Improved Learning of Bayesian Networks in Biomedicine
Pisa(2009)
摘要
Bayesian Networks represent one of the most successful tools for medical diagnosis and therapies follow-up. We present an algorithm for Bayesian network structure learning, that is a variation of the standard search-and-score approach. The proposed approach overcomes the creation of redundant network structures that may include non significant connections between variables. In particular, the algorithm finds which relationships between the variables must be prevented, by exploiting the binarization of a square matrix containing the mutual information (MI) among all pairs of variables. Four different binarization methods are implemented. The MI binary matrix is exploited as a preconditioning step for the subsequent greedy search procedure that optimizes the network score, reducing the number of possible search paths in the greedy search. Our approach has been tested on two different medical datasets and compared against the standard search-and-score algorithm as implemented in the DEAL package.
更多查看译文
关键词
algorithm find,redundant network structure,bayesian networks,greedy search,standard search-and-score algorithm,standard search-and-score approach,possible search path,improved learning,subsequent greedy search procedure,bayesian network structure learning,network score,biomedicine,learning artificial intelligence,bayesian network,finite element methods,binary matrix,data mining,bayesian methods,medical diagnosis,mutual information,biomedical engineering,cancer,square matrix,greedy algorithms,symmetric matrices
AI 理解论文
溯源树
样例
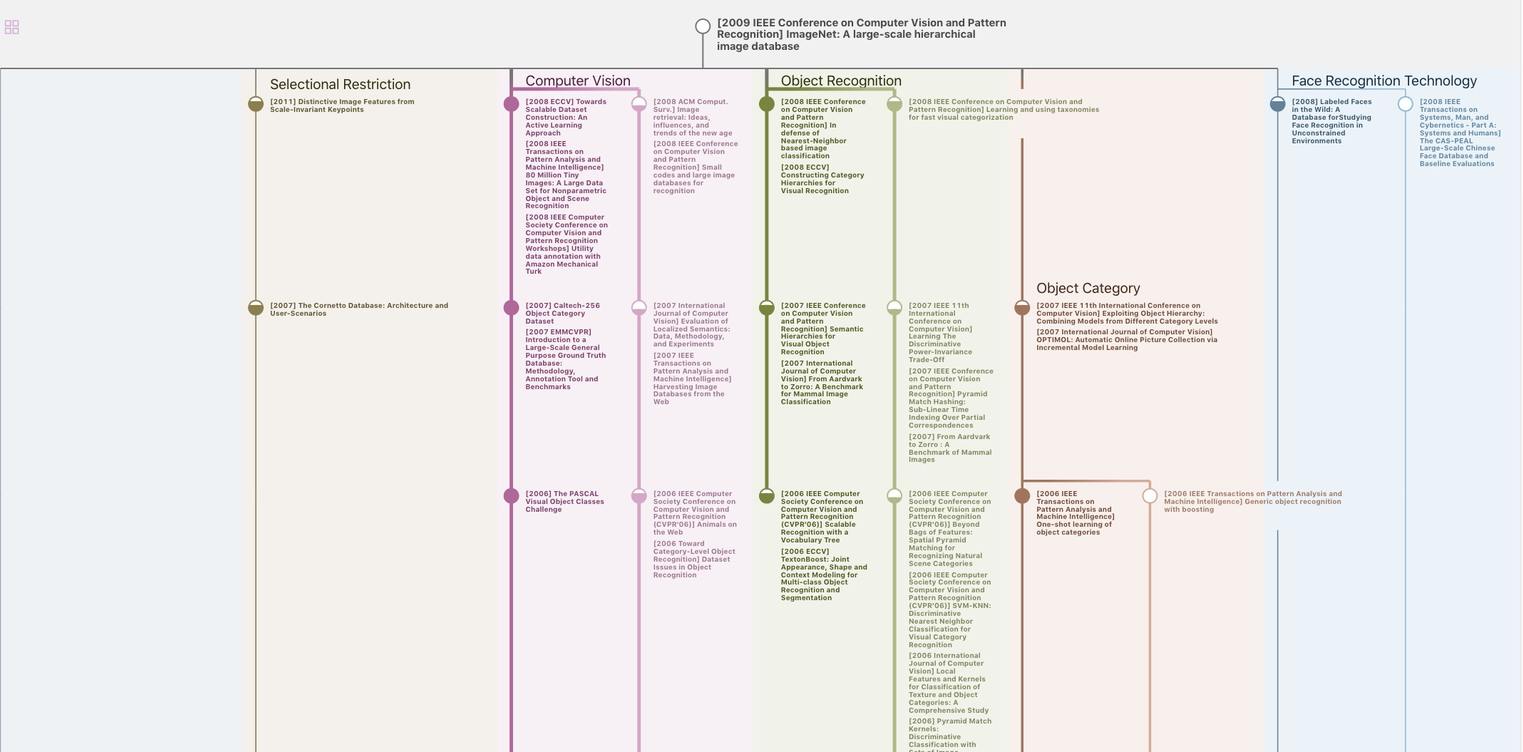
生成溯源树,研究论文发展脉络
Chat Paper
正在生成论文摘要