Predicting mobility events on personal devices.
Pervasive and Mobile Computing(2010)
摘要
High-end mobile phones are quickly becoming versatile sensing platforms, capable of continuously capturing the dynamic context of their owners through various sensors. A change in this context is often caused by the fact that owners-and therefore the devices they carry-are moving from one place to another. In this paper, we model the sensed environment as a stream of events, and assume, given that people are creatures of habit, that time correlations exists between successive events. We propose a method for the prediction in time of the next occurrence of an event of interest, such as 'arriving at a certain location' or 'meeting with another person', with a focus on the prediction of network visibility events as observed through the wireless network interfaces of the device. Our approach is based on using other events in the stream as predictors for the event we are interested in, and, in the case of multiple predictors, applying different strategies for the selection of the best predictor. Using two real-world data sets, we found that including predictors of infrequently occurring events results in better predictions using the best selection strategy. Also, we found that cross-sensor (cross-interface) information in most cases improves the prediction performance.
更多查看译文
关键词
mobile device,time series,network interface,mobility model,synthetic data,kernel density estimate,kernel density estimation,personalization
AI 理解论文
溯源树
样例
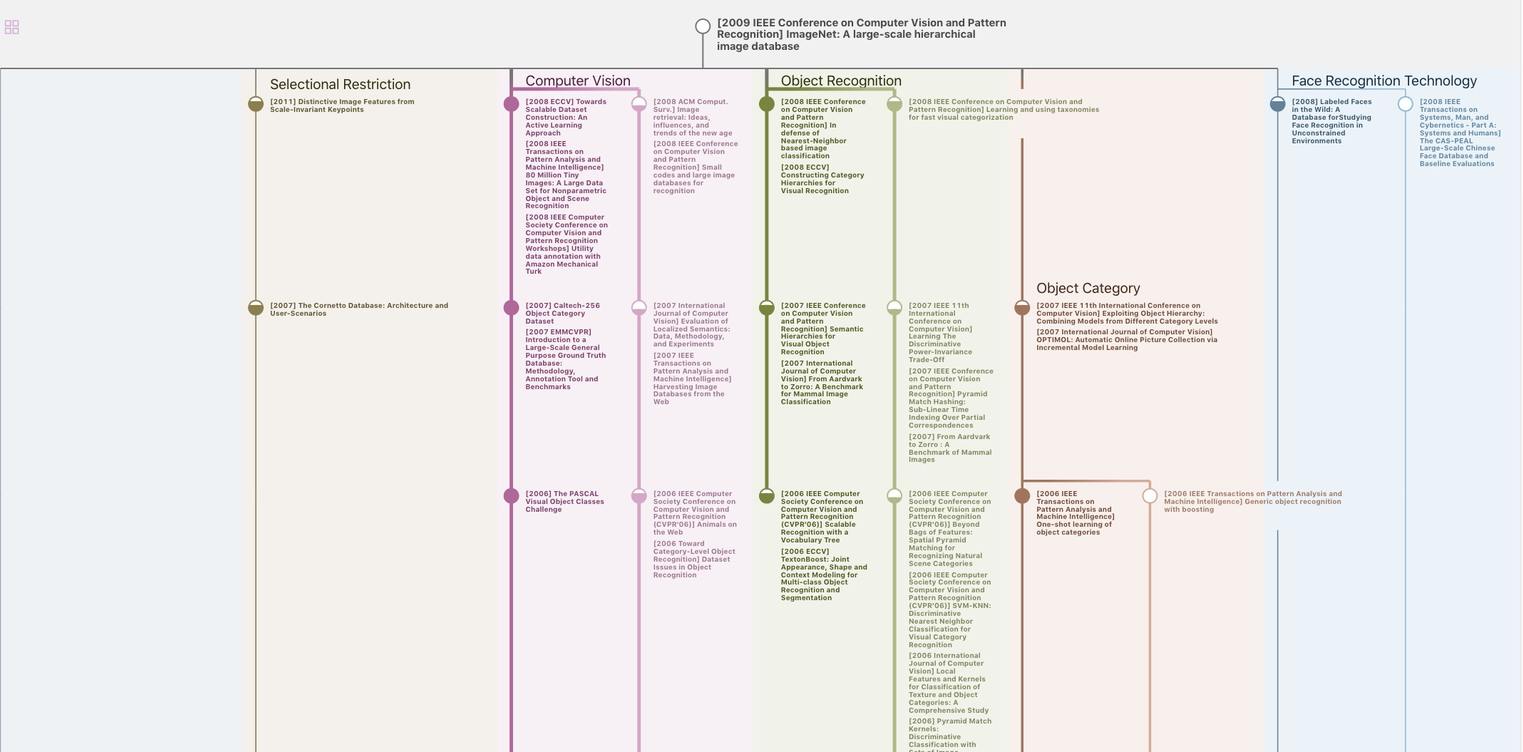
生成溯源树,研究论文发展脉络
Chat Paper
正在生成论文摘要