A dynamically adaptive wavelet approach to stochastic computations based on polynomial chaos - capturing all scales of random modes on independent grids
Journal of Computational Physics(2011)
摘要
In stochastic computations, or uncertainty quantification methods, the spectral approach based on the polynomial chaos expansion in random space leads to a coupled system of deterministic equations for the coefficients of the expansion. The size of this system increases drastically when the number of independent random variables and/or order of polynomial chaos expansions increases. This is invariably the case for large scale simulations and/or problems involving steep gradients and other multiscale features; such features are variously reflected on each solution component or random/uncertainty mode requiring the development of adaptive methods for their accurate resolution. In this paper we propose a new approach for treating such problems based on a dynamically adaptive wavelet methodology involving space-refinement on physical space that allows all scales of each solution component to be refined independently of the rest. We exemplify this using the convection-diffusion model with random input data and present three numerical examples demonstrating the salient features of the proposed method. Thus we establish a new, elegant and flexible approach for stochastic problems with steep gradients and multiscale features based on polynomial chaos expansions.
更多查看译文
关键词
uncertainty quantification,stochastic convection–diffusion,random mode,dynamically adaptive wavelet approach,solution component,polynomial chaos expansions increase,new approach,polynomial chaos expansion,independent random variable,stochastic space-refinement,independent grid,stochastic wavelet multiscale solver,random space,flexible approach,steep gradients in stochastic problems,spectral approach,steep gradient,spectral methods,random input data,spectral method,polynomial chaos,diffusion model
AI 理解论文
溯源树
样例
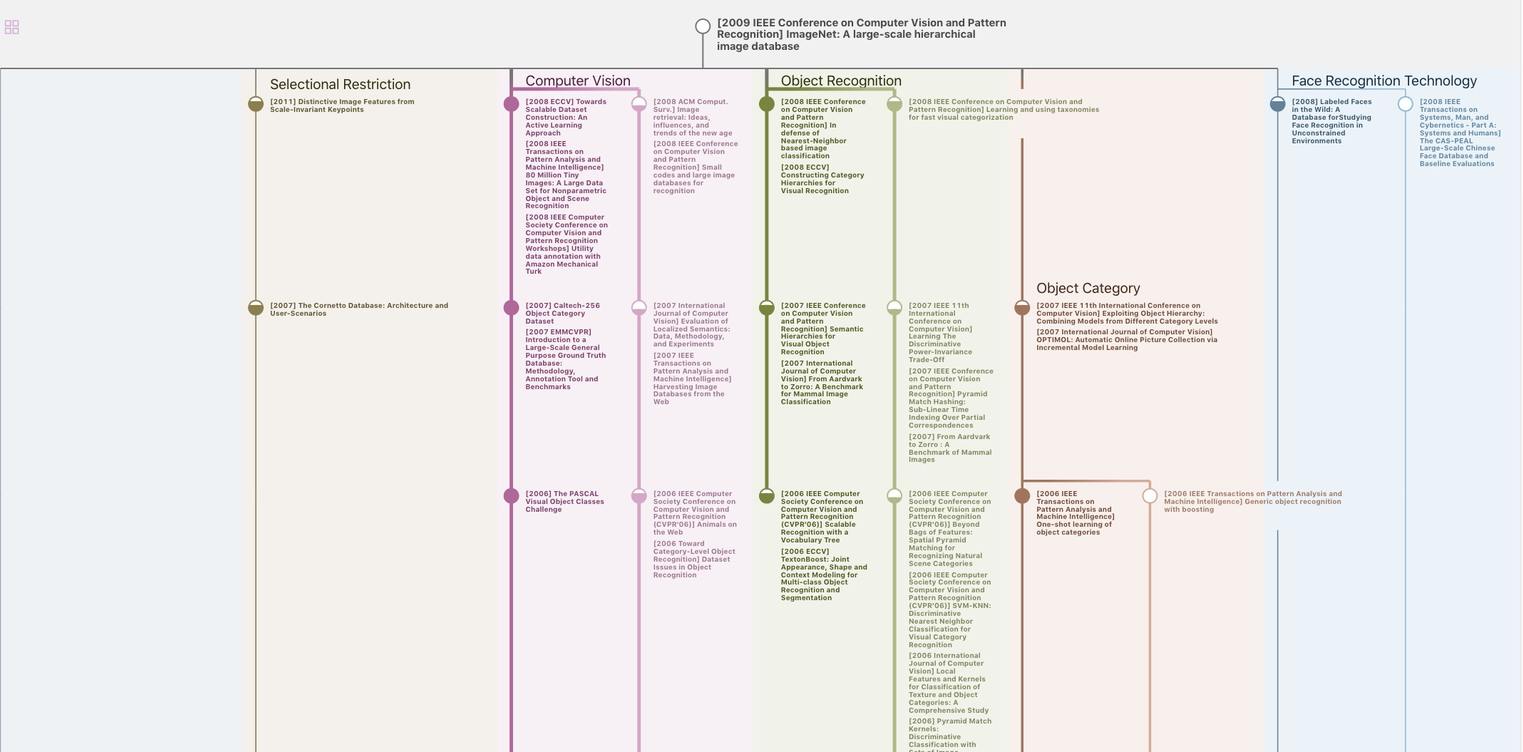
生成溯源树,研究论文发展脉络
Chat Paper
正在生成论文摘要