Non-exhaustive Learning for Bacteria Detection
Indianapolis, IN(2009)
摘要
Technologies for rapid detection and classification of bacterial pathogens are crucial for securing the food supply. A light-scattering sensor recently developed for real-time detection and identification of colonies of multiple pathogens has shown great promise for distinguishing bacteria cultures at the genus and species level for Listeria, Staphylococcus, Salmonella, Vibrio, and Escherichia. Unlike traditional testing methods, this new technology does not require a labeling reagent or biochemical processing. The classification approach currently used with this technology relies on supervised learning. For an accurate detection and classification of bacterial pathogens, the training library used to train the classifier should consist of samples of all possible forms of the pathogens. Construction of such a training library is impractical if not impossible due to the high mutation rate that characterizes some of the infectious agents. In this study we propose a Bayesian approach to advance this sensor technology to allow for the detection of new classes/subclasses of bacteria, which do not exist in the training library. Learning with a nonexhaustive training library is an ill-defined problem. We assume Gaussian distributions for bacteria subclasses and implement a maximum likelihood classifier. A pair of conjugate priors based on Wishart distribution is defined and the covariance matrices are estimated by the posterior mean. A new sample is classified into one of the existing set of classes if the maximum of the likelihoods is above a designated threshold. If not, the sample is considered a novelty, i.e. a sample of a potentially new class. We compare the proposed approach with a benchmark support estimation technique as well as a simulated Bayesian modelling approach recently proposed.
更多查看译文
关键词
training library,bayesian approach,new sample,new technology,bacterial pathogen,bacteria detection,new class,accurate detection,nonexhaustive training library,non-exhaustive learning,classification approach,wishart distribution,data mining,maximum likelihood estimation,test methods,real time,covariance matrix,gaussian distribution,silicon,microorganisms,mutation rate,light scattering,biophysics,bayesian methods,conjugate prior,learning artificial intelligence,supervised learning
AI 理解论文
溯源树
样例
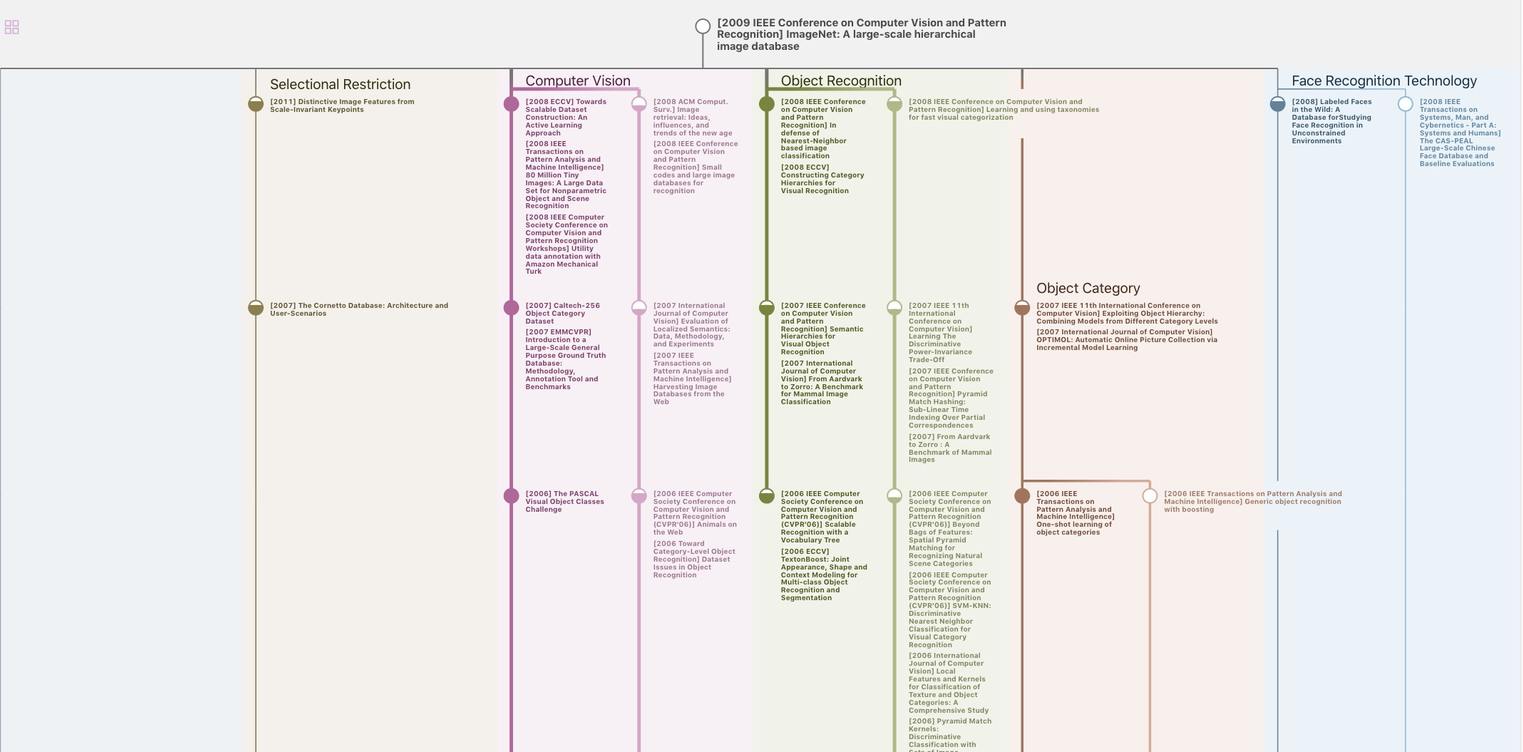
生成溯源树,研究论文发展脉络
Chat Paper
正在生成论文摘要