Time series prediction using RBF neural networks with a nonlinear time-varying evolution PSO algorithm
Neurocomputing(2009)
摘要
The time series prediction of a practical power system is investigated in this paper. The radial basis function neural network (RBFNN) with a nonlinear time-varying evolution particle swarm optimization (NTVE-PSO) algorithm is developed. When training RBFNNs, the NTVE-PSO method is adopted to determine the optimal structure of the RBFNN to predict time series, in which the NTVE-PSO algorithm is a dynamically adaptive optimization approach using the nonlinear time-varying evolutionary functions for adjusting inertia and acceleration coefficients. The proposed PSO method will expedite convergence toward the global optimum during the iterations. To compare the performance of the proposed NTVE-PSO method with existing PSO methods, the different practical load types of Taiwan power system (Taipower) are utilized for time series prediction of one-day ahead and five-days ahead. Simulation results illustrate that the proposed NTVE-PSO-RBFNN has better forecasting accuracy and computational efficiency for different electricity demands than the other PSO-RBFNNs.
更多查看译文
关键词
time series,nonlinear time-varying evolution pso,proposed ntve-pso-rbfnn,nonlinear time-varying evolution,proposed ntve-pso method,taiwan power system,particle swarm optimization,time series prediction,rbf neural network,proposed pso method,ntve-pso method,radial basis function networks,pso method,different electricity demand,ntve-pso algorithm,radial basis function network,power system
AI 理解论文
溯源树
样例
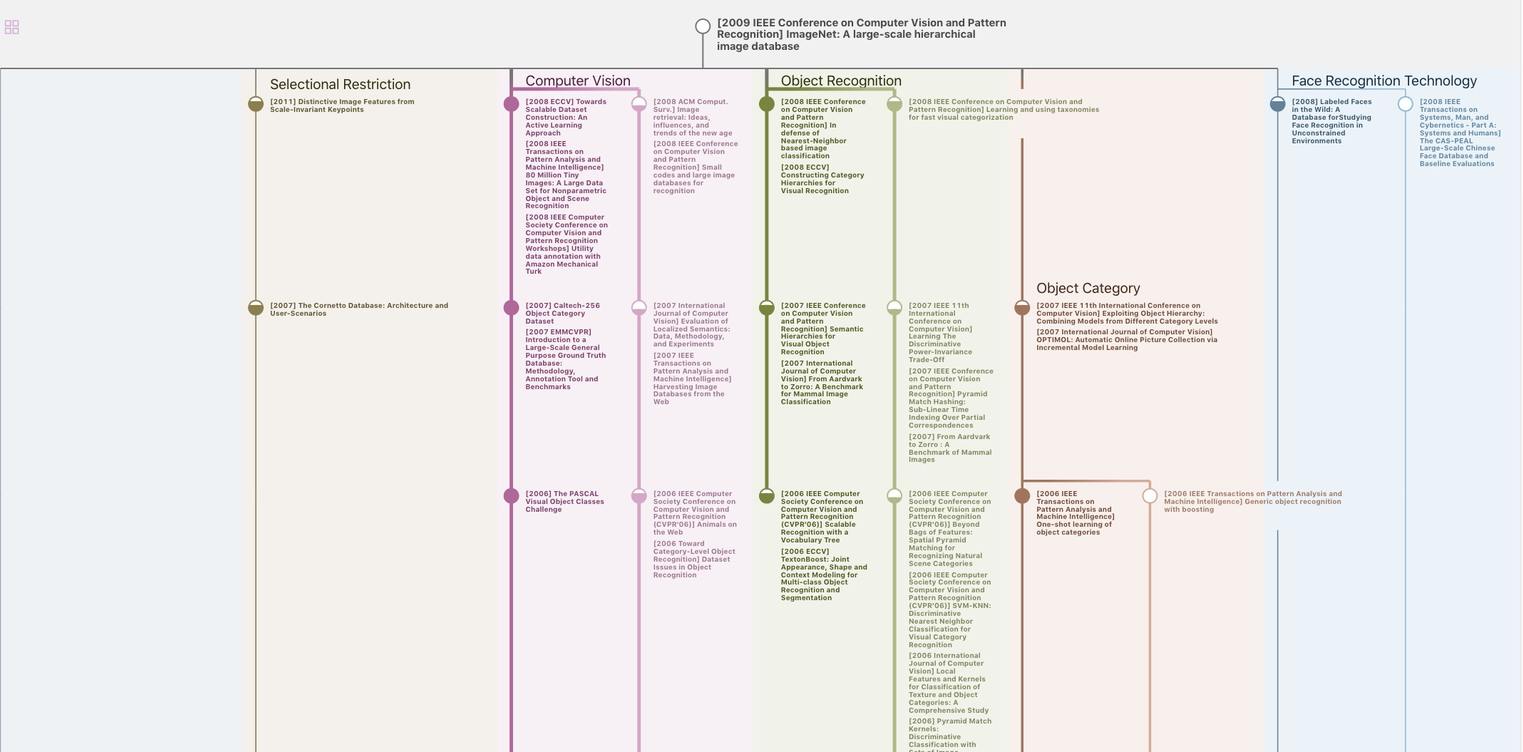
生成溯源树,研究论文发展脉络
Chat Paper
正在生成论文摘要