L0 regularized logistic regression for large-scale data
PATTERN RECOGNITION(2024)
摘要
In this paper, we investigate L-0-regularized logistic regression models, and design two fast and efficient algorithms for high-dimensional correlated data and massive data, respectively. Our first algorithm, the Variable Sorted Active Set (VSAS) algorithm, is based on the local quadratic approximation of the KKT conditions for L-0-penalized maximum log-likelihood function in high-dimensional correlated data. We establish an L-infinity error upper bound for the estimator obtained by the VSAS algorithm and prove its optimal convergence rate. Moreover, when the target signal exceeds the detectable level, the estimator obtained by the VSAS algorithm can achieve the oracle estimator with high probability. Our second algorithm, Communication Effective Variable Sorted Active Set (CEVSAS), aims to solve high-dimensional and large-sample L-0-regularized logistic regression models by reduce computational and communication costs, while maintaining estimation efficiency. Finally, simulations and real data demonstrate the effectiveness of our proposed VSAS and CEVSAS algorithms.
更多查看译文
关键词
Distributed learning,L-0 penalty,KKT conditions,Oracle property,Correlated effects
AI 理解论文
溯源树
样例
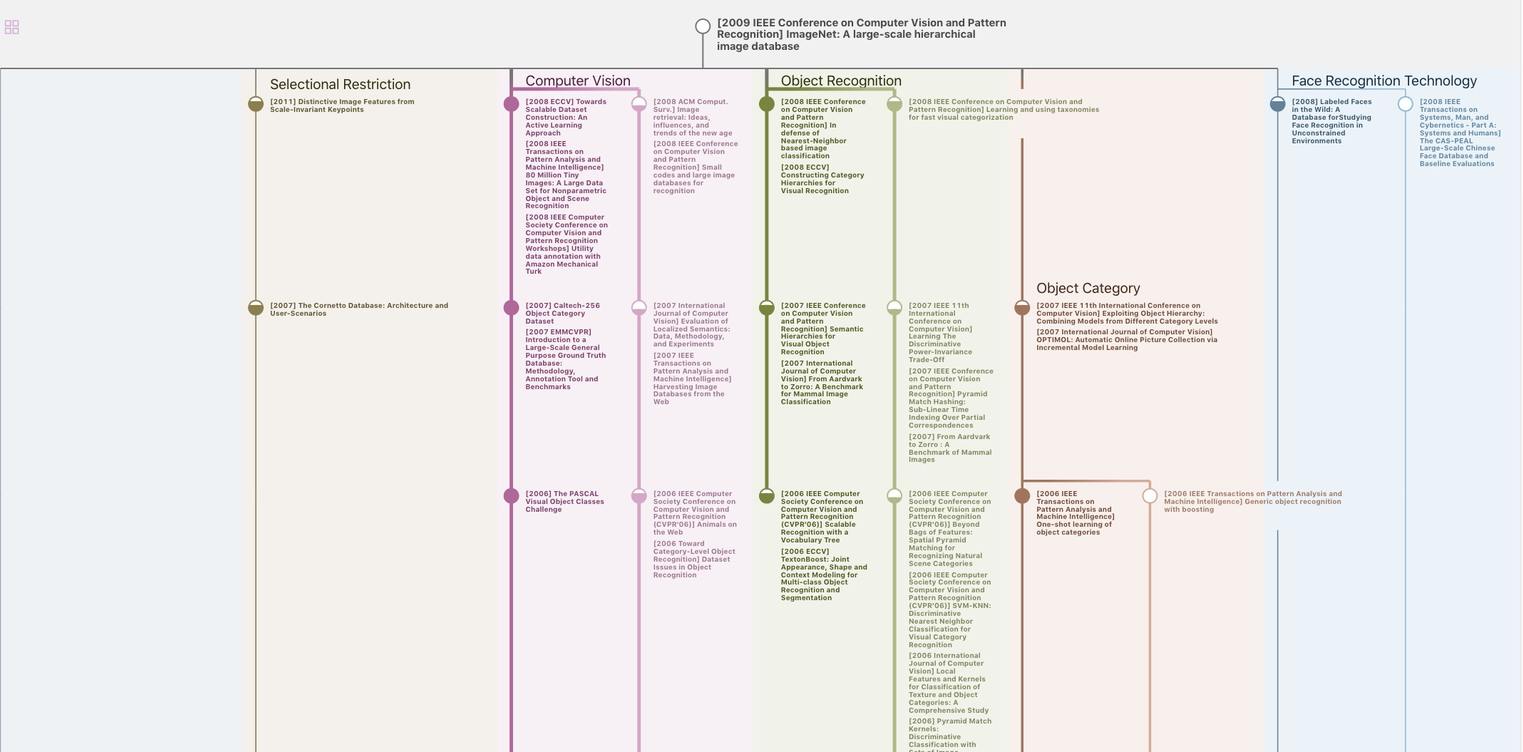
生成溯源树,研究论文发展脉络
Chat Paper
正在生成论文摘要