Predicting Travel Times with Context-Dependent Random Forests by Modeling Local and Aggregate Traffic Flow
Data Mining Workshops(2010)
摘要
Predicting future traffic flow has the potential to decrease travel times. This paper describes a methodology developed to predict future travel times based on GPS reports of 1% of the cars on the road in a simulation framework. Features representing local and aggregate models of traffic flow were extracted from these reports. These included the counts of moving and stopped cars in different areas, along with each road segment’s mean speed. Context-dependent Random Forests were then trained to predict traffic flow six and thirty minutes into the future. This algorithm performed best in one track of the 2010 IEEE ICDM Contest: Tom Tom Traffic Prediction for Intelligent GPS Navigation, improving 9% on the next-best algorithm and 62.5% on the baseline.
更多查看译文
关键词
next-best algorithm,context-dependent random forests,traffic flow,intelligent gps navigation,travel time,predicting travel times,aggregate traffic flow,gps report,future travel time,future traffic flow,road segment,tom tom traffic prediction,prediction algorithms,global positioning system,predictive models,random forest,context dependent,principal component analysis
AI 理解论文
溯源树
样例
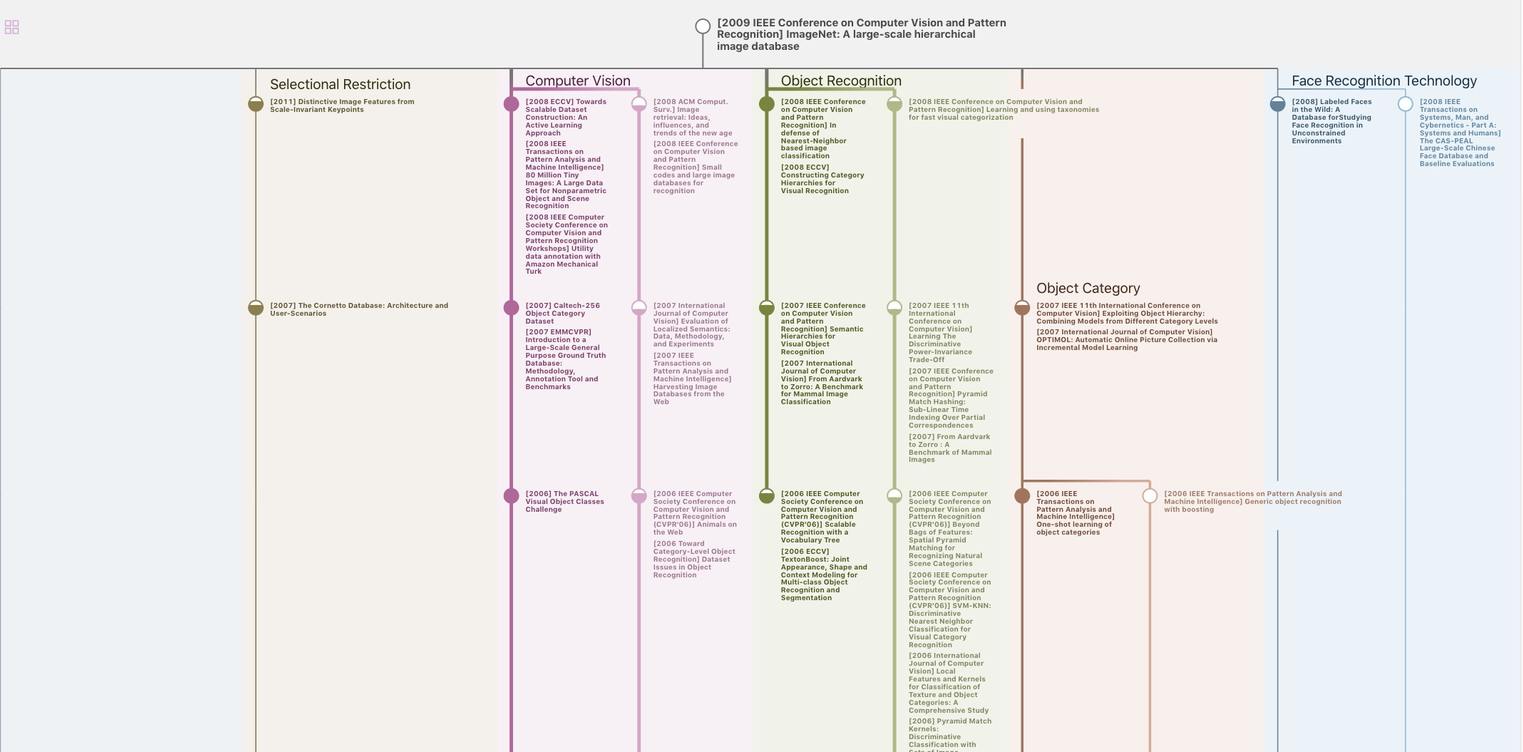
生成溯源树,研究论文发展脉络
Chat Paper
正在生成论文摘要