Sensitive White Space Detection with Spectral Covariance Sensing
IEEE Transactions on Wireless Communications(2010)
摘要
This paper proposes a novel, highly effective spectrum sensing algorithm for cognitive radio and white space applications. The proposed spectral covariance sensing (SCS) algorithm exploits the different statistical correlations of the received signal and noise in the frequency domain. Test statistics are computed from the covariance matrix of a partial spectrogram and compared with a decision threshold to determine whether a primary signal or arbitrary type is present or not. This detector is analyzed theoretically and verified through realistic open-source simulations using actual digital television signals captured in the US. Compared to the state of the art in the literature, SCS improves sensitivity by 3 dB for the same dwell time, which is a very significant improvement for this application. Further, it is shown that SCS is highly robust to noise uncertainty, whereas many other spectrum sensors are not.
更多查看译文
关键词
statistical correlation,received signal,arbitrary type,spectral covariance sensing,effective spectrum,spectral covariance,white space,noise,scs algorithm,partial spectrogram,cognitive radio,frequency-domain analysis,ieee 802.22,covariance matrices,spectral analysis,decision threshold,actual digital television,spectrum sensor,frequency domain,sensitive white space detection,test statistics,digital television signal,proposed spectral covariance,spectrum sensing,noise uncertainty,signal detection,correlation methods,covariance matrix,primary signal,testing,digital television,correlation,detectors,frequency domain analysis,signal to noise ratio,spectrogram,white spaces,dwell time,statistical analysis,spectrum,signal analysis
AI 理解论文
溯源树
样例
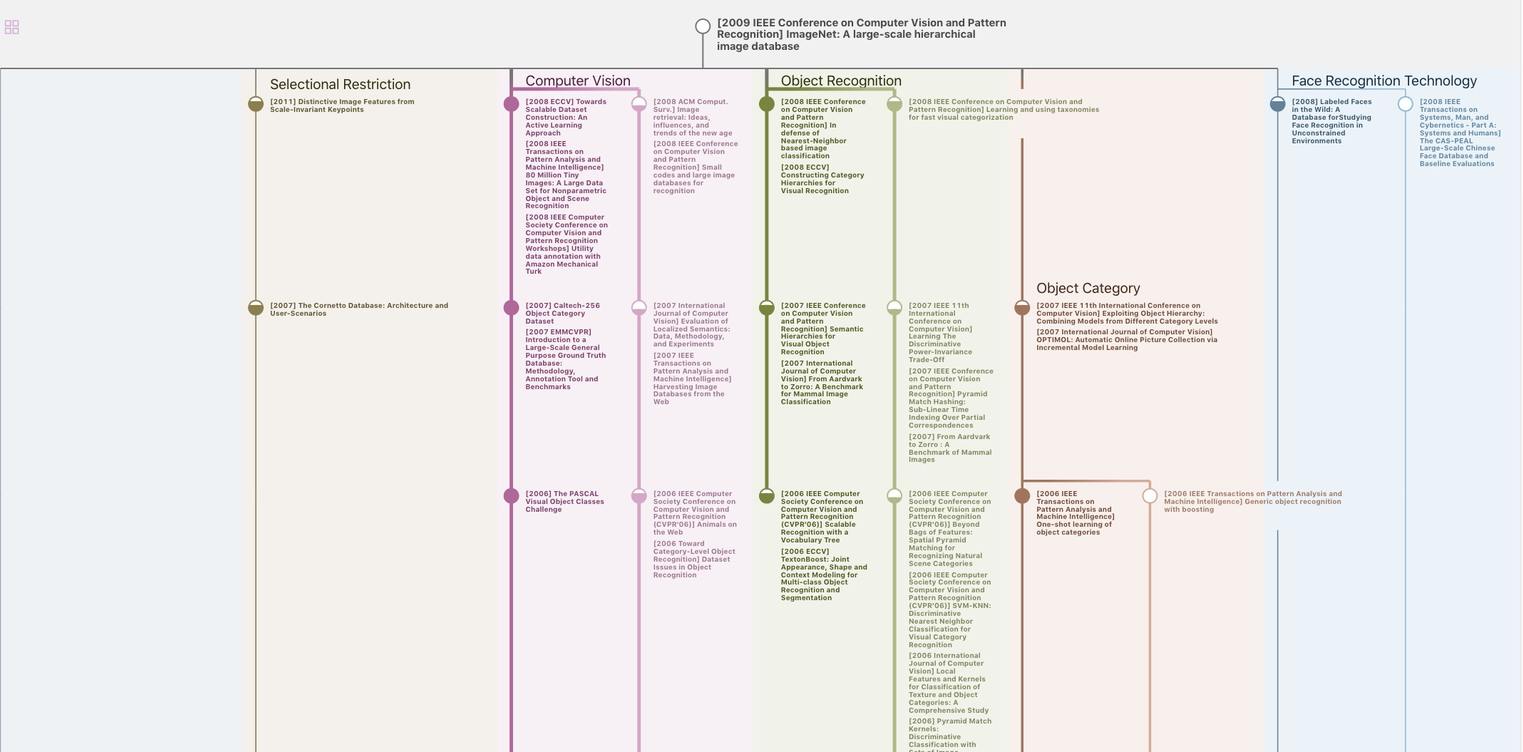
生成溯源树,研究论文发展脉络
Chat Paper
正在生成论文摘要