Inferring Parameters of Gene Regulatory Networks via Particle Filtering
EURASIP Journal on Advances in Signal Processing(2010)
摘要
Gene regulatory networks are highly complex dynamical systems comprising biomolecular components which interact with each other and through those interactions determine gene expression levels, that is, determine the rate of gene transcription. In this paper, a particle filter with Markov Chain Monte Carlo move step is employed for the estimation of reaction rate constants in gene regulatory networks modeled by chemical Langevin equations. Simulation studies demonstrate that the proposed technique outperforms previously considered methods while being computationally more efficient. Dynamic behavior of gene regulatory networks averaged over a large number of cells can be modeled by ordinary differential equations. For this scenario, we compute an approximation to the Cramer-Rao lower bound on the mean-square error of estimating reaction rates and demonstrate that, when the number of unknown parameters is small, the proposed particle filter can be nearly optimal.
更多查看译文
关键词
particle filter, gene regulatory network, parameter estimation
AI 理解论文
溯源树
样例
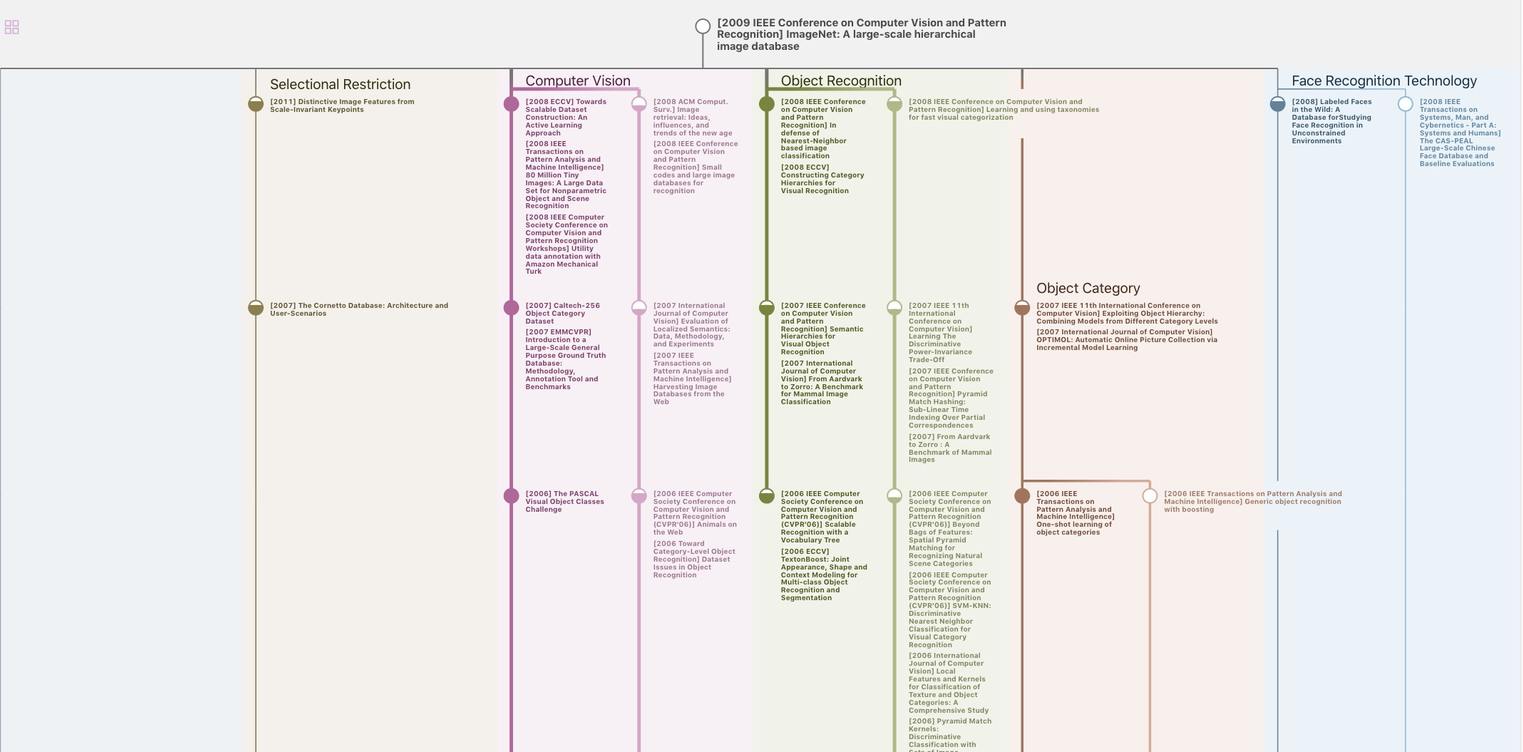
生成溯源树,研究论文发展脉络
Chat Paper
正在生成论文摘要