Dimensionality reduction using a Gaussian Process Annealed Particle Filter for tracking and classification of articulated body motions
Computer Vision and Image Understanding(2011)
摘要
This paper presents a framework for 3D articulated human body tracking and action classification. The method is based on nonlinear dimensionality reduction of high-dimensional data space to low dimensional latent space. Human body motion is described by concatenation of low-dimensional manifolds that characterize different motion types. We introduce a body pose tracker thats uses the learned mapping function from latent space to body pose space. The trajectories in the latent space provide low dimensional representations of body pose sequences representing a specific action type. These trajectories are used to classify human actions. The approach is illustrated on the HumanEvaI and HumanEvaII datasets, as well as on other datasets that include scenarios of interactions between people. A comparison to other methods is presented. The tracker is shown to be robust when classifying individual actions and is also capable of the harder task of classifying interactions between people.
更多查看译文
关键词
classifying individual action,articulated human body tracking,action classification,human body motion,gaussian process annealed particle,articulated body tracking,latent space,human action,articulated body motion,high-dimensional data space,low dimensional latent space,humanevaii datasets,dimensionality reduction,classifying interaction,gaussian process,human body,particle filter,nonlinear dimensionality reduction,high dimensional data
AI 理解论文
溯源树
样例
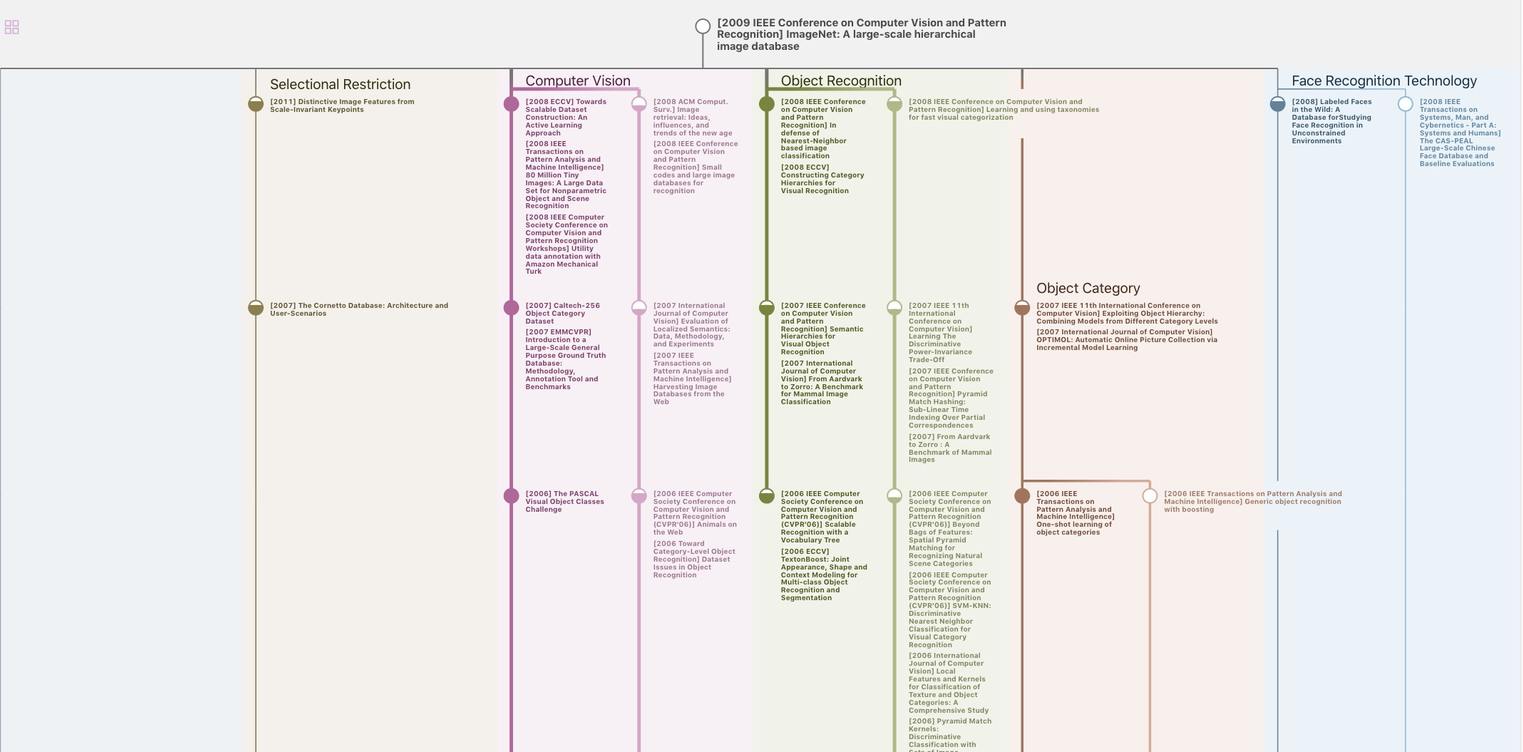
生成溯源树,研究论文发展脉络
Chat Paper
正在生成论文摘要