Distortion minimization in Gaussian layered broadcast coding with successive refinement
IEEE Transactions on Information Theory(2009)
摘要
A transmitter without channel state information wishes to send a delay-limited Gaussian source over a slowly fading channel. The source is coded in superimposed layers, with each layer successively refining the description in the previous one. The receiver decodes the layers that are supported by the channel realization and reconstructs the source up to a distortion. The expected distortion is minimized by optimally allocating the transmit power among the source layers. For two source layers, the allocation is optimal when power is first assigned to the higher layer up to a power ceiling that depends only on the channel fading distribution; all remaining power, if any, is allocated to the lower layer. For convex distortion cost functions with convex constraints, the minimization is formulated as a convex optimization problem. In the limit of a continuum of infinite layers, the minimum expected distortion is given by the solution to a set of linear differential equations in terms of the density of the fading distribution. As the number of channel uses per source symbol tends to zero, the power distribution that minimizes expected distortion converges to the one that maximizes expected capacity.
更多查看译文
关键词
distortion minimization,minimum expected distortion,source symbol,source layer,channel state information,expected distortion,distortion converges,convex distortion cost function,delay-limited gaussian source,fading channel,channel realization,successive refinement,linear differential equation,information theory,broadcasting,channel coding,linear differential equations,broadcast,superposition,convex optimization,distortion,cost function,source coding
AI 理解论文
溯源树
样例
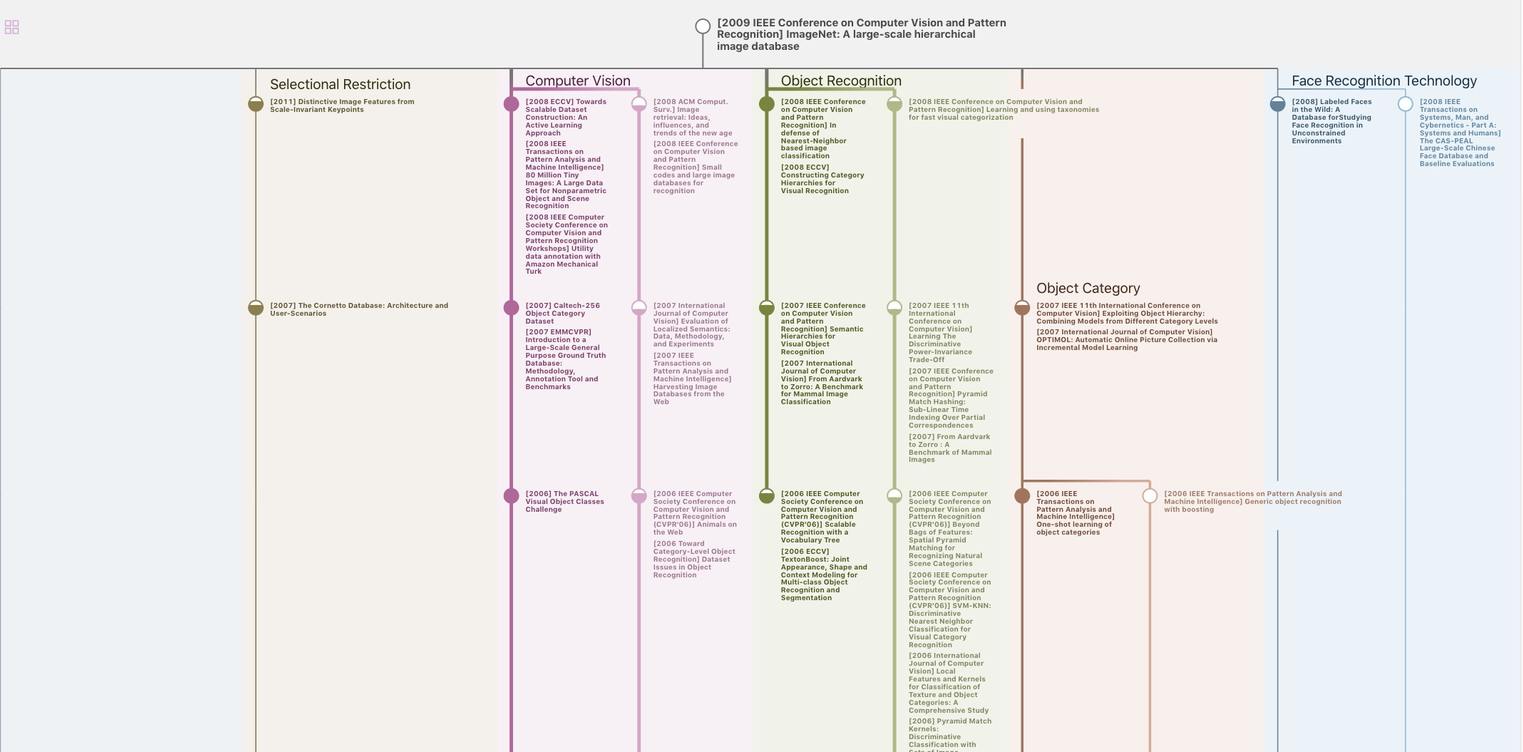
生成溯源树,研究论文发展脉络
Chat Paper
正在生成论文摘要