Ensembling Heterogeneous Learning Models with Boosting
NEURAL INFORMATION PROCESSING, PT 1, PROCEEDINGS(2009)
摘要
In this paper, we investigate the potentials of a novel classifier ensemble scheme, referred to as heterogeneous boosting (HB), which aims at delivering higher levels of diversity by allowing that distinct learning algorithms be recruited to induce the different components of the boosting sequence. For the automatic design of the HB structures in accord with the nuances of the problem at hand, a genetic algorithm engine is adopted to work jointly with AdaBoost, the state-of-the-art boosting algorithm. To validate the novel approach, experiments involving well-known learning algorithms and classification datasets from the UCI repository are discussed. The accuracy, generalization, and diversity levels incurred with HB are matched against those delivered by AdaBoost working solely with RBF neural networks, with the first either significantly prevailing over or going in par with the latter in all the cases.
更多查看译文
关键词
boosting,heterogeneous models,genetic algorithms.
AI 理解论文
溯源树
样例
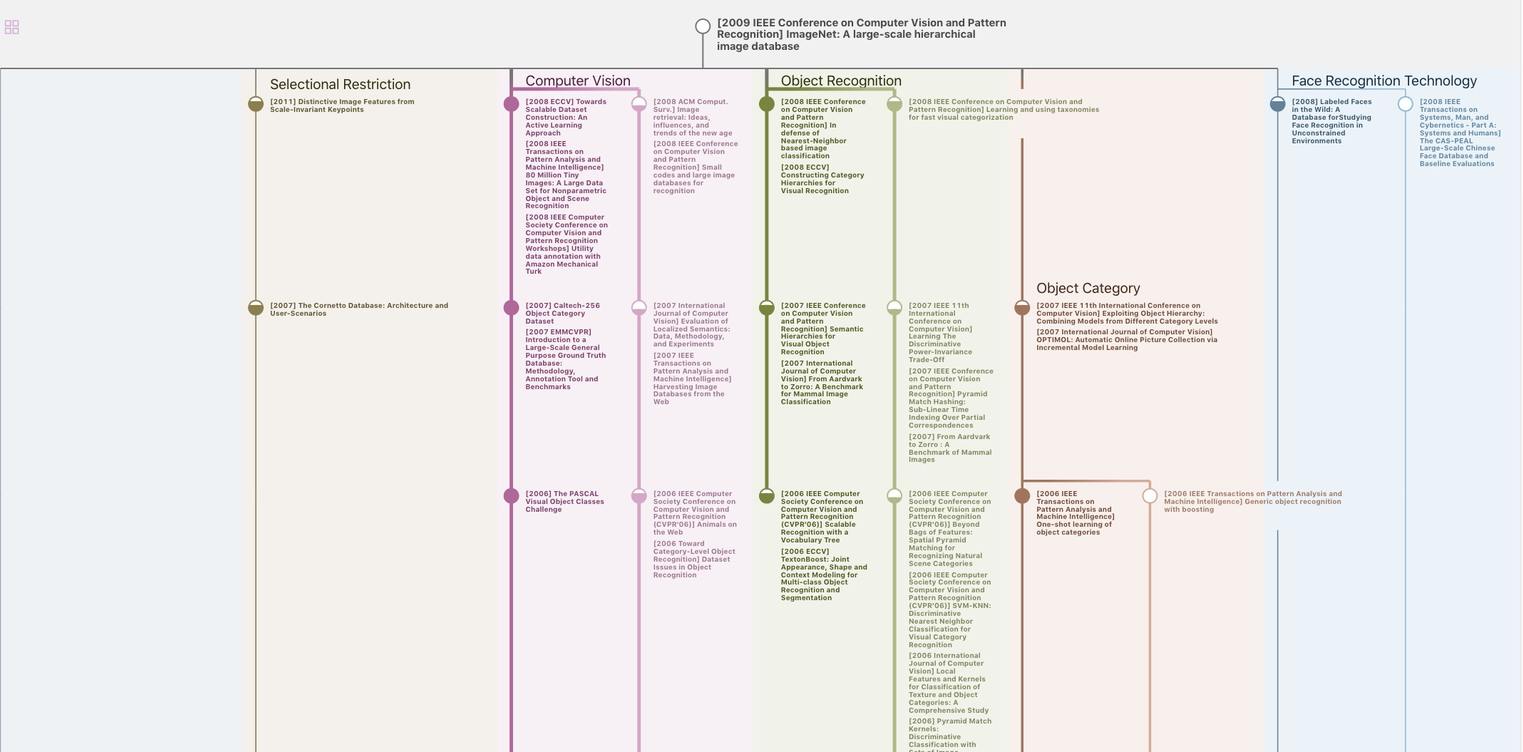
生成溯源树,研究论文发展脉络
Chat Paper
正在生成论文摘要