Rank Internet Service Quality Using Multiple Features: A Machine Learning Approach
Semantics Knowledge and Grid(2010)
摘要
This paper addresses the problem of Ranking Internet service quality by taking a machine learning approach using multiple service features. Ranking helps find good services for applications that use services as building blocks. Unlike other ranking problems, the goodness of Internet service qualities is dependent upon multiple key features. The key features vary across different service categories and have unequally discriminative natures. This paper divides the ranking problem into four subtasks including categorizing services according to the service functionalities, identifying key features that determine the quality, denoising for feature measurement values and computing global ranking scores with multiple key features, which are cast into machine learning problems and solved using techniques classification, feature selection, clustering, and regression respectively. In particular, we propose in this paper an efficient dense-block based denoising method for subjective features, and a Supported Vector Regression based method for computing global ranking scores. Experimental results on both synthetic and real data show that the proposed approach can quantitatively identify the key features across service categories, discard noisy measurement values in 10 times faster, and compute the global ranking scores using multiple features with low mean squared errors for both linear and nonlinear ranking functions.
更多查看译文
关键词
global ranking score,supported vector regression,multiple key feature,multiple key features,noisy measurement values,nonlinear ranking function,classification,internet service quality,web services,categorizing service,dense-block based denoising method,learning (artificial intelligence),ranking internet service quality,ranking problem,different service category,good service,rank internet service quality,global ranking scores,nonlinear ranking functions,feature measurement values,multiple features,machine learning approach,multiple service features,ranking,feature selection,low mean squared errors,machine learning,key feature,internet service,mean square error methods,learning artificial intelligence,computational modeling,clustering algorithms,support vector machines,support vector regression,mean square error,training data
AI 理解论文
溯源树
样例
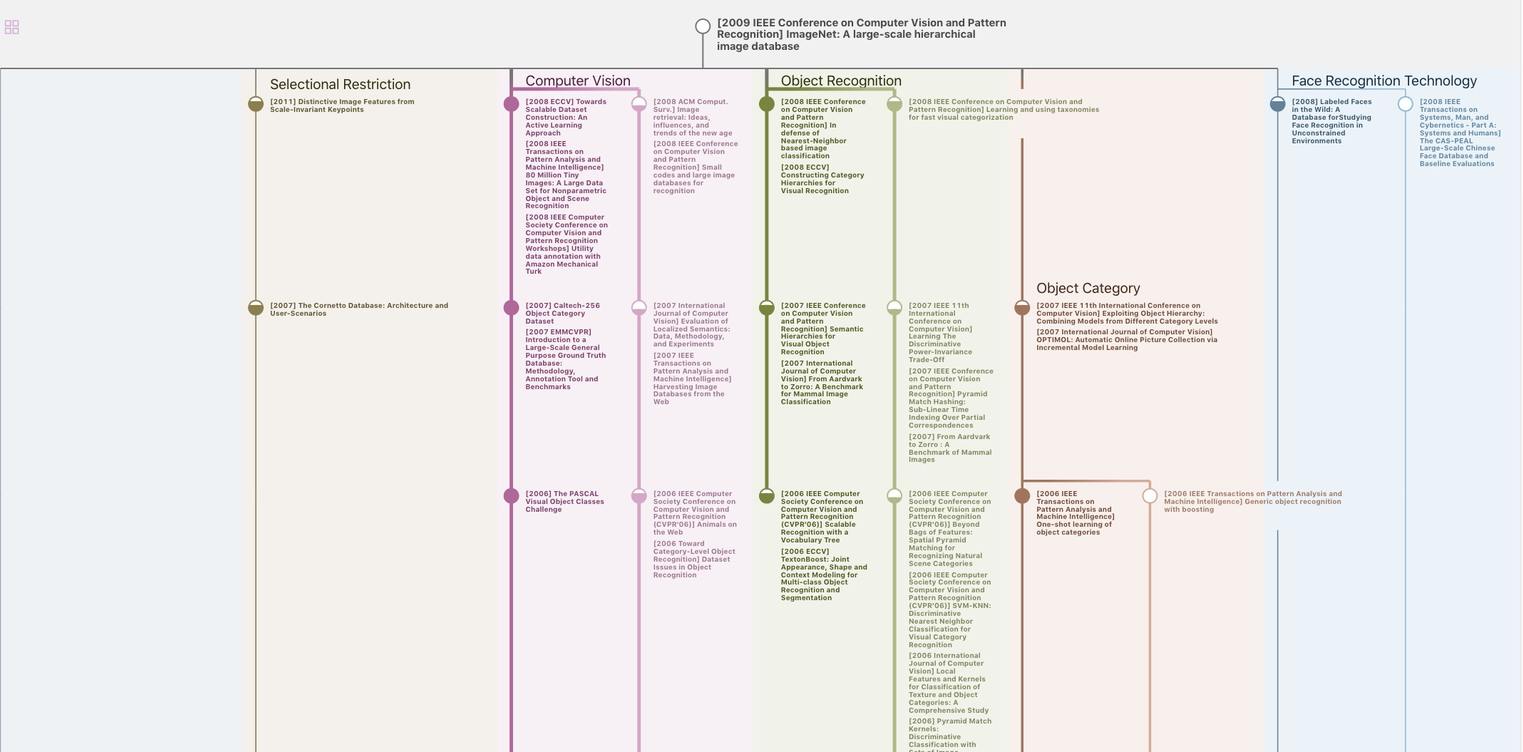
生成溯源树,研究论文发展脉络
Chat Paper
正在生成论文摘要