A Hybrid Support Vector Regression for Time Series Prediction
Phuket(2010)
摘要
Due to time series forecasting involves a rather complex data pattern, there are lots of novel forecasting approaches to improve the forecasting accuracy. Unlike most conventional neural network models, which are based on the empirical risk minimization principle, SVM applies the structural risk minimization principle to minimize an upper bound of the generalization error, rather than minimizing the training error. It has been successfully used to solve nonlinear regression and times series problems. One particular model can not capture all data patterns easily. This article presents a hybrid SVM model with mixed kernel function to exploit the unique strength of the linear and nonlinear SVM models to deal with this problem. Furthermore, parameters of both the linear and nonlinear SVM models are determined by Immune Algorithm (IA). A numerical example is employed to compare the performance of the proposed model. Experiment results reveal that the proposed model promising alternative for forecasting time series problems.
更多查看译文
关键词
particular model,neural network models,forecasting accuracy,mixed kernel function,ia,nonlinear svm model,training error,forecasting theory,hybrid svm model,hybrid support,svr,vector regression,nonlinear regression,regression analysis,time series problem,svm,conventional neural network model,generalization error,time series forecasting,hybrid support vector regression,novel forecasting approach,minimisation,time series prediction,time series,support vector machines,neural nets,empirical risk minimization principle,structural risk minimization,support vector regression,neural network model,upper bound,kernel function,complex data,empirical risk minimization
AI 理解论文
溯源树
样例
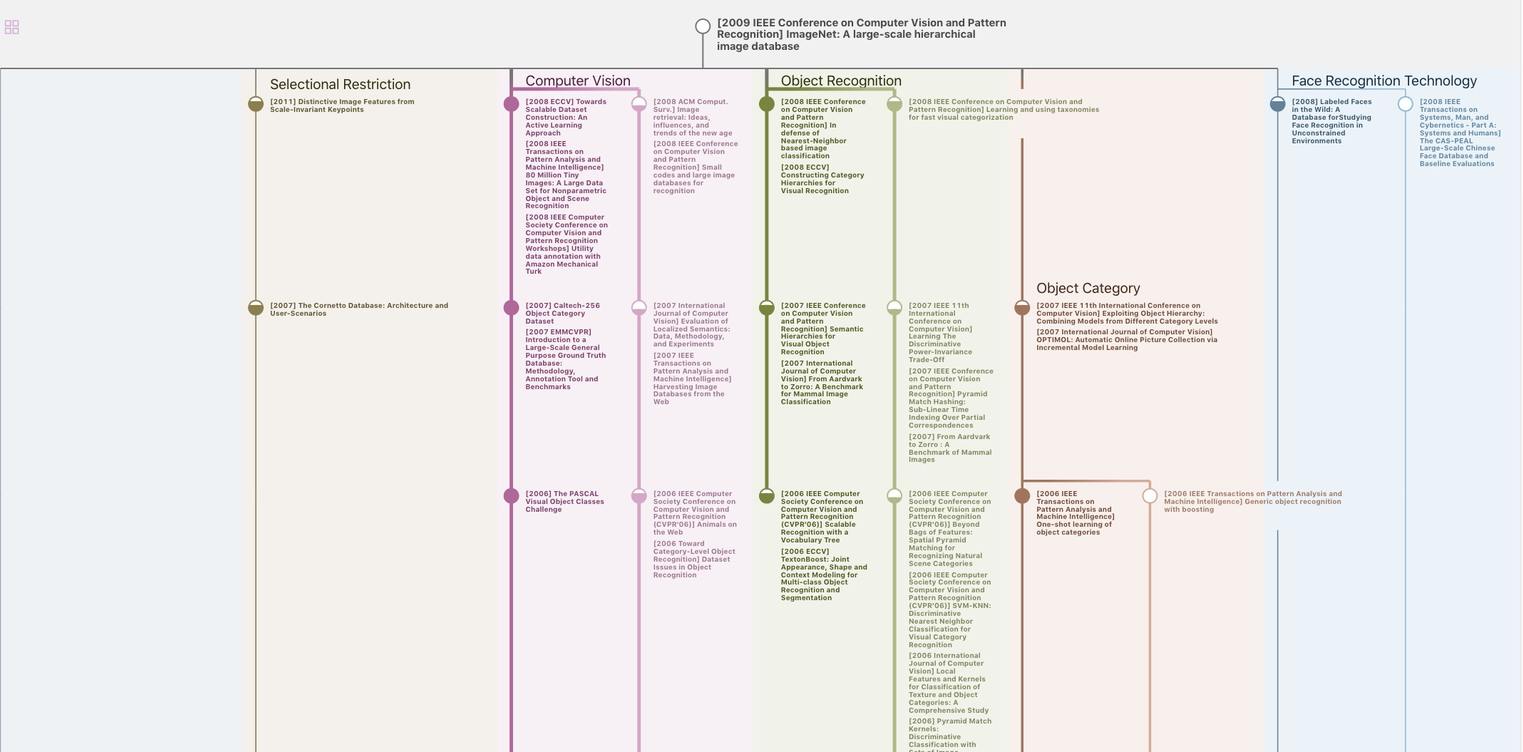
生成溯源树,研究论文发展脉络
Chat Paper
正在生成论文摘要