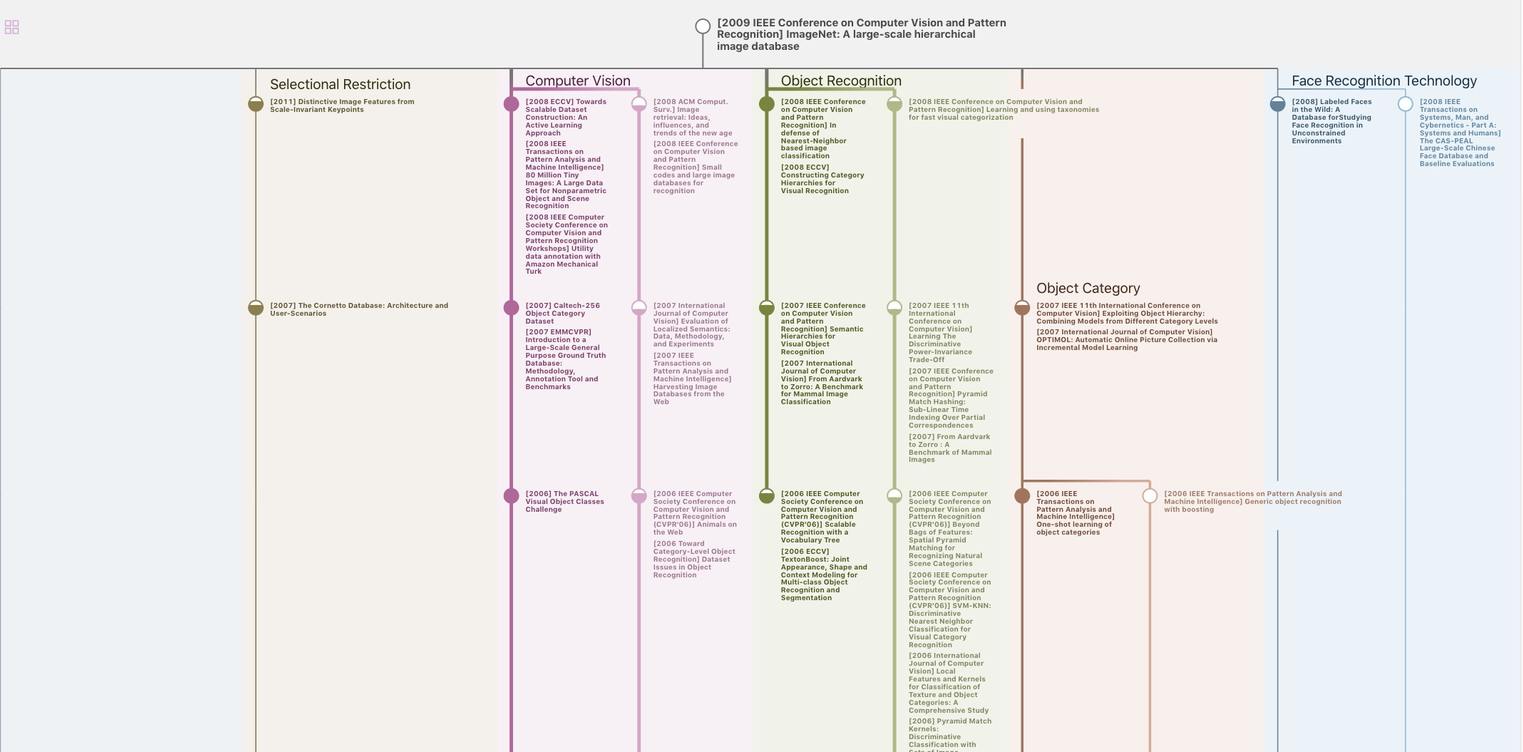
生成溯源树,研究论文发展脉络
Chat Paper
正在生成论文摘要