Robust Stability of Discrete-Time Stochastic Neural Networks with Markovian Jumping Parameters and Mode-Dependent Delays
Innovative Computing, Information and Control(2009)
摘要
This paper investigates the problem of robust stability for a class of uncertain discrete-time stochastic recurrent neural networks (RNNs) with Markovian jumping parameters and mode-dependent delays. Stochastic item is nonlinear, and jumping parameters are considered as discrete time, discrete-state Markov process. We can get novel robust stability conditions in terms of linear matrix inequality (LMI) approach. Furthermore, we will introduce into some free weighting matrices by adding to a zero item in order to lead to much less conservative results. At last, a numerical example is given to illustrate the effectiveness of the proposed method.
更多查看译文
关键词
uncertain systems,discrete time,conservative result,robust stability,stochastic systems,uncertain discrete-time stochastic recurrent,novel robust stability condition,linear matrix inequality,zero item,markovian jumping parameters,delays,stochastic item,discrete-time stochastic neural networks,free weighting matrix,discrete time systems,linear matrix inequalities,uncertain discrete-time stochastic recurrent neural networks,recurrent neural nets,free weighting matrices,stability,markov processes,discrete-state markov process,mode-dependent delays,recurrent neural networks,symmetric matrices,artificial neural networks,stability analysis,markov process,recurrent neural network,neural network
AI 理解论文
溯源树
样例
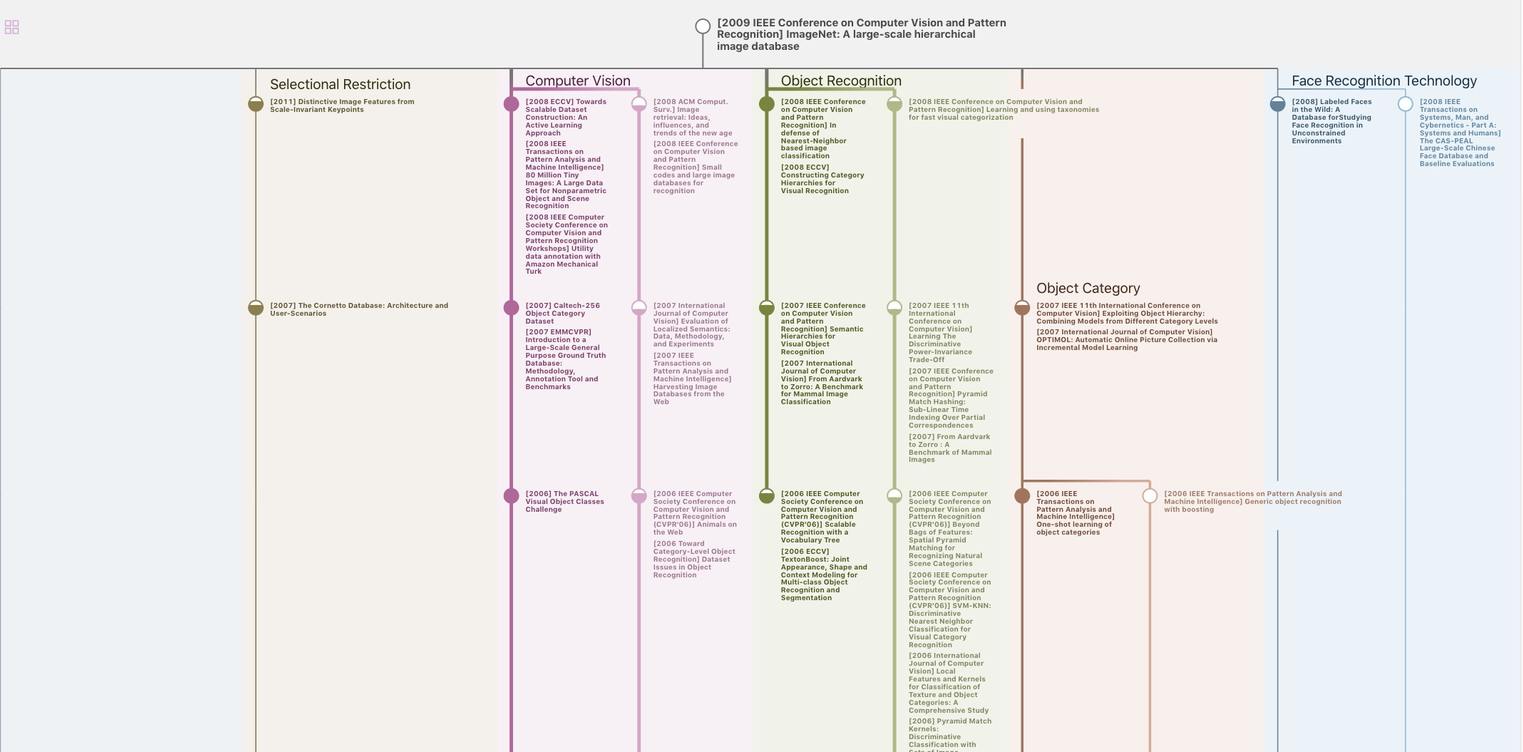
生成溯源树,研究论文发展脉络
Chat Paper
正在生成论文摘要