Incremental kNN Classifier Exploiting Correct-Error Teacher for Activity Recognition
Machine Learning and Applications(2010)
摘要
Non-stationary data distributions are a challenge in activity recognition from body worn motion sensors. Classifier models have to be adapted online to maintain a high recognition performance. Typical approaches for online learning are either unsupervised and potentially unstable, or require ground truth information which may be expensive to obtain. As an alternative we propose a teacher signal that can be provided by the user in a minimally obtrusive way. It indicates if the predicted activity for a feature vector is correct or wrong. To exploit this information we propose a novel incremental online learning strategy to adapt a k-nearest-neighbor classifier from instances that are indicated to be correctly or wrongly classified. We characterize our approach on an artificial dataset with abrupt distribution change that simulates a new user of an activity recognition system. The adapted classifier reaches the same accuracy as a classifier trained specifically for the new data distribution. The learning based on the provided correct - error signal also results in a faster learning speed compared to online learning from ground truth. We validate our approach on a real world gesture recognition dataset. The adapted classifiers achieve an accuracy of 78.6% compared to the subject independent baseline of 68.3%.
更多查看译文
关键词
classifier model,incremental knn,activity recognition system,k-nearest-neighbor classifier,classifier exploiting correct-error teacher,online learning,activity recognition,abrupt distribution change,high recognition performance,novel incremental online,non-stationary data distribution,real world gesture recognition,learning artificial intelligence,feature vector,artificial neural networks,upper bound,sensors,ground truth,accuracy,gesture recognition,stability analysis,k nearest neighbor,data models
AI 理解论文
溯源树
样例
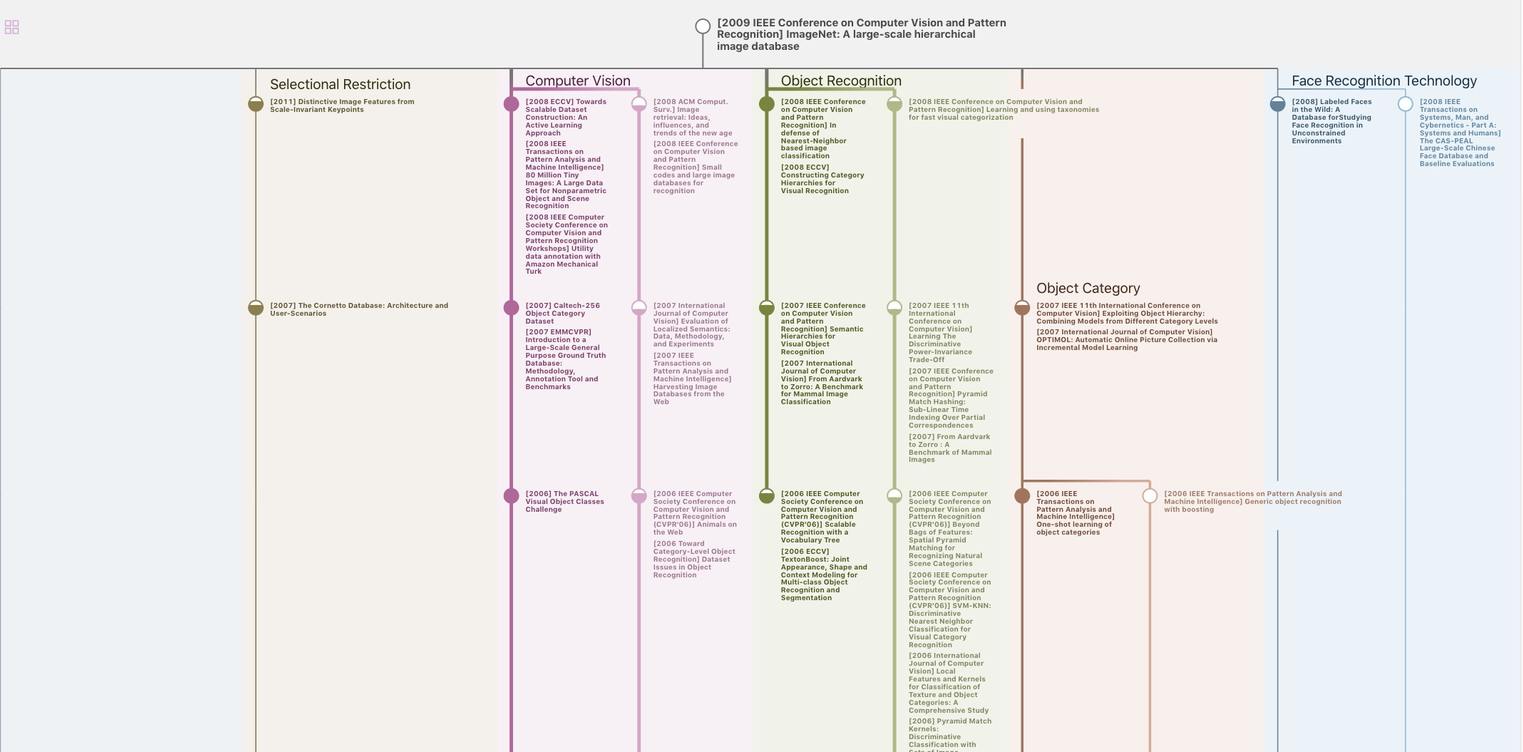
生成溯源树,研究论文发展脉络
Chat Paper
正在生成论文摘要